Retrieval-Based Gradient Boosting Decision Trees for Disease Risk Assessment
KDD '22: Proceedings of the 28th ACM SIGKDD Conference on Knowledge Discovery and Data Mining(2022)
Abstract
In recent years, machine learning methods have been widely used in modern electronic health record (EHR) systems, and have shown more accurate prediction performance on disease risk assessment tasks than traditional methods. However, most of the existing machine learning methods make the assessment solely based on features of the target case but ignore the cross-sample feature interactions between the target case and other similar cases, which is inconsistent with the general practice of evidence-based medicine of making diagnoses based on existing clinical experience. Moreover, current methods that focus on mining cross-sample information rely on deep neural networks to extract cross-sample feature interactions, which would suffer from the problems of data insufficiency, data heterogeneity and lack of interpretability in disease risk assessment tasks. In this work, we propose a novel retrieval-based gradient boosting decision trees (RB-GBDT) model with a cross-sample extractor to mine cross-sample information while exploiting the superiority of GBDT of robustness, generalization and interpretability. Experiments on real-world clinical datasets show the superiority and efficacy of RB-GBDT on disease risk assessment tasks. The developed software has been deployed in hospital as an auxiliary diagnosis tool for risk assessment of venous thromboembolism.
MoreTranslated text
Key words
gradient boosting decision trees,risk assessment,retrieval-based
AI Read Science
Must-Reading Tree
Example
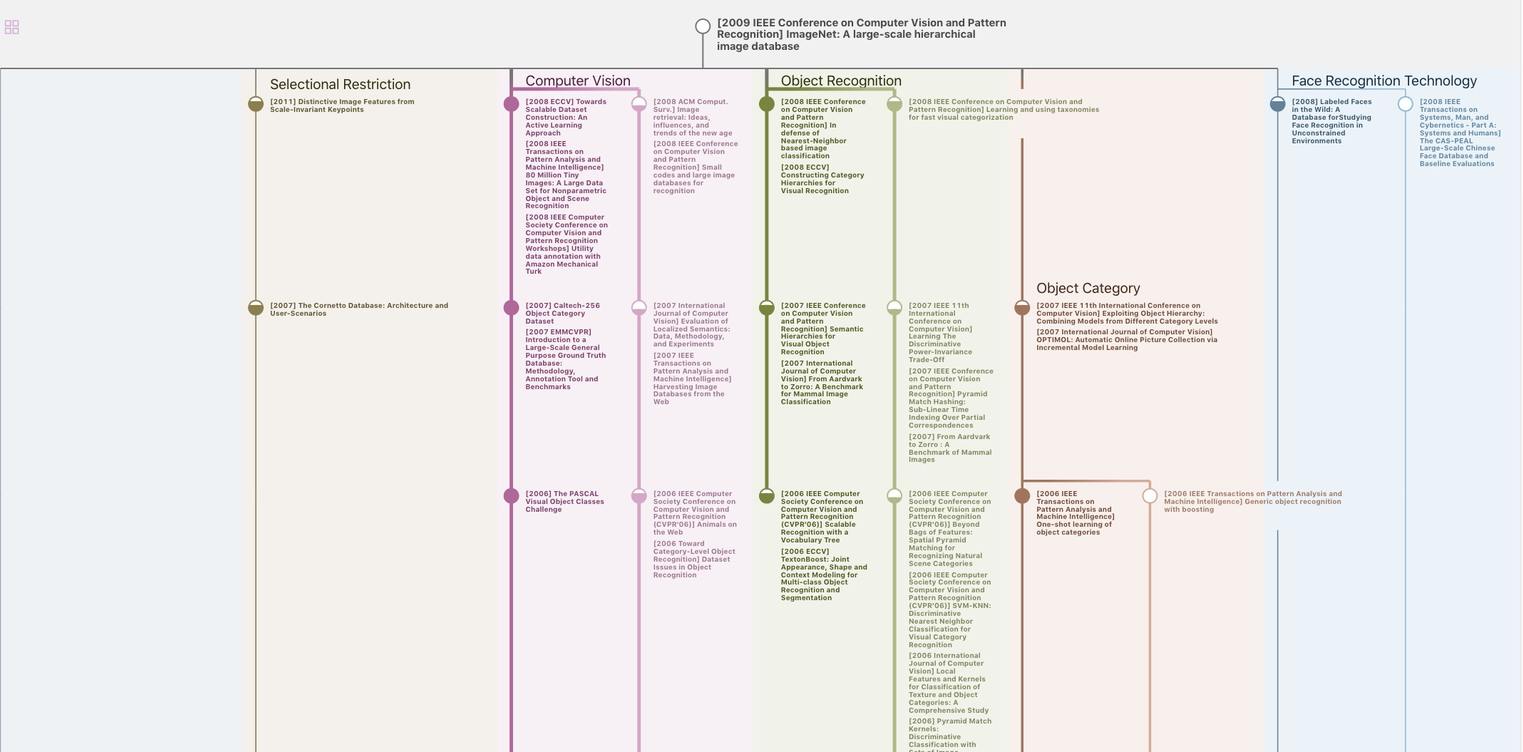
Generate MRT to find the research sequence of this paper
Chat Paper
Summary is being generated by the instructions you defined