Three-Stage Root Cause Analysis for Logistics Time Efficiency via Explainable Machine Learning
KDD '22: Proceedings of the 28th ACM SIGKDD Conference on Knowledge Discovery and Data Mining(2022)
摘要
The performance of logistics highly depends on the time efficiency, and hence, plenty of efforts have been devoted to ensuring the on-time delivery in modern logistics industry. However, the delay in logistics transportation and delivery can still happen due to various practical issues, which significantly impact the quality of logistics service. In order to address this issue, this work investigates the root causes impacting the time efficiency, thereby facilitating the operation of logistics systems such that resources can be appropriately allocated to improve the performance. The proposed solution comprises three stages, where statistical methods are employed in the first stage to analyze the pattern of on-time delivery rate and detect the abnormalities induced by non-ideality of operations. Subsequently, a machine learning model is trained to capture the underlying correlations between time efficiency and potential impacting factors. Finally, explainable machine learning techniques are utilized to quantify the contributions of the impacting factors to the time efficiency, thereby recognizing the root causes. The proposed method is comprehensively studied on the real JD Logistics data through experiments, where it can identify the root causes that impact the time efficiency of logistics delivery with high accuracy. Furthermore, it is also demonstrated to outperform the baselines including a recent state-of-the-art method.
更多查看译文
关键词
logistics time efficiency,explainable machine learning,machine learning,three-stage
AI 理解论文
溯源树
样例
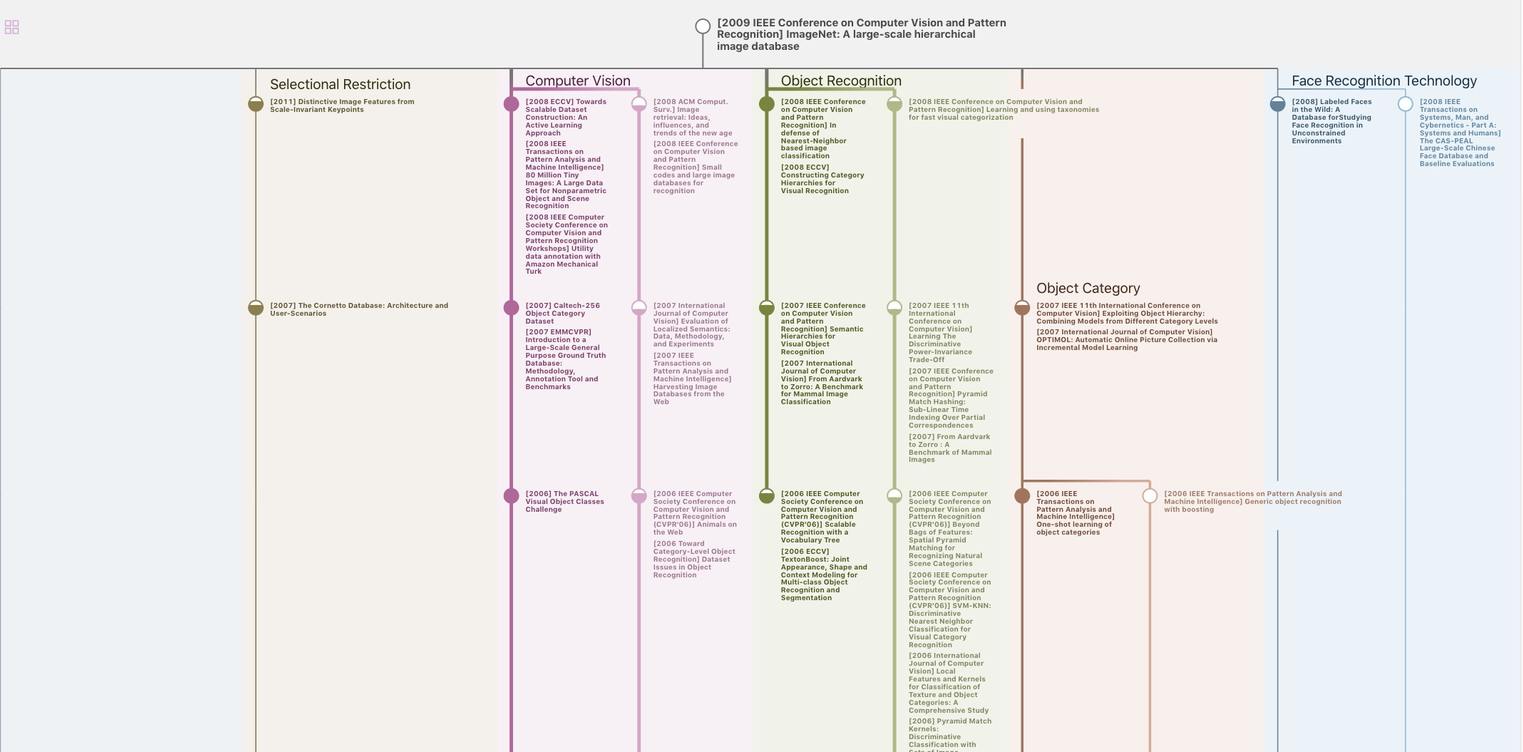
生成溯源树,研究论文发展脉络
Chat Paper
正在生成论文摘要