Talent Demand-Supply Joint Prediction with Dynamic Heterogeneous Graph Enhanced Meta-Learning
KDD '22: Proceedings of the 28th ACM SIGKDD Conference on Knowledge Discovery and Data Mining(2022)
摘要
Talent demand and supply forecasting aims to model the variation of the labor market, which is crucial to companies for recruitment strategy adjustment and to job seekers for proactive career path planning. However, existing approaches either focus on talent demand or supply forecasting, but overlook the interconnection between demand-supply sequences among different companies and positions. To this end, in this paper, we propose a Dynamic Heterogeneous Graph Enhanced Meta-learning (DH-GEM) framework for fine-grained talent demand-supply joint prediction. Specifically, we first propose a Demand-Supply Joint Encoder-Decoder (DSJED) and a Dynamic Company-Position Heterogeneous Graph Convolutional Network (DyCP-HGCN) to respectively capture the intrinsic correlation between demand and supply sequences and company-position pairs. Moreover, a Loss-Driven Sampling based Meta-learner (LDSM) is proposed to optimize long-tail forecasting tasks with a few training data. Extensive experiments have been conducted on three real-world datasets to demonstrate the effectiveness of our approach compared with five baselines. DH-GEM has been deployed as a core component of the intelligent human resource system of a cooperative partner.
更多查看译文
关键词
prediction,demand-supply,meta-learning
AI 理解论文
溯源树
样例
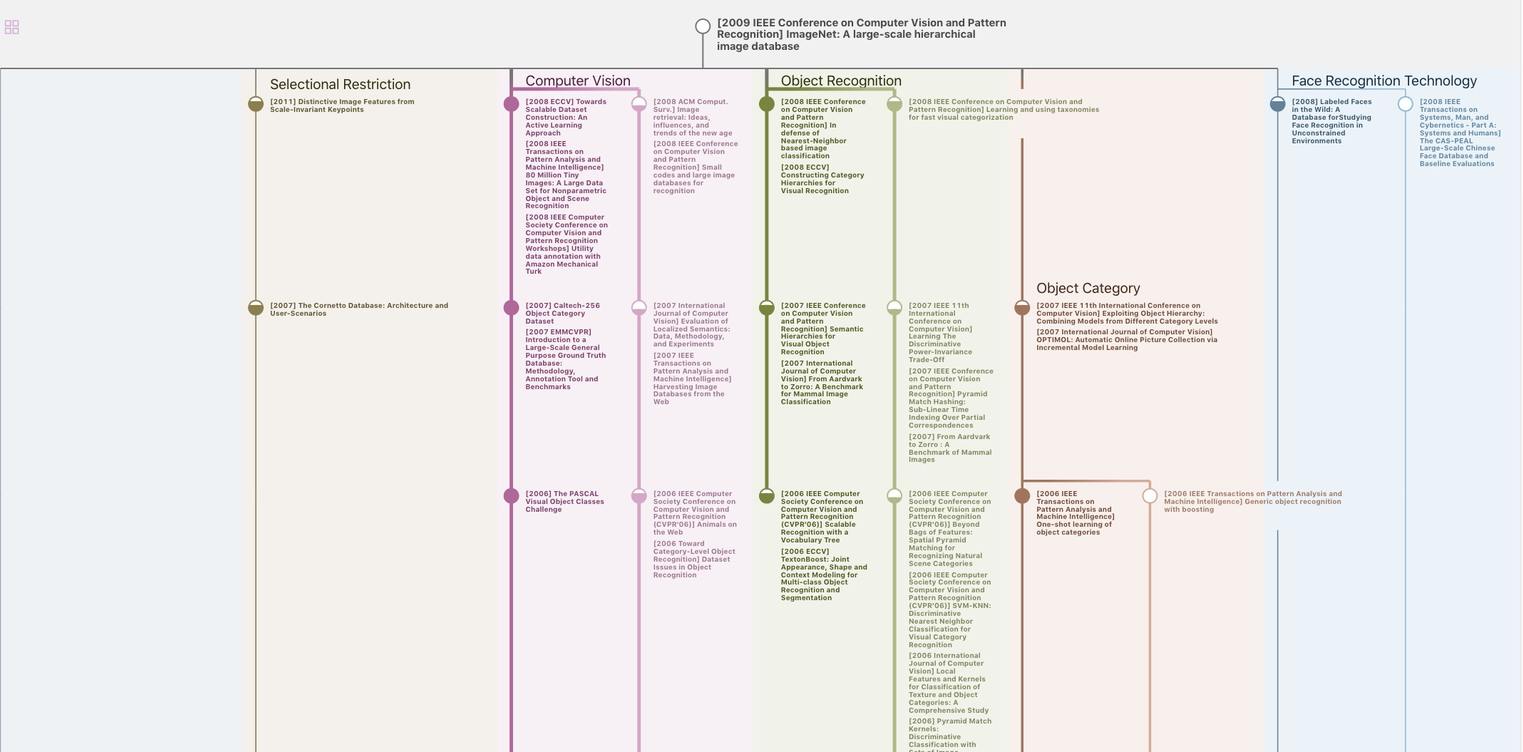
生成溯源树,研究论文发展脉络
Chat Paper
正在生成论文摘要