Dual Bidirectional Graph Convolutional Networks for Zero-shot Node Classification
PROCEEDINGS OF THE 28TH ACM SIGKDD CONFERENCE ON KNOWLEDGE DISCOVERY AND DATA MINING, KDD 2022(2022)
摘要
Zero-shot node classification is a very important challenge for classical semi-supervised node classification algorithms, such as Graph Convolutional Network (GCN) which has been widely applied to node classification. In order to predict the unlabeled nodes from unseen classes, zero-shot node classification needs to transfer knowledge from seen classes to unseen classes. It is crucial to consider the relations between the classes in zero-shot node classification. However, the GCN only considers the relations between the nodes, not the relations between the classes. Therefore, the GCN can not handle the zero-shot node classification effectively. This paper proposes a Dual Bidirectional Graph Convolutional Networks (DBiGCN) that consists of dual BiGCNs from the perspective of the nodes and the classes, respectively. The BiGCN can integrate the relations between the nodes and between the classes simultaneously in an united network. In addition, to make the dual BiGCNs work collaboratively, a label consistency loss is introduced, which can achieve mutual guidance and mutual improvement between the dual BiGCNs. Finally, the experimental results on real-world graph data sets verify the effectiveness of the proposed method.
更多查看译文
关键词
Dual Bidirectional GCNs,Zero-shot Node Classification,Graph Data Analysis
AI 理解论文
溯源树
样例
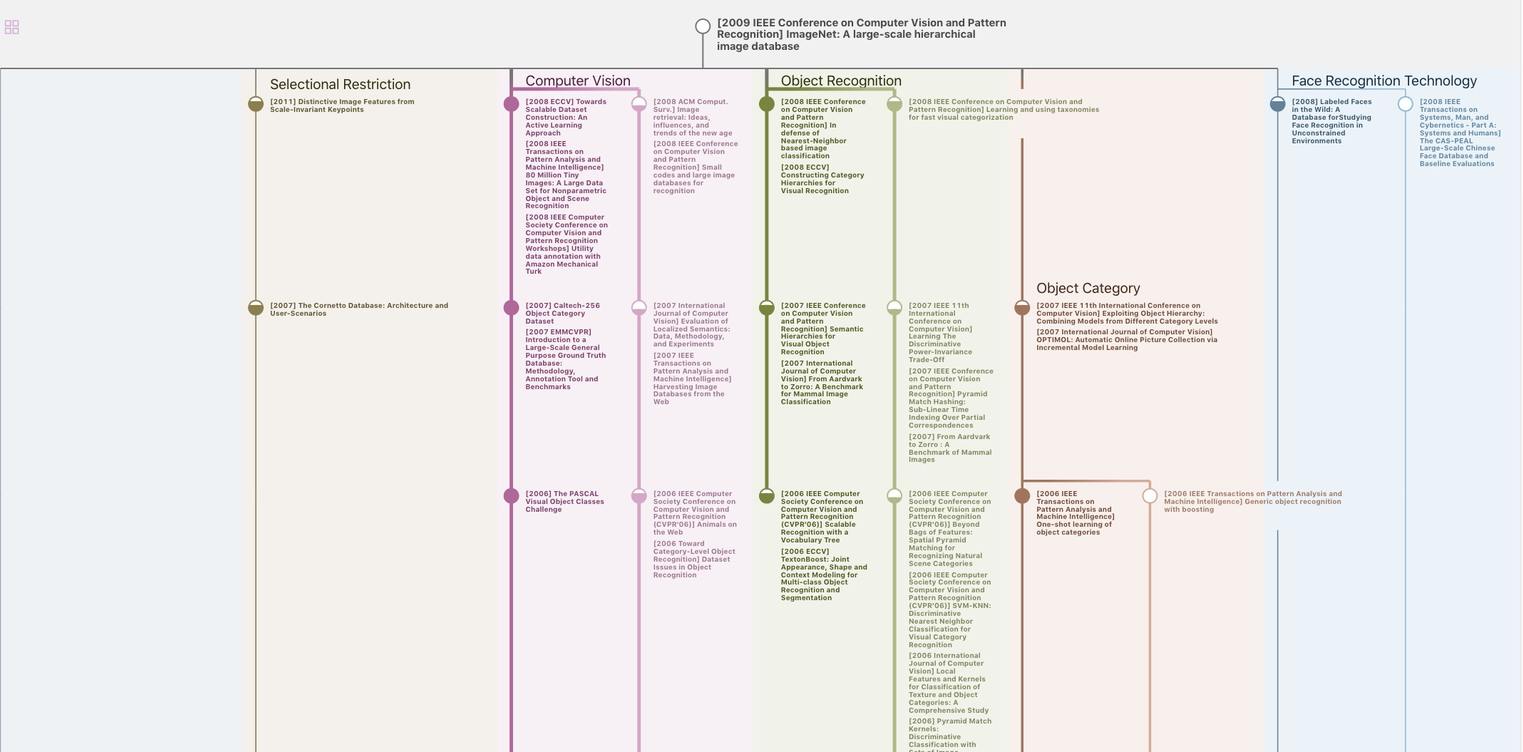
生成溯源树,研究论文发展脉络
Chat Paper
正在生成论文摘要