MonoSIM: Simulating Learning Behaviors of Heterogeneous Point Cloud Object Detectors for Monocular 3D Object Detection
arxiv(2022)
摘要
Monocular 3D object detection is a fundamental but very important task to many applications including autonomous driving, robotic grasping and augmented reality. Existing leading methods tend to estimate the depth of the input image first, and detect the 3D object based on point cloud. This routine suffers from the inherent gap between depth estimation and object detection. Besides, the prediction error accumulation would also affect the performance. In this paper, a novel method named MonoSIM is proposed. The insight behind introducing MonoSIM is that we propose to simulate the feature learning behaviors of a point cloud based detector for monocular detector during the training period. Hence, during inference period, the learned features and prediction would be similar to the point cloud based detector as possible. To achieve it, we propose one scene-level simulation module, one RoI-level simulation module and one response-level simulation module, which are progressively used for the detector's full feature learning and prediction pipeline. We apply our method to the famous M3D-RPN detector and CaDDN detector, conducting extensive experiments on KITTI and Waymo Open datasets. Results show that our method consistently improves the performance of different monocular detectors for a large margin without changing their network architectures. Our codes will be publicly available at https://github.com/sunh18/MonoSIM}{https://github.com/sunh18/MonoSIM.
更多查看译文
关键词
object detectors,heterogeneous point cloud
AI 理解论文
溯源树
样例
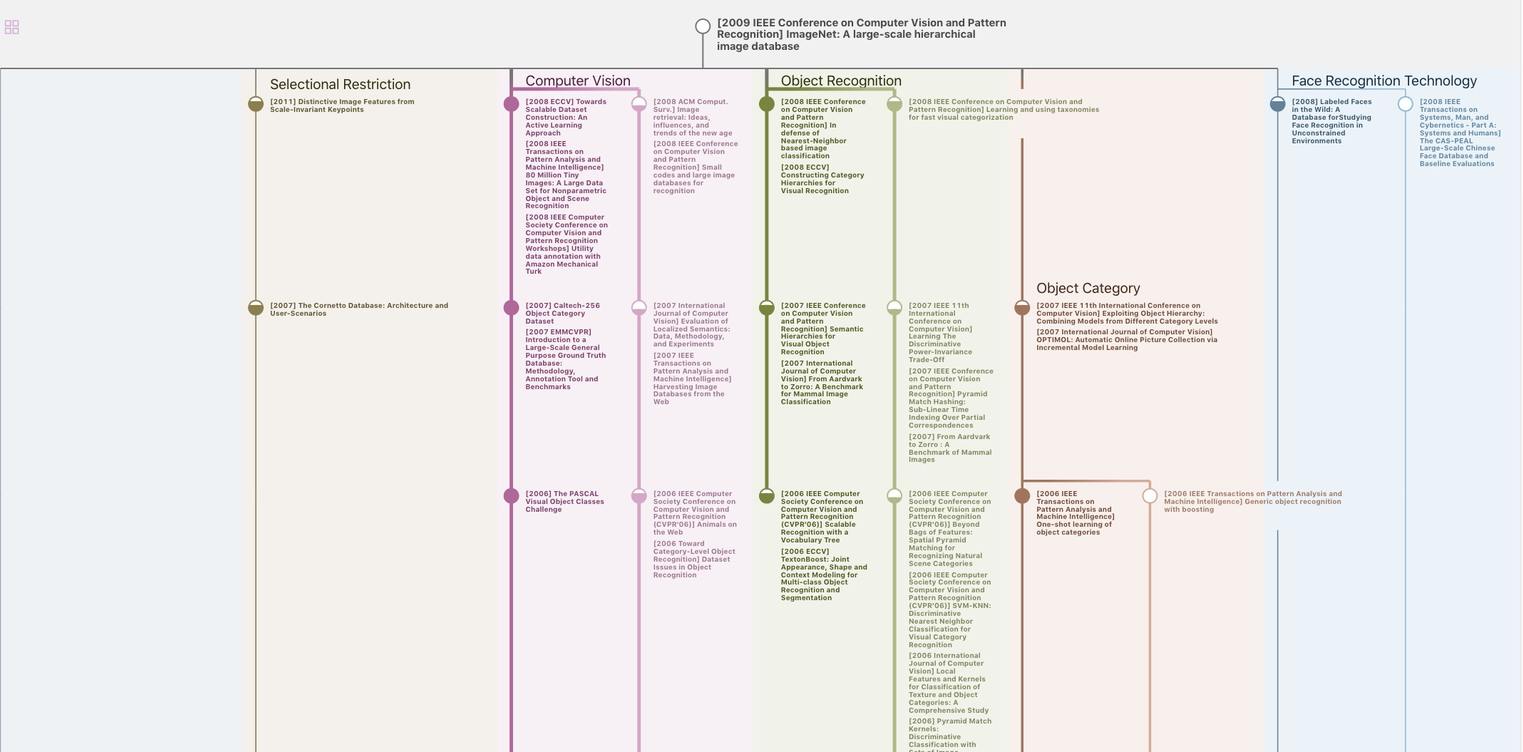
生成溯源树,研究论文发展脉络
Chat Paper
正在生成论文摘要