Disentangled Representation with Causal Constraints for Counterfactual Fairness
Advances in Knowledge Discovery and Data Mining(2022)
摘要
Much research has been devoted to the problem of learning fair representations; however, they do not explicitly the relationship between latent representations. In many real-world applications, there may be causal relationships between latent representations. Furthermore, most fair representation learning methods focus on group-level fairness and are based on correlations, ignoring the causal relationships underlying the data. In this work, we theoretically demonstrate that using the structured representations enable downstream predictive models to achieve counterfactual fairness, and then we propose the Counterfactual Fairness Variational AutoEncoder (CF-VAE) to obtain structured representations with respect to domain knowledge. The experimental results show that the proposed method achieves better fairness and accuracy performance than the benchmark fairness methods.
更多查看译文
关键词
Counterfactual Fairness, Representation Learning, Variational AutoEncoder
AI 理解论文
溯源树
样例
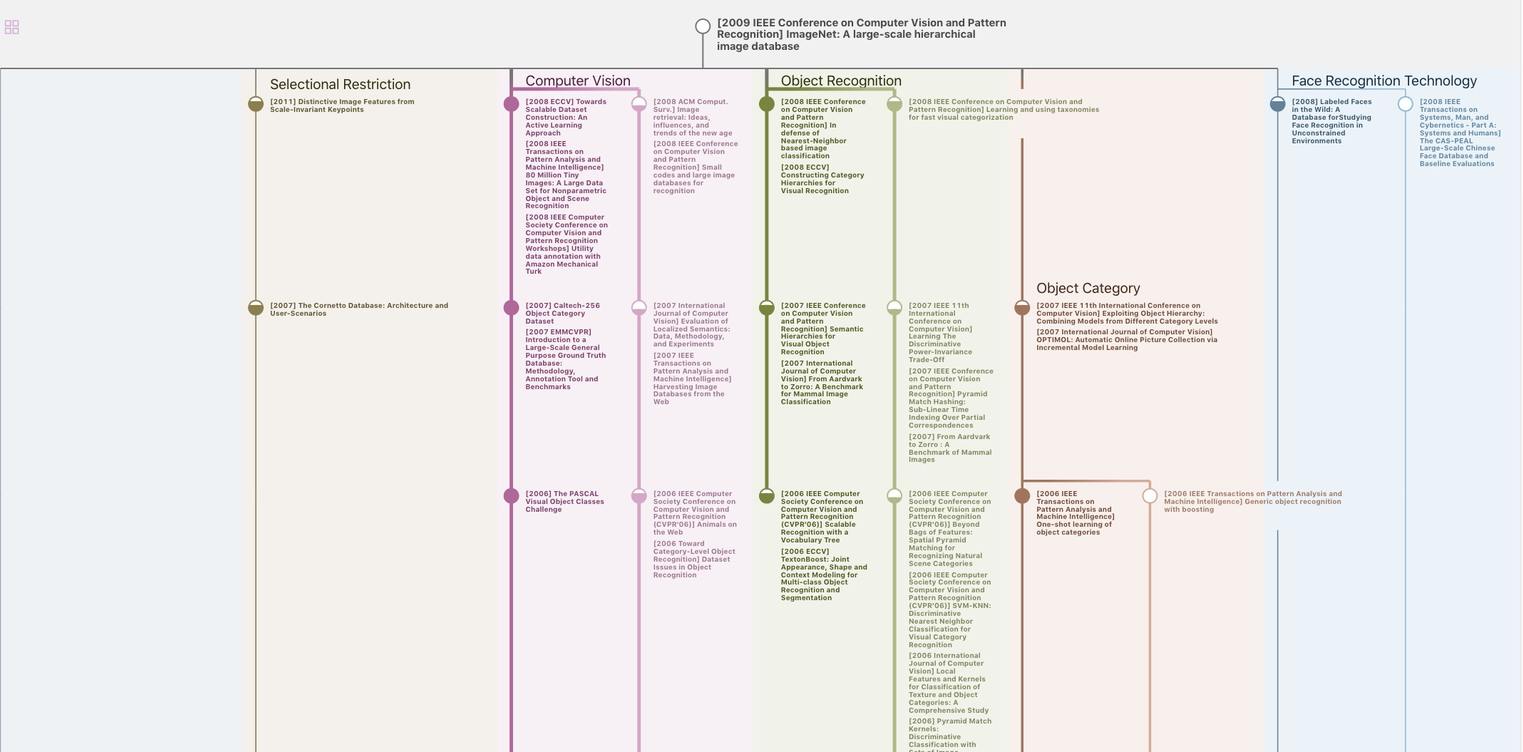
生成溯源树,研究论文发展脉络
Chat Paper
正在生成论文摘要