3M: An Effective Multi-view, Multi-granularity, and Multi-aspect Modeling Approach to English Pronunciation Assessment
arxiv(2022)
摘要
As an indispensable ingredient of computer-assisted pronunciation training (CAPT), automatic pronunciation assessment (APA) plays a pivotal role in aiding self-directed language learners by providing multi-aspect and timely feedback. However, there are at least two potential obstacles that might hinder its performance for practical use. On one hand, most of the studies focus exclusively on leveraging segmental (phonetic)-level features such as goodness of pronunciation (GOP); this, however, may cause a discrepancy of feature granularity when performing suprasegmental (prosodic)-level pronunciation assessment. On the other hand, automatic pronunciation assessments still suffer from the lack of large-scale labeled speech data of non-native speakers, which inevitably limits the performance of pronunciation assessment. In this paper, we tackle these problems by integrating multiple prosodic and phonological features to provide a multi-view, multi-granularity, and multi-aspect (3M) pronunciation modeling. Specifically, we augment GOP with prosodic and self-supervised learning (SSL) features, and meanwhile develop a vowel/consonant positional embedding for a more phonology-aware automatic pronunciation assessment. A series of experiments conducted on the publicly-available speechocean762 dataset show that our approach can obtain significant improvements on several assessment granularities in comparison with previous work, especially on the assessment of speaking fluency and speech prosody.
更多查看译文
关键词
computer-assisted pronunciation training,pronunciation assessment,goodness of pronunciation,segmental and suprasegmental features,self-supervised learning
AI 理解论文
溯源树
样例
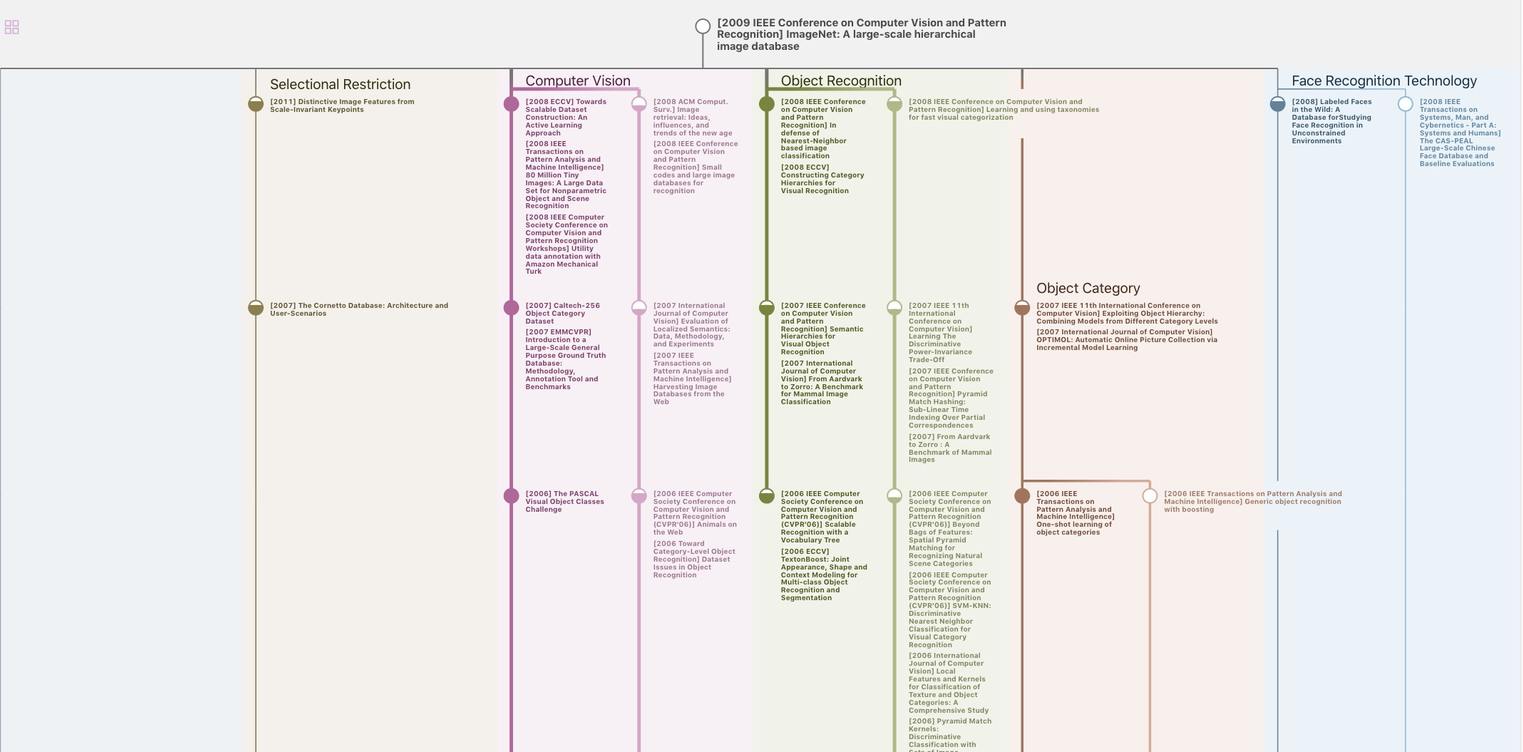
生成溯源树,研究论文发展脉络
Chat Paper
正在生成论文摘要