Construction of a HPLC-SERS hyphenated system for continuous separation and detection based on paper substrates
ANALYST(2022)
摘要
The achievement of high-throughput separation and high-sensitivity detection of complex samples has been one of the most challenging issues in the field of analytical science. The application of a single technology alone could not satisfy the above requirements. The combination of technologies with the capability of high-efficiency separation and high-sensitivity structural-recognition is highly desired to meet the technical requirements. Herein, an automatic high-performance liquid chromatography (HPLC)-surface enhanced Raman spectroscopy (SERS) hyphenated system using paper substrates as the "interface" was constructed to achieve efficient separation and real-time detection. A homogeneous Au nanoparticle was printed on the hydrophobic filter paper with the inkjet technology. The prepared substrates served as a linkage for the continuous realization of HPLC and SERS functions. The complex system was separated by HPLC, and the effluents were loaded onto automatically and continuously replaceable paper substrates for real time SERS measurements. The continuous rapid separation and real-time detection of various two-component mixtures were achieved with the separation efficiency and detection sensitivity of each technology. The results demonstrated that the HPLC-SERS hyphenated system exhibited the complementary capability of the on-line separation and continuous structural identification of illegal additives in real samples. The detection sensitivity was increased by an order of magnitude to reach 10(-5) mol dm(-3), and the efficiency and accuracy for the separation and identification on the multi-components samples were higher than those of the individual HPLC or SERS technology. It is believed that the continuous paper substrate-based HPLC-SERS hyphenated system would be developed as a promising technique for the separation and identification of multi-components mixtures with high throughput.
更多查看译文
AI 理解论文
溯源树
样例
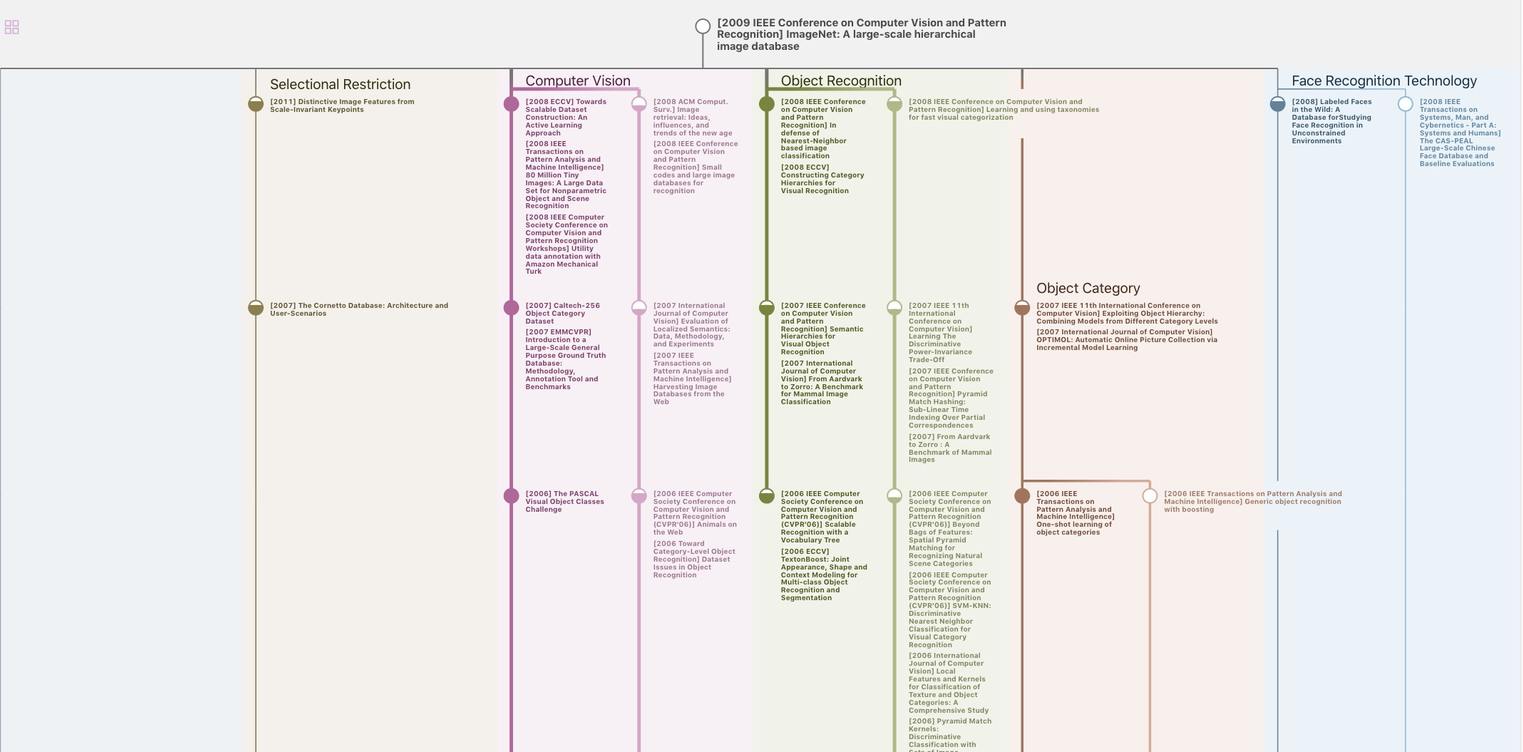
生成溯源树,研究论文发展脉络
Chat Paper
正在生成论文摘要