COPE: End-to-end trainable Constant Runtime Object Pose Estimation
WACV(2023)
摘要
State-of-the-art object pose estimation handles multiple instances in a test image by using multi-model formulations: detection as a first stage and then separately trained networks per object for 2D-3D geometric correspondence prediction as a second stage. Poses are subsequently estimated using the Perspective-n-Points algorithm at runtime. Unfortunately, multi-model formulations are slow and do not scale well with the number of object instances involved. Recent approaches show that direct 6D object pose estimation is feasible when derived from the aforementioned geometric correspondences. We present an approach that learns an intermediate geometric representation of multiple objects to directly regress 6D poses of all instances in a test image. The inherent end-to-end trainability overcomes the requirement of separately processing individual object instances. By calculating the mutual Intersectionover-Unions, pose hypotheses are clustered into distinct instances, which achieves negligible runtime overhead with respect to the number of object instances. Results on multiple challenging standard datasets show that the pose estimation performance is superior to single-model stateof-the-art approaches despite being more than similar to 35 times faster. We additionally provide an analysis showing realtime applicability (> 24 fps) for images where more than 90 object instances are present. Further results show the advantage of supervising geometric correspondence-based object pose estimation with the 6D pose.
更多查看译文
关键词
object,estimation,end-to-end
AI 理解论文
溯源树
样例
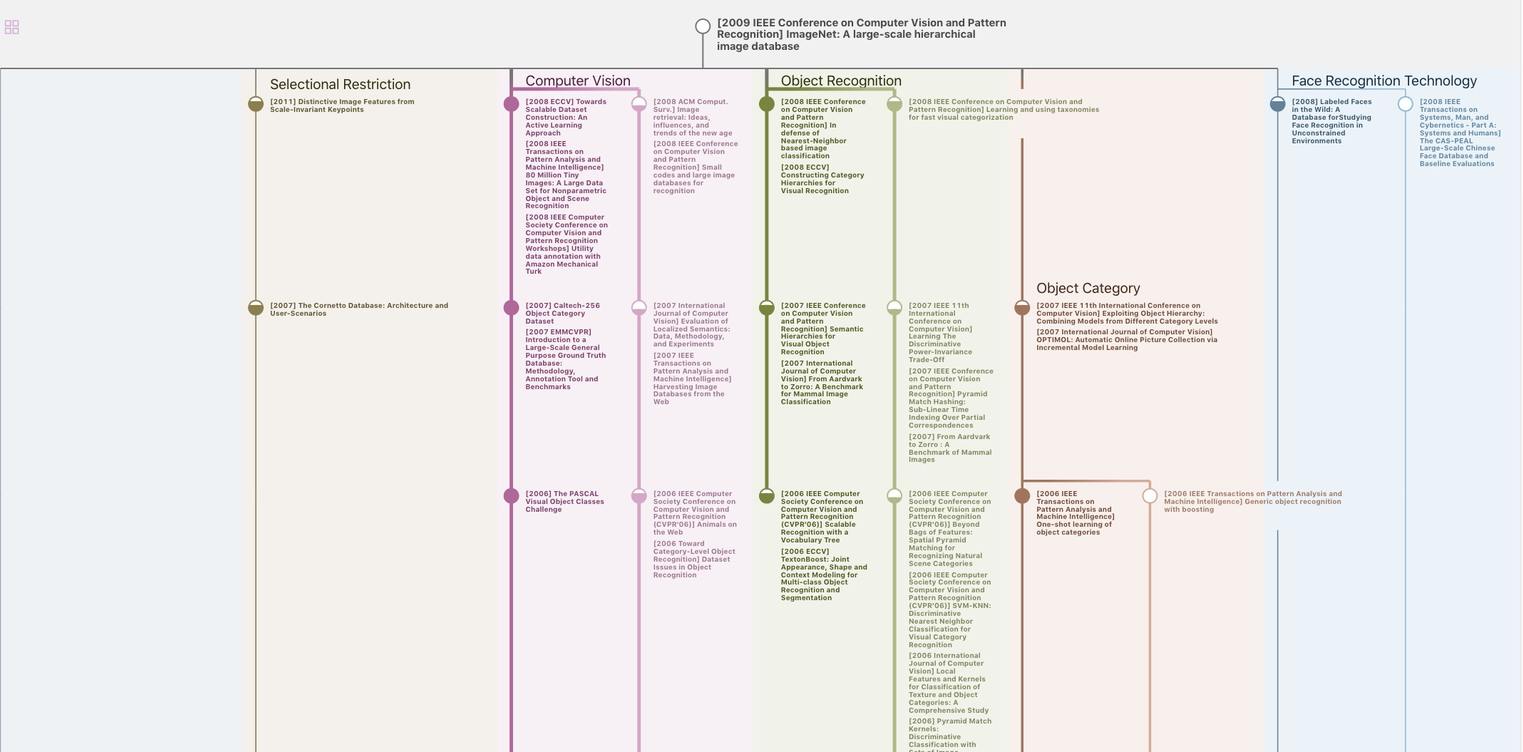
生成溯源树,研究论文发展脉络
Chat Paper
正在生成论文摘要