AoI-based Temporal Attention Graph Neural Network for Popularity Prediction and Content Caching
IEEE Transactions on Cognitive Communications and Networking(2022)
摘要
Along with the fast development of network technology and the rapid growth of network equipment, the data throughput is sharply increasing. To handle the problem of backhaul bottleneck in cellular network and satisfy people's requirements about latency, the network architecture like information-centric network (ICN) intends to proactively keep limited popular content at the edge of network based on predicted results. Meanwhile, the interactions between the content (e.g., deep neural network models, Wikipedia-alike knowledge base) and users could be regarded as a dynamic bipartite graph. In this paper, to maximize the cache hit rate, we leverage an effective dynamic graph neural network (DGNN) to jointly learn the structural and temporal patterns embedded in the bipartite graph. Furthermore, in order to have deeper insights into the dynamics within the evolving graph, we propose an age of information (AoI) based attention mechanism to extract valuable historical information while avoiding the problem of message staleness. Combining this aforementioned prediction model, we also develop a cache selection algorithm to make caching decisions in accordance with the prediction results. Extensive results demonstrate that our model can obtain a higher prediction accuracy than other state-of-the-art schemes in two real-world datasets. The results of hit rate further verify the superiority of the caching policy based on our proposed model over other traditional ways.
更多查看译文
关键词
Content caching,popularity prediction,dynamic graph neural network,age of information
AI 理解论文
溯源树
样例
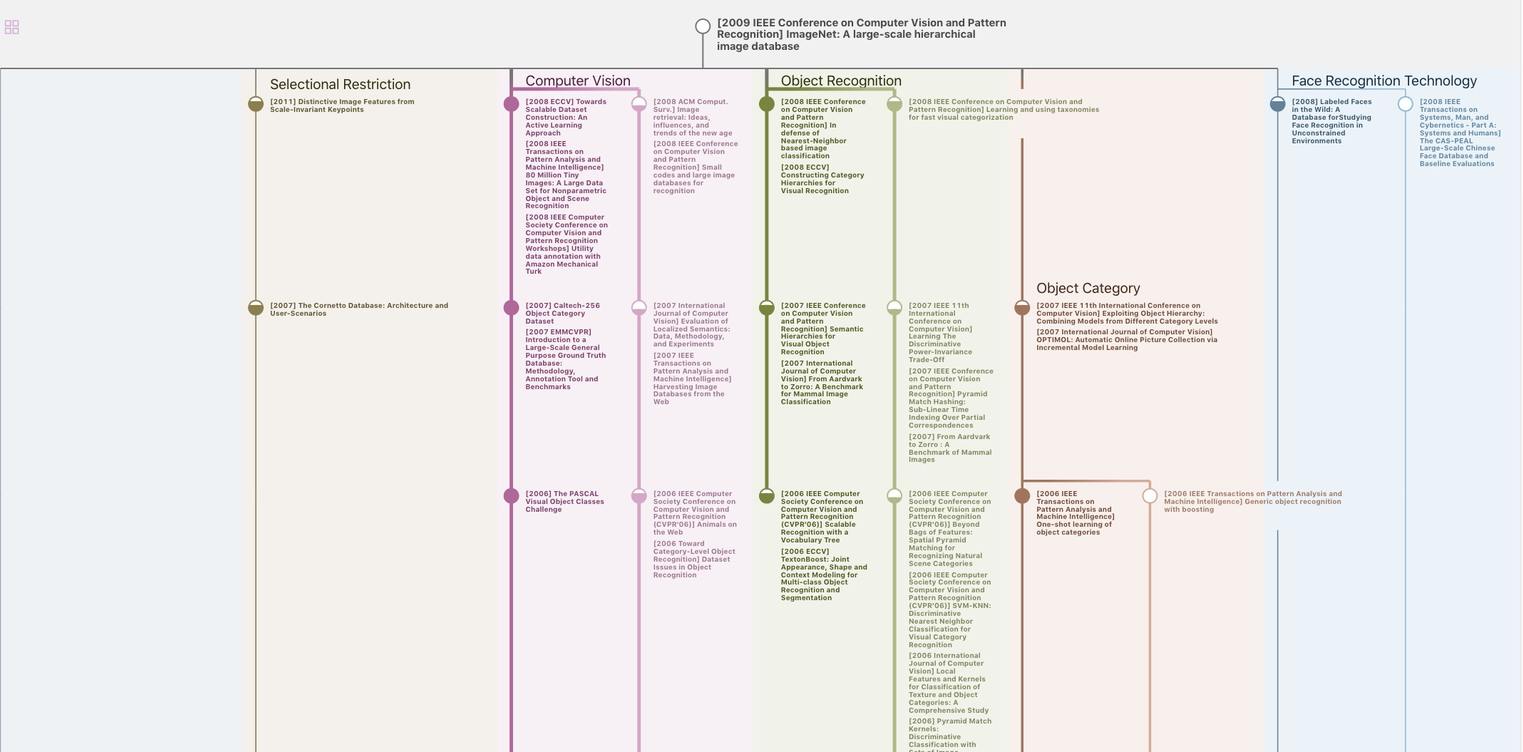
生成溯源树,研究论文发展脉络
Chat Paper
正在生成论文摘要