GRAPHIC: GatheR-And-Process in Highly parallel with In-SSD Compression Architecture in Very Large-Scale Graph
arXiv (Cornell University)(2022)
Abstract
Graph convolutional network (GCN), an emerging algorithm for graph computing, has achieved promising performance in graphstructure tasks. To achieve acceleration for data-intensive and sparse graph computing, ASICs such as GCNAX have been proposed for efficient execution of aggregation and combination in GCN. GCNAX reducing 8x DRAM accesses compared with previous efforts. However, as graphs have reached terabytes in size, off-chip data movement from SSD to DRAM becomes a serious latency bottleneck. This paper proposes Compressive Graph Transmission (CGTrans), which performs the aggregation in SSD to dramatically relieves the transfer latency bottleneck due to SSD loading compared to CMOS-based graph accelerator ASICs. InSSD computing technique is required for CGTrans. Recently, Insider was proposed as a near-SSD processing system computing by integrating FPGA in SSD. However, the Insider still suffers low area efficiency, which will limit the performance of CGTrans. The recently proposed Fully Concurrent Access Technique (FAST) is utilized. FAST-GAS, as an in-SSD graph computing accelerator, is proposed to provide high-concurrent gather-andscatter operations to overcome the area efficiency problem. We proposed the GRAPHIC system containing CGTrans dataflow deployed on FAST-GAS. Experiments show CGTrans reduces SSD loading by a factor of 50x, while GRAPHIC achieves 3.6x, and 2.4x speedup on average over GCNAX and CGTrans on Insider, respectively.
MoreTranslated text
Key words
compression
AI Read Science
Must-Reading Tree
Example
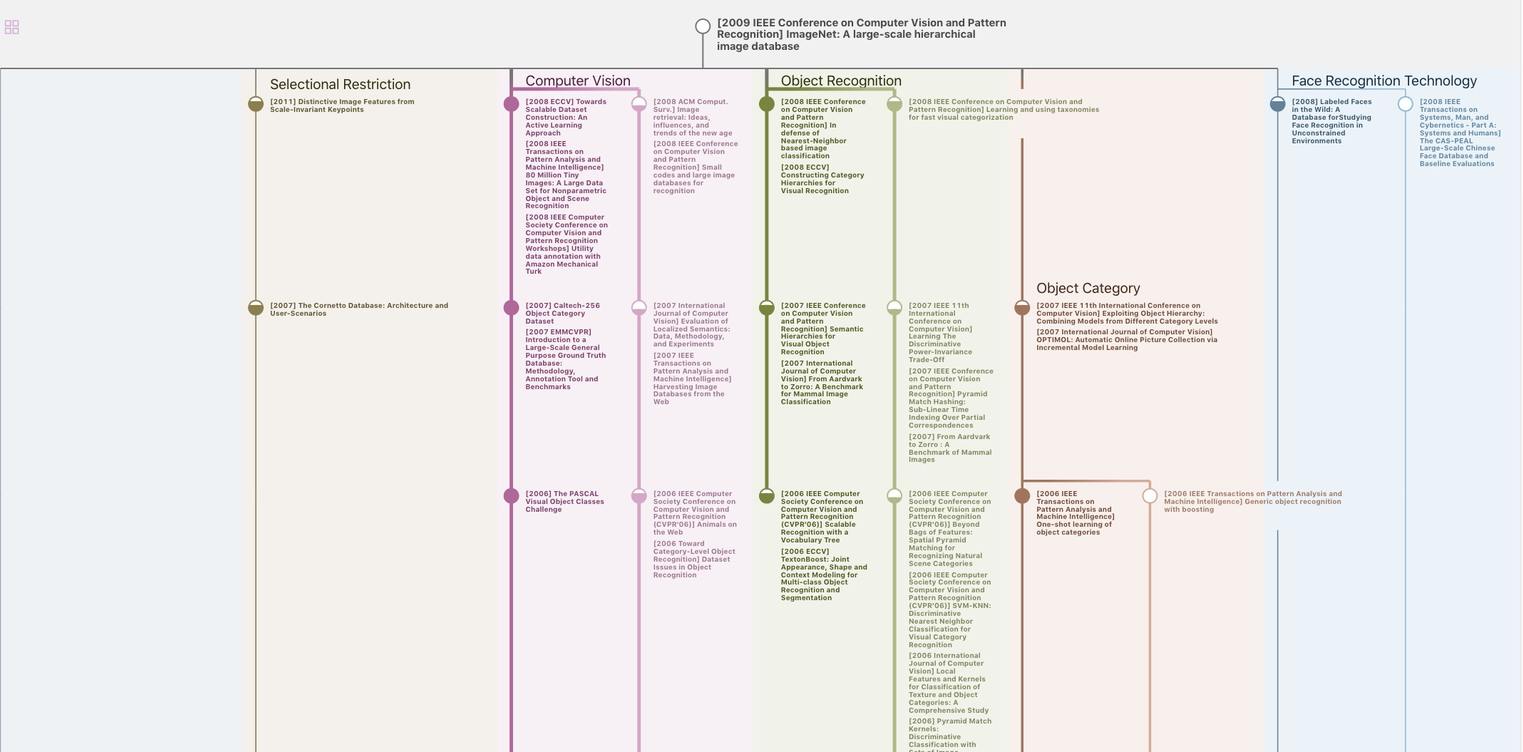
Generate MRT to find the research sequence of this paper
Chat Paper
Summary is being generated by the instructions you defined