Modeling urban expansion by integrating a convolutional neural network and a recurrent neural network
INTERNATIONAL JOURNAL OF APPLIED EARTH OBSERVATION AND GEOINFORMATION(2022)
摘要
Simulating urban expansion (UE) accurately is fundamental for projecting ecological and environmental impacts of future UE, for optimizing the urban landscape patterns, and for improving urban sustainability. We proposed a new UE model by integrating a convolutional neural network (i.e., U-Net) and a recurrent neural network (i.e., long short-term memory, LSTM), and applied it in the Beijing-Tianjin-Hebei urban agglomeration (BTHUA). The results yielded a high overall accuracy (99.18 %), a Kappa coefficient of 0.88 and a figure of merit of 0.13, which are greater than those of existing models. Such improvements are attributed to the multiscale neighborhood information powered by U-Net and the time series information of historical urban expansion uncovered by LSTM. The urban land in the BTHUA is projected to peak at 8736-9155 km(2) during the period 2039-2043, which is an increase in the range of 10.99-16.31 % compared with that in 2020. The results are useful for supporting urban planning in the BTHUA, while the proposed UE model has the potential to be employed worldwide.
更多查看译文
关键词
Urban expansion simulation,Cellular automata,Machine learning,Deep learning,Scenario analysis,Shared socioeconomic pathways
AI 理解论文
溯源树
样例
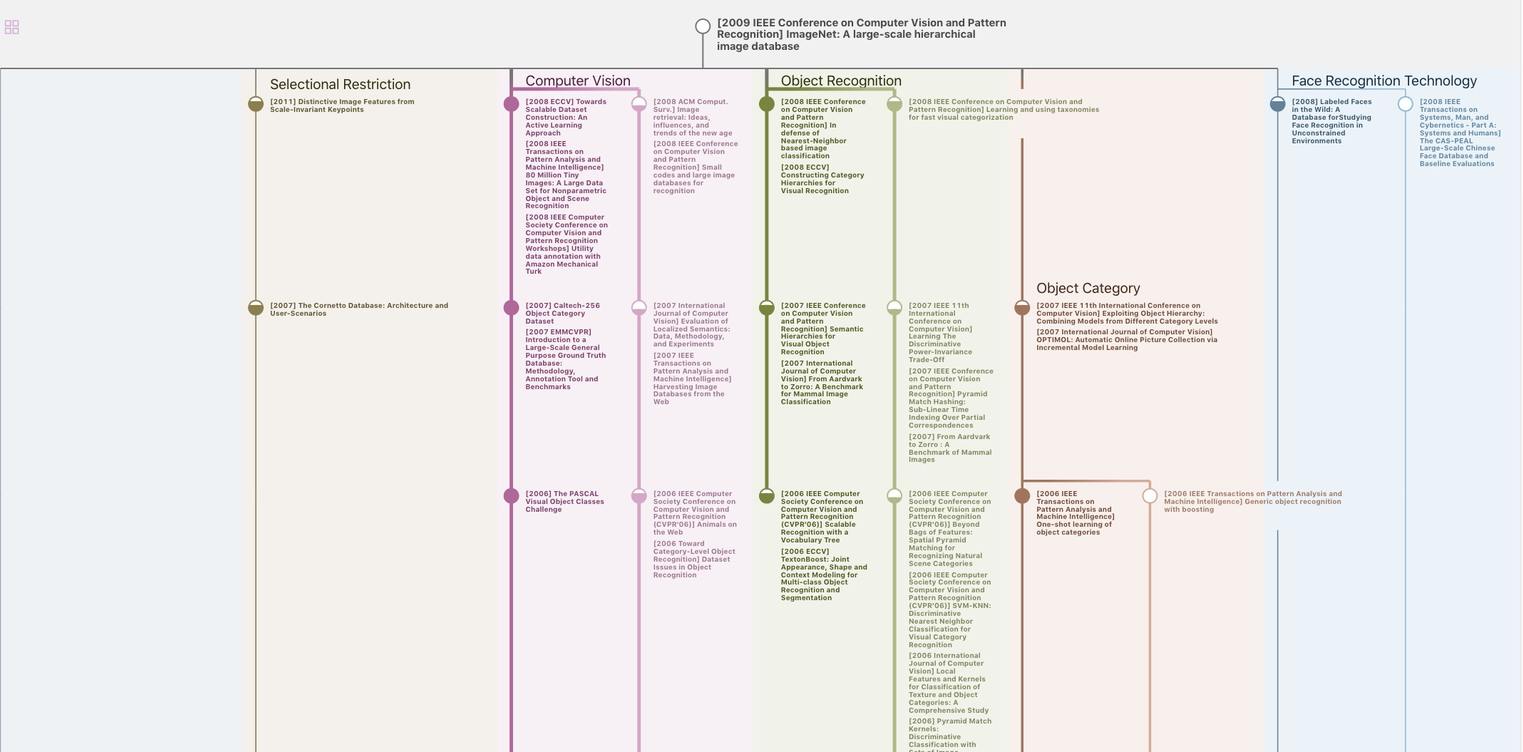
生成溯源树,研究论文发展脉络
Chat Paper
正在生成论文摘要