Synthesis of classification and predictive FFNN models on the basis of tenzoresistive transducer data
THE 9TH INTERNATIONAL CONFERENCE OF THE INDONESIAN CHEMICAL SOCIETY ICICS 2021: Toward a Meaningful Society AIP Conference Proceedings(2022)
Abstract
The paper presents quantitative and qualitative diagnosing approach for analysis of the sensory elements used to force measurements force at metal deformation and predicting of the degree of influence of applied force “F” using Feed- Forward Neural Networks (FFNN). This approach consists in the design and selection of neural models by backpropagation algorithms, respectively Scaled Conjugate Gradient (SCG) and Levenberg-Marquardt with functional procedures based on set of evaluation criteria, including Accuracy, Cross-Entropy (CE) and Mean Squared Error (MSE). The registered output electrical voltages from bridge circuits of switching on one and two strain gauge transducers from performed laboratory experiments were applied to investigate of FNN target models. According to SCG algorithm, neural architecture for quantitative sensory identification with 100 % achieved accuracy was synthesized. Using LM training rules, an architecture with a minimum MSE = 0.0029 for predictive analysis of the force effects on the test specimens was found.
MoreTranslated text
Key words
predictive ffnn models,classification
AI Read Science
Must-Reading Tree
Example
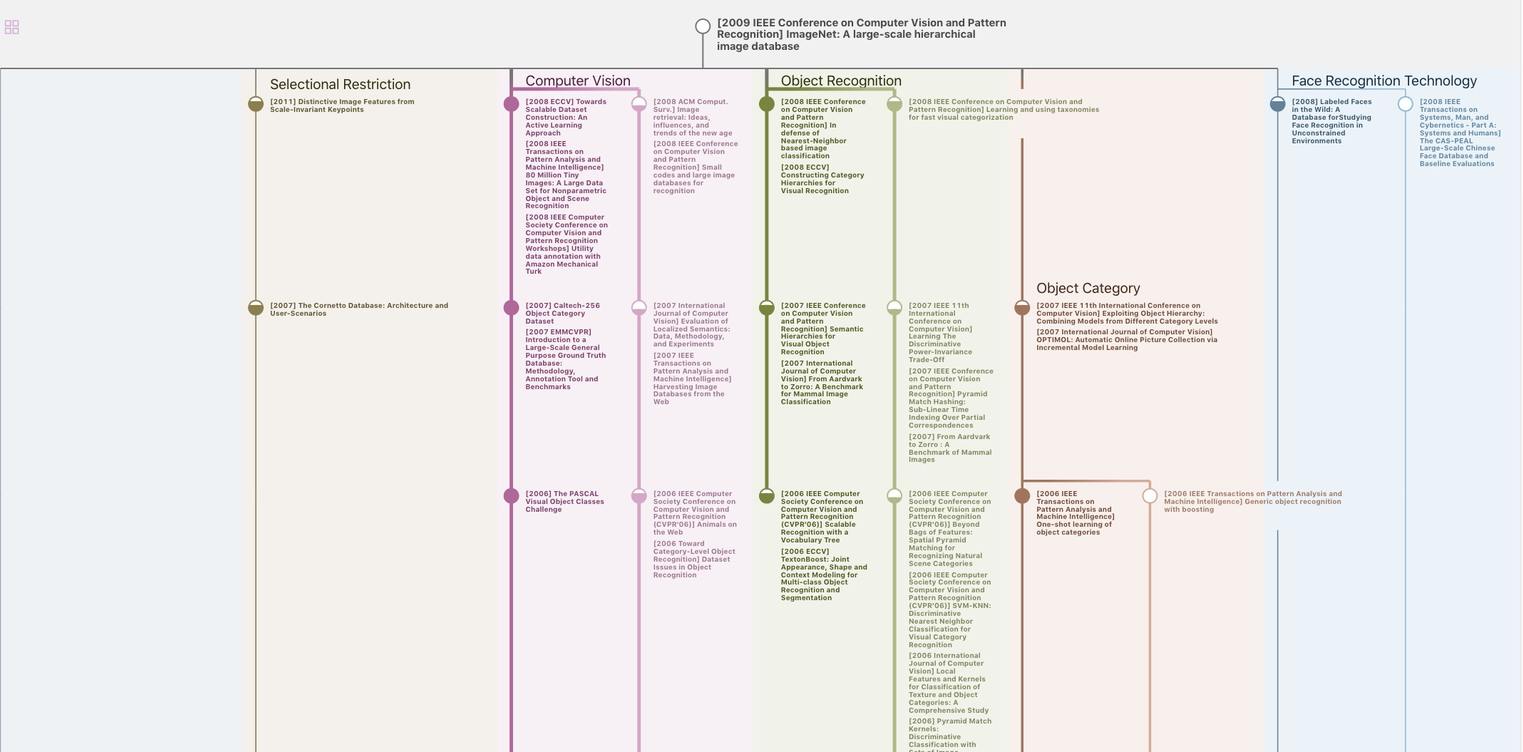
Generate MRT to find the research sequence of this paper
Chat Paper
Summary is being generated by the instructions you defined