Towards Explainable Dialogue System using Two-Stage Response Generation
ACM Transactions on Asian and Low-Resource Language Information Processing(2022)
摘要
In recent years, neural networks have achieved impressive performance on dialogue response generation. However, most of these models still suffer from some shortcomings, such as yielding uninformative responses and lacking explainable ability. The paper proposes a Two-Stage Dialogue Response Generation model (TSRG), which specifies a method to generate diverse and informative responses based on an interpretable procedure between stages. TSRG involves a two-stage framework that generates a candidate response first and then instantiates it as the final response. The positional information and a resident token are injected into the candidate response to stabilize the multi-stage framework, alleviating the shortcomings in the multi-stage framework. Additionally, TSRG allows adjusting and interpreting the interaction pattern between the two generation stages, making the generation response somewhat explainable and controllable. We evaluate the proposed model on three dialogue datasets that contain millions of single-turn message-response pairs between web users. The results show that, compared with the previous multi-stage dialogue generation models, TSRG can produce more diverse and informative responses and maintain fluency and relevance.
更多查看译文
关键词
Natural language processing,explainable text generation,neural response generation,Asian language
AI 理解论文
溯源树
样例
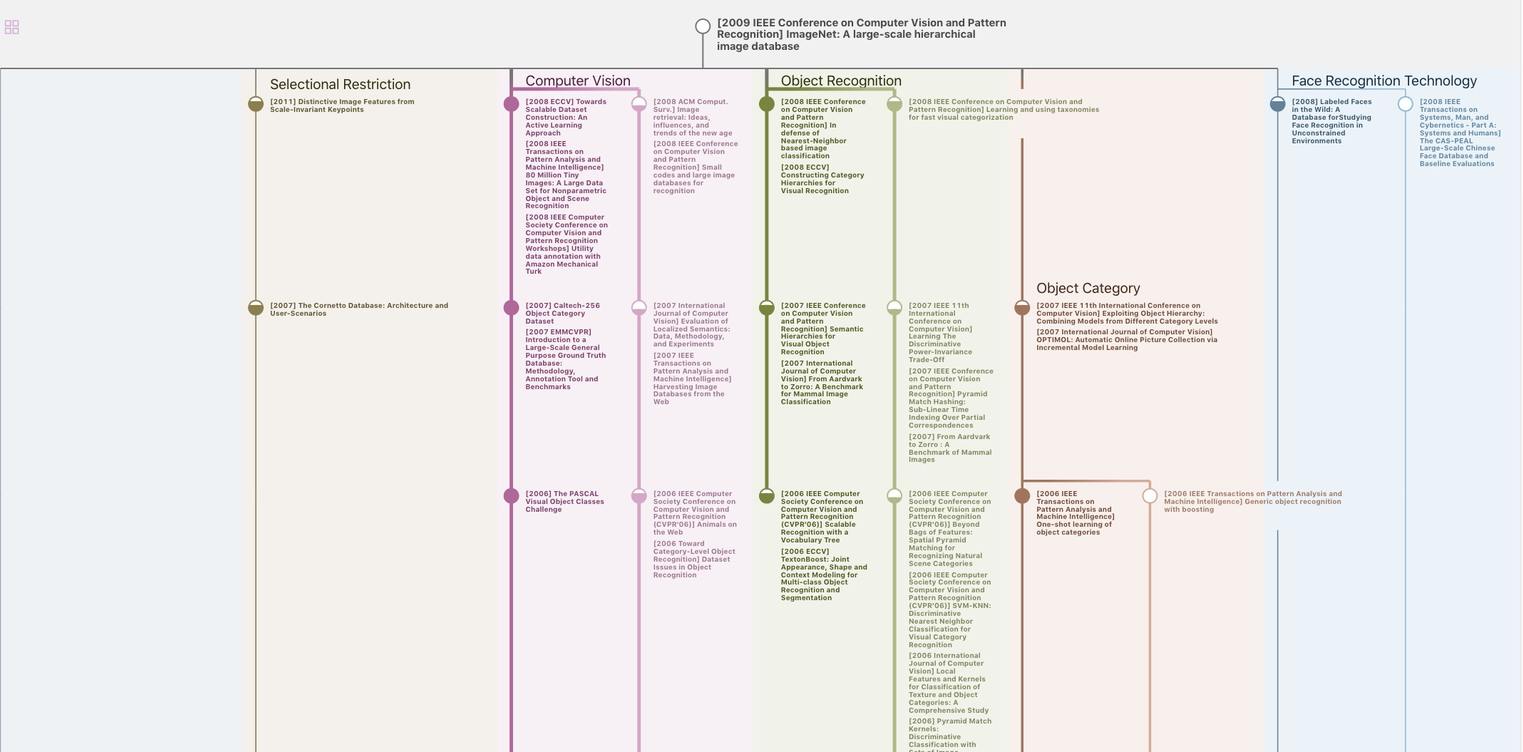
生成溯源树,研究论文发展脉络
Chat Paper
正在生成论文摘要