Spatial-Statistical Analysis of Landscape-Level Wildfire Rate of Spread
REMOTE SENSING(2022)
摘要
The objectives of this study were to evaluate spatial sampling and statistical aspects of landscape-level wildfire rate of spread (ROS) estimates derived from airborne thermal infrared imagery (ATIR). Wildfire progression maps and ROS estimates were derived from repetitive ATIR image sequences collected during the 2017 Thomas and Detwiler wildfire events in California. Three separate landscape sampling unit (LSU) sizes were used to extract remotely sensed environmental covariates known to influence fire behavior. Statistical relationships between fire spread rates and landscape covariates were analyzed using (1) bivariate regression, (2) multiple stepwise regression, (3) geographically weighted regression (GWR), (4) eigenvector spatial filtering (ESF) regression, (5) regression trees (RT), and (6) and random forest (RF) regression. GWR and ESF regressions reveal that relationships between covariates and ROS estimates are substantially non-stationary and suggest that the global association of fire spread controls are locally differentiated on landscape scales. Directional slope is by far the most strongly associated covariate of ROS for the imaging sequences analyzed and the size of LSUs has little influence on any of the covariate relationships.
更多查看译文
关键词
remote sensing,wildland fire,fire rate of spread,thermal imagery,spatial statistics,machine learning
AI 理解论文
溯源树
样例
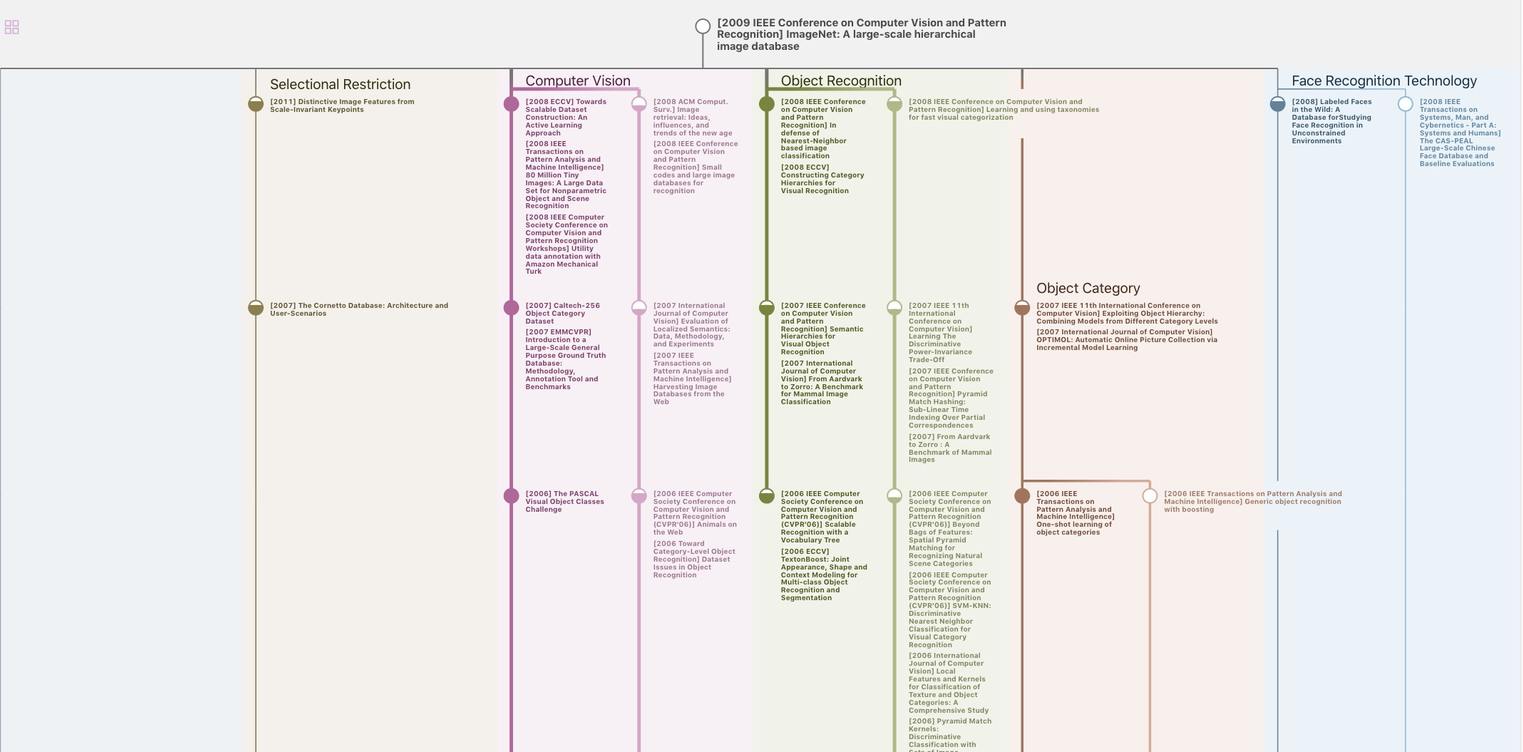
生成溯源树,研究论文发展脉络
Chat Paper
正在生成论文摘要