Solving Robotic Trajectory Sequential Writing Problem via Learning Character's Structural and Sequential Information
IEEE TRANSACTIONS ON CYBERNETICS(2024)
摘要
The writing sequence of numerals or letters often affects aesthetic aspects of the writing outcomes. As such, it remains a challenge for robotic calligraphy systems to perform, mimicking human writers' implicit intention. This article presents a new robot calligraphy system that is able to learn writing sequences with limited sequential information, producing writing results compatible to human writers with good diversity. In particular, the system innovatively applies a gated recurrent unit (GRU) network to generate robotic writing actions with the support of a prelabeled trajectory sequence vector. Also, a new evaluation method is proposed that considers the shape, trajectory sequence, and structural information of the writing outcome, thereby helping ensure the writing quality. A swarm optimization algorithm is exploited to create an optimal set of parameters of the proposed system. The proposed approach is evaluated using Arabic numerals, and the experimental results demonstrate the competitive writing performance of the system against state-of-the-art approaches regarding multiple criteria (including FID, MAE, PSNR, SSIM, and PerLoss), as well as diversity performance concerning variance and entropy. Importantly, the proposed GRU-based robotic motion planning system, supported with swarm optimization can learn from a small dataset, while producing calligraphy writing with diverse and aesthetically pleasing outcomes.
更多查看译文
关键词
Writing,Robots,Trajectory,Logic gates,Training,Feature extraction,Particle swarm optimization,Gated recurrent unit (GRU) network,robotic calligraphy,robotic motion planning
AI 理解论文
溯源树
样例
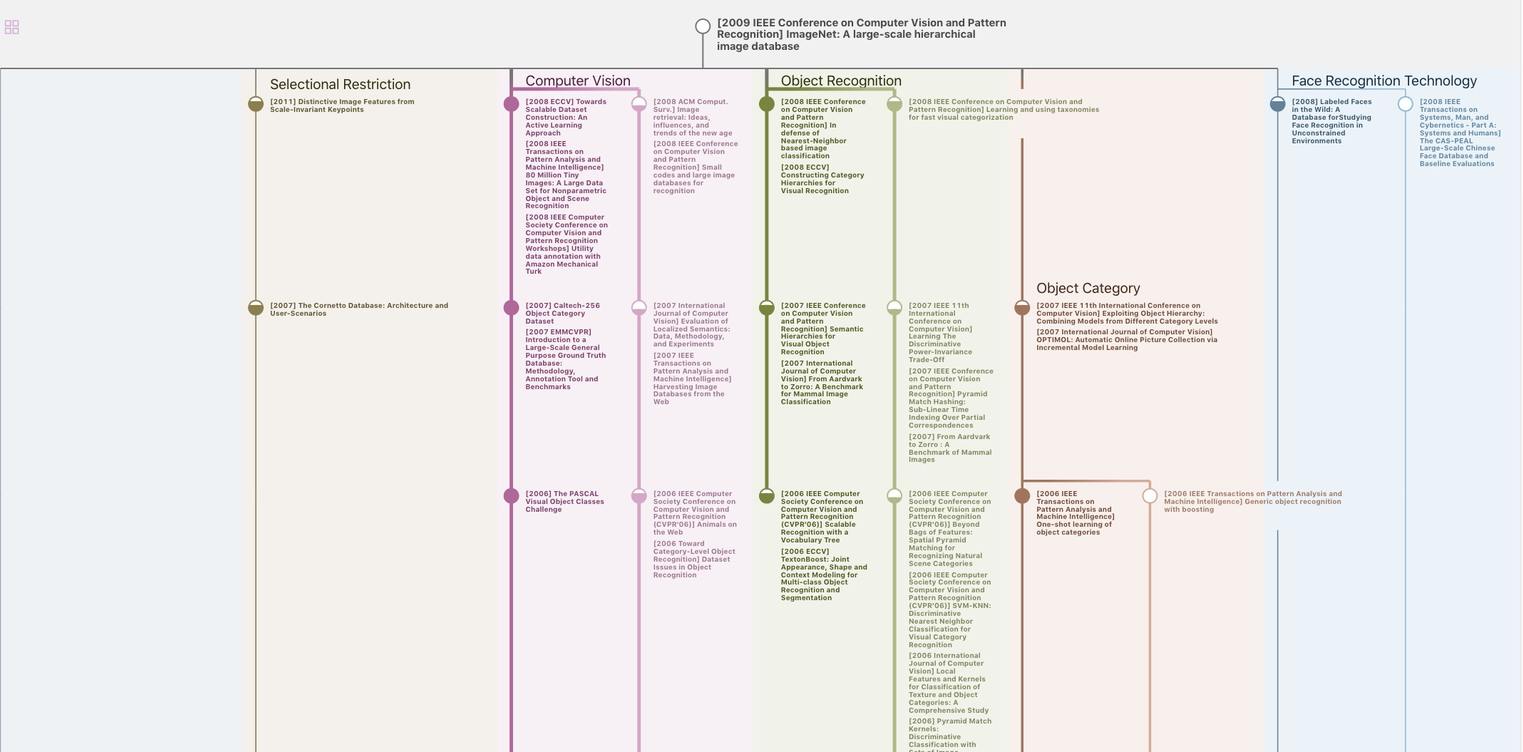
生成溯源树,研究论文发展脉络
Chat Paper
正在生成论文摘要