A new cross-domain prediction model of air pollutant concentration based on secure federated learning and optimized LSTM neural network
Environmental science and pollution research international(2022)
摘要
As air pollution worsens, the fast prediction of air pollutant concentration becomes increasingly important for public health. This paper proposes a new cross-domain prediction model of air pollutant concentration based on federated learning (FL), differential privacy laplace mechanism (DPLA) and long and short-term memory network optimized by sparrow search algorithm (SSA-LSTM), named FL-DPLA-SSA-LSTM. Firstly, with FL, SSA-LSTM is used as local training model for each city and predicts air pollutant concentration. Secondly, DPLA is used to add noise to the local model parameters, which can protect local data security. Then, the global model is updated by using the federated averaging algorithm (FedAvg). Lastly, FL is used to share global model for all cities, which can safely and quickly cross-domain predict air pollutant concentration. For data set, it is taken from hourly air pollutants and meteorological data from 12 cities in the Fenhe River and Weihe River Plains in China in 2020. The experimental results show that the prediction performance of the proposed model is significantly better than all comparison models. FedAvg updating with local model parameters with DPLA noise has little effect on the performance of the global model and even exceeds that of the global model. The calculation time of FL-DPLA-SSA-LSTM model is reduced by 99.95% compared with that of not using FL-DPLA machine learning model. It is proved that the model is high sharing and high safety, which greatly improves the training efficiency and has better generalization ability. It is significant for joint air pollution prevention and control and environmental protection.
更多查看译文
关键词
Air pollutant concentration prediction,Federated learning (FL),Differential privacy laplace mechanism (DPLA),Sparrow search algorithm (SSA),LSTM neural network,Joint prevention and control
AI 理解论文
溯源树
样例
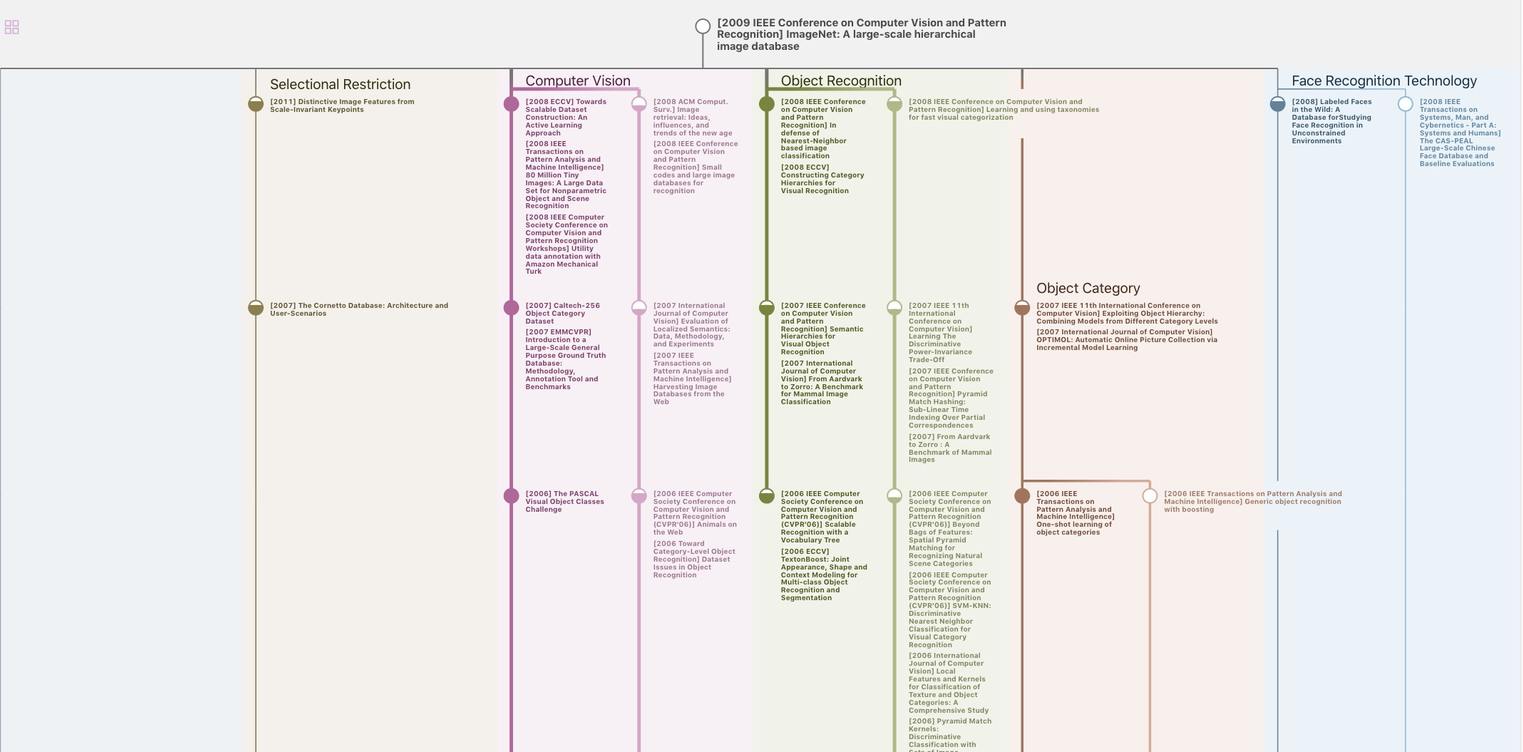
生成溯源树,研究论文发展脉络
Chat Paper
正在生成论文摘要