SEHLNet: Separate Estimation of High- and Low-Frequency components for Depth Completion
2022 International Conference on Robotics and Automation (ICRA)(2022)
摘要
Depth completion refers to inferring the dense depth map from a sparse depth map with or without corre-sponding color image. Numerous neural networks have been proposed to accomplish this task. However, insufficient uti-lization of heteromorphic data and the fact that predicted dense depth prefers a sparse depth enormously damage the performance of approaches. To reduce data preference and fully utilize two modalities, this paper proposes a novel network that predicts high- and low-frequency components of dense depth separately. Specifically, the framework consists of a Low-Frequency(LF) branch and a High-Frequency(HF) branch. In the LF branch, we recover the low-frequency depth component from sparse depth through an Adaptive Graph-Generate Graph Attention Network, which can be seen as a low-pass filter. In the HF branch, we model the high-frequency component, e.g. boundaries, as residuals to mitigate the impact of data preferences. Moreover, in this branch, we propose an Attention-based Self-Fusion mechanism to efficiently fuse multi-scale features extracted from the sparse depth and color image. Extensive experiments demonstrate that our approach achieves state-of-the-art performance on the KITTI benchmark and ranks 1st in root mean squared error among other published approaches.
更多查看译文
关键词
depth,completion,separate estimation,low-frequency
AI 理解论文
溯源树
样例
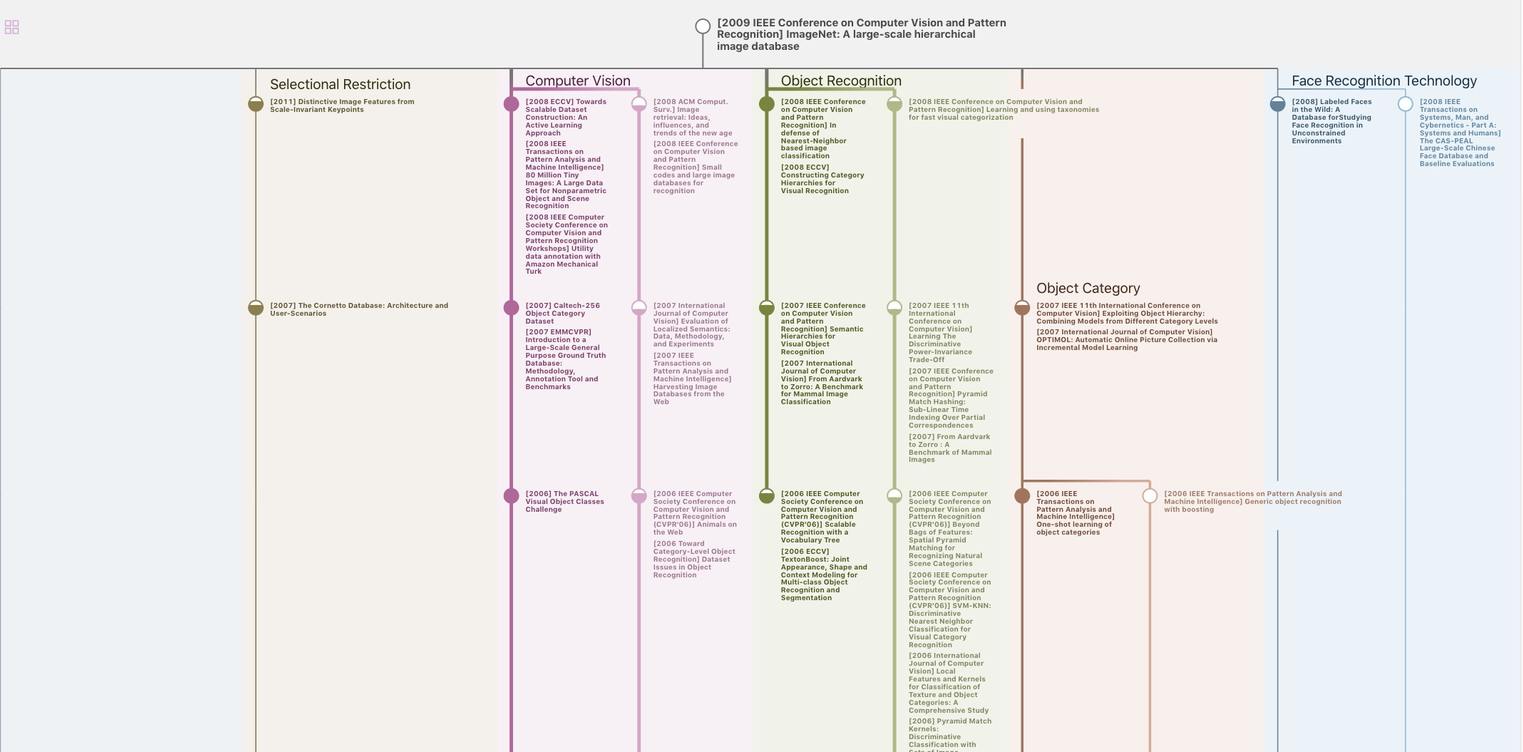
生成溯源树,研究论文发展脉络
Chat Paper
正在生成论文摘要