Model-driven reinforcement learning and action dimension extension method for efficient asymmetric assembly
IEEE International Conference on Robotics and Automation(2022)
Abstract
Complex assembly tasks remain huge challenge for robots because the traditional control methods rely on complicated contact state analysis. Reinforcement learning (RL) becomes one of the preferred embodiments to construct the control strategy of complex tasks. In this paper, the method of model-driven RL (MDRL) is employed to construct the control strategy. Then a completely innovative action dimension extension (ADE) mechanism is proposed to further accelerate the training process of RL. The simulation and experiment results demonstrate that the control strategy obtained through combining MDRL and ADE guarantees a more compliant assembly process. Besides, ADE method will enhance the data-efficiency of RL algorithms greatly (about 30%~40%) as well as increase the stable reward.
MoreTranslated text
Key words
robot force control,assembly,model-driven reinforcement learning,action dimension,efficient exploration
AI Read Science
Must-Reading Tree
Example
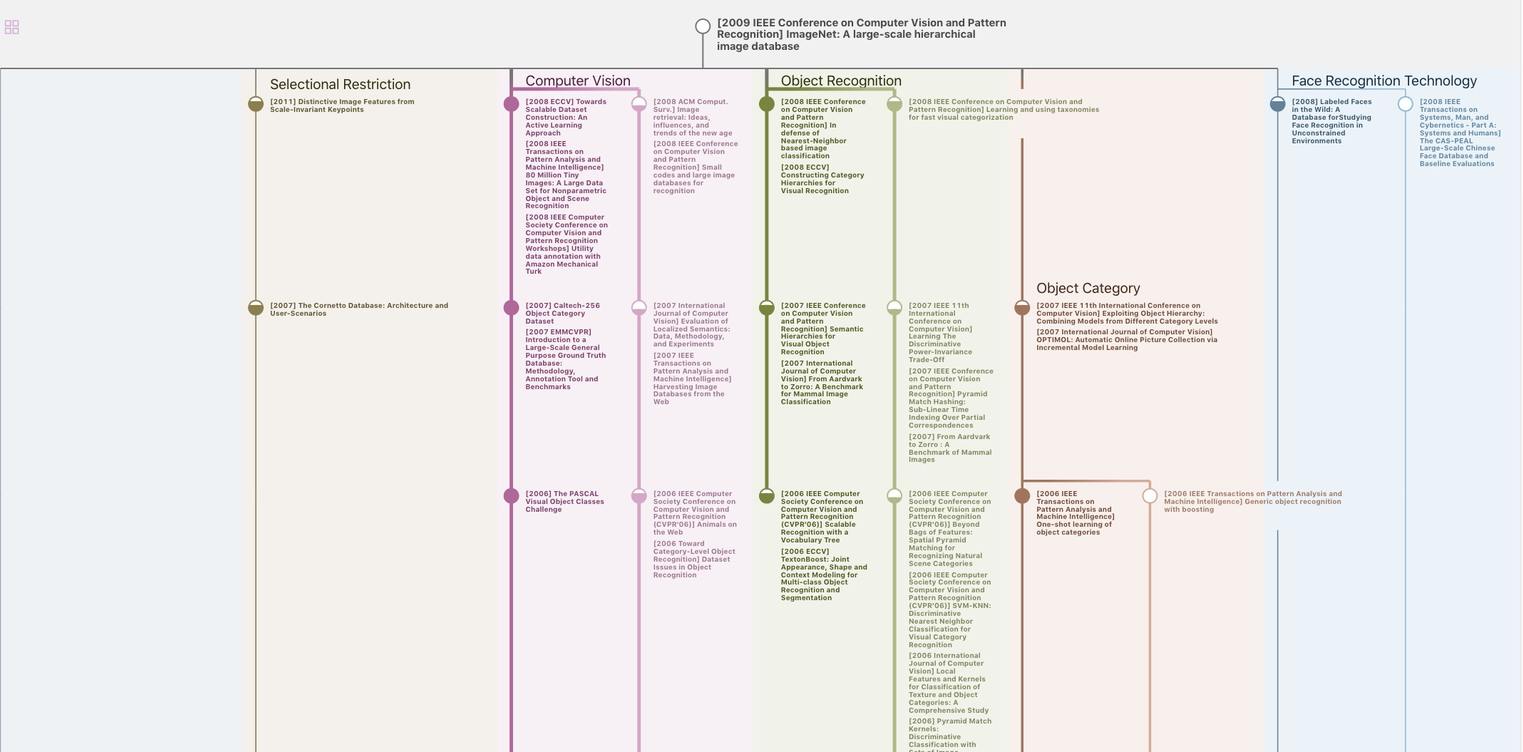
Generate MRT to find the research sequence of this paper
Chat Paper
Summary is being generated by the instructions you defined