Tightly-coupled GNSS-aided Visual-Inertial Localization
IEEE International Conference on Robotics and Automation(2022)
摘要
A navigation system which can output drift-free global trajectory estimation with local consistency holds great potential for autonomous vehicles and mobile devices. We propose a tightly-coupled GNSS-aided visual-inertial navigation system (GAINS) which is able to leverage the complementary sensing modality from a visual-inertial sensing pair, which provides high-frequency local information, and a Global Navigation Satellite System (GNSS) receiver with low-frequency global observations. Specifically, the raw GNSS measurements (including pseudorange, carrier phase changes, and Doppler frequency shift) are carefully leveraged and tightly fused within a visual-inertial framework. The proposed GAINS can accurately model the raw measurement uncertainties by canceling the atmospheric effects (e.g., ionospheric and tropospheric delays) which requires no prior model information. A robust state initialization procedure is presented to facilitate the fusion of global GNSS information with local visual-inertial odometry, and the spatiotemporal calibration between IMU-GNSS are also optimized in the estimator. The proposed GAINS is evaluated on extensive Monte-Carlo simulations on a trajectory generated from a large-scale urban driving dataset with specific verification for each component (i.e., online calibration and system initialization). GAINS also demonstrates competitive performance against existing state-of-the-art methods on a publicly available dataset with ground truth.
更多查看译文
关键词
global GNSS information,visual-inertial odometry,IMU-GNSS,online calibration,system initialization,GNSS-aided visual-inertial localization,output drift-free global trajectory estimation,local consistency,autonomous vehicles,GNSS-aided visual-inertial navigation system,complementary sensing modality,visual-inertial sensing pair,high-frequency local information,Global Navigation Satellite System receiver,low-frequency global observations,raw GNSS measurements,carrier phase changes,Doppler frequency shift,visual-inertial framework,raw measurement uncertainties,prior model information
AI 理解论文
溯源树
样例
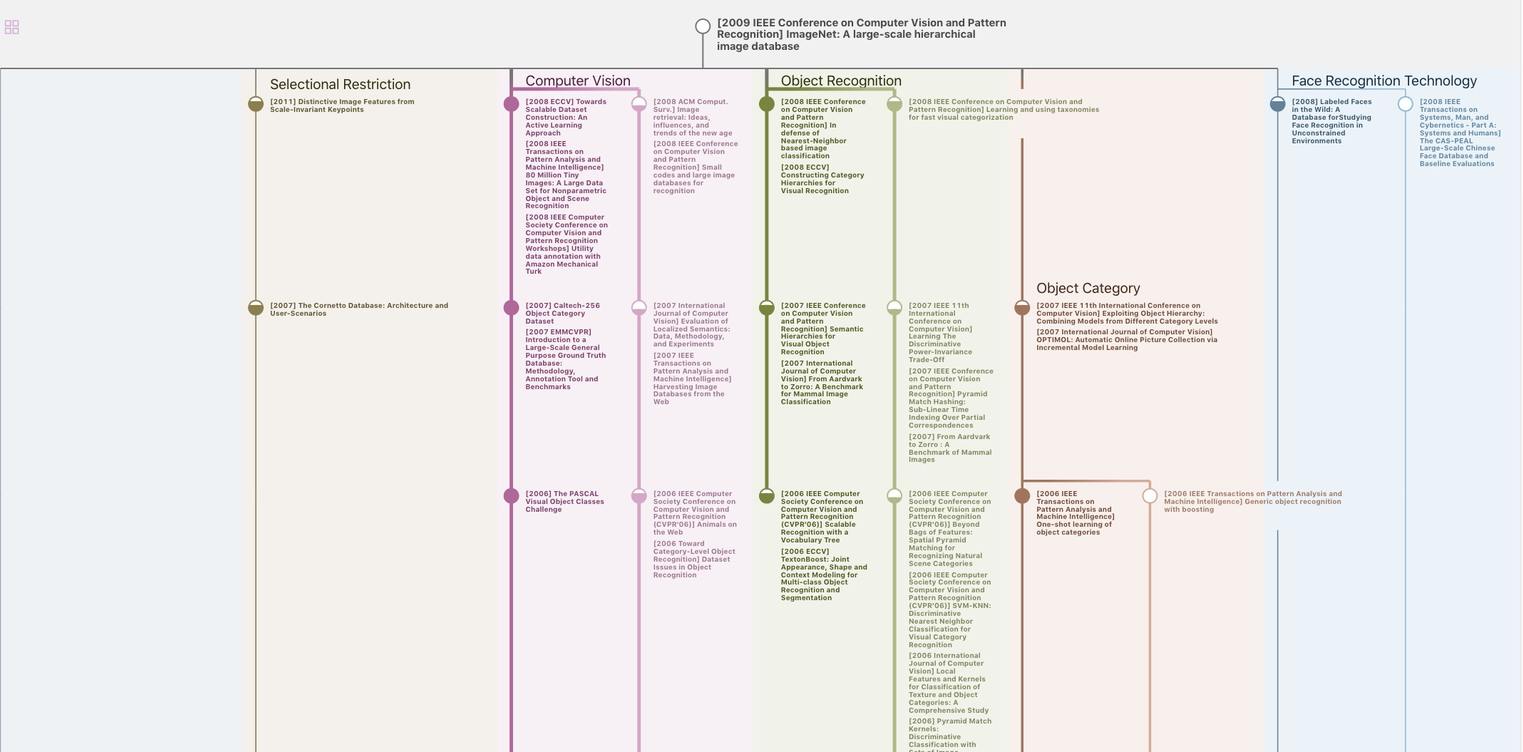
生成溯源树,研究论文发展脉络
Chat Paper
正在生成论文摘要