FEJ2: A Consistent Visual-Inertial State Estimator Design
IEEE International Conference on Robotics and Automation(2022)
摘要
In this paper, we propose a novel consistent state estimator design for visual-inertial systems. Motivated by first-estimates Jacobian (FEJ) based estimators - which uses the first-ever estimates as linearization points to preserve proper observability properties of the linearized estimator thereby improving the consistency - we carefully model measurement linearization errors due to its Jacobian evaluation and propose a methodology which still leverages FEJ to ensure the estimator's observability properties, but additionally explicitly compensate for linearization errors caused by poor first estimates. We term this estimator FEJ2, which directly addresses the discrepancy between the best Jacobian evaluated at the latest state estimate and the first-estimates Jacobian evaluated at the first-time-ever state estimate. We show that this process explicitly models that the FEJ used is imperfect and thus contributes additional error which, as in FEJ2, should be modeled and consistently increase the state covariance during update. The proposed FEJ2 is evaluated against state-of-the-art visual-inertial estimators in both Monte-Carlo simulations and real-world experiments, which has been shown to outperform existing methods and to robustly handle poor first estimates and high measurement noises.
更多查看译文
关键词
visual-inertial
AI 理解论文
溯源树
样例
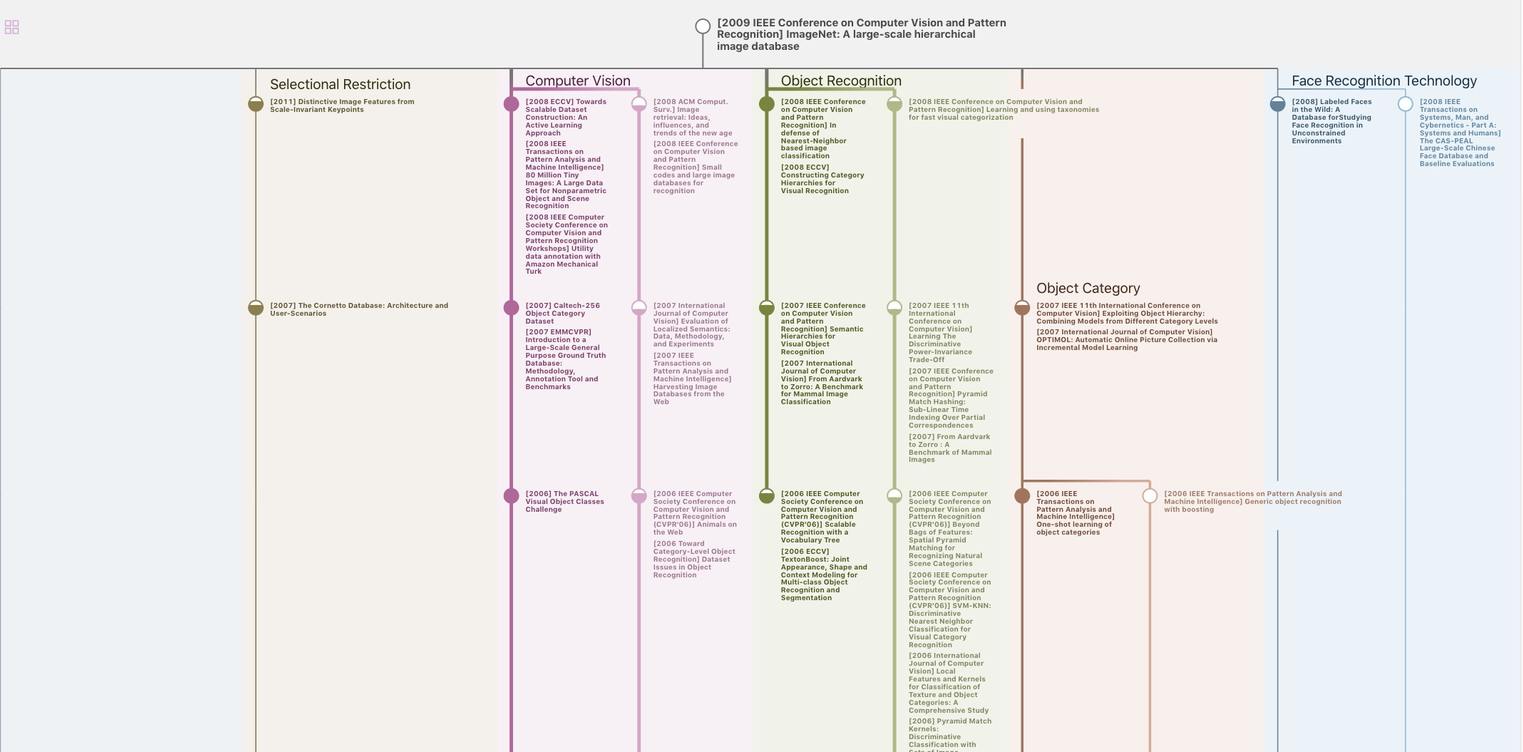
生成溯源树,研究论文发展脉络
Chat Paper
正在生成论文摘要