Deep Networks for Point Cloud Map Validation
IEEE International Conference on Robotics and Automation(2022)
摘要
Modern SLAM engines typically rely on high-end sensor rigs and robust algorithms to guarantee the high-quality requirements that self-driving cars and other complex autonomous systems require from 3D point cloud maps. Nonetheless, multiple factors can impact the reconstruction quality and it is not uncommon to end up with generally consistent maps affected by local distortions and artifacts, especially when mapping increasingly larger environments. We tackle the problem of identifying these low-consistency areas in point cloud maps by analyzing the quality of pair-wise point cloud alignments. Rather than relying on geometric consistency analysis or visual inspection, we leverage on deep point networks and formulate the validation as a binary classification problem, allowing us to quickly and effectively identify areas of improvement.
更多查看译文
关键词
low-consistency areas,point cloud maps,pair-wise point cloud alignments,geometric consistency analysis,deep point networks,deep networks,point cloud map validation,modern SLAM engines,high-end sensor rigs,robust algorithms,high-quality requirements,complex autonomous systems,reconstruction quality,generally consistent maps,local distortions
AI 理解论文
溯源树
样例
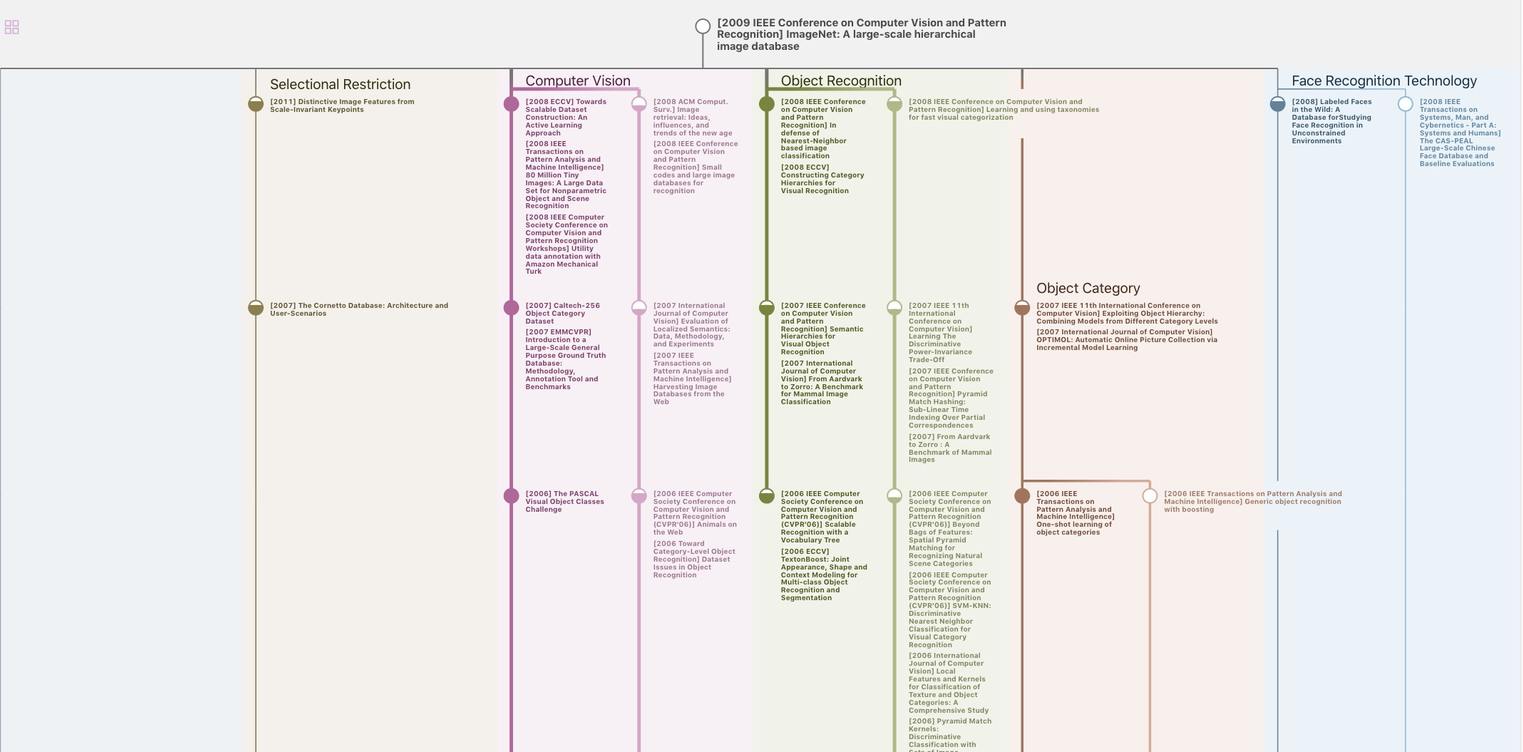
生成溯源树,研究论文发展脉络
Chat Paper
正在生成论文摘要