Locally Normalized Soft Contrastive Clustering for Compact Clusters
European Conference on Artificial Intelligence (ECAI)(2022)
摘要
Linear temporal logic over finite traces (LTLf) satisfiability checking is a fundamental and hard (PSPACE-complete) problem in the artificial intelligence community. We explore teaching end-to-end neural networks to check satisfiability in polynomial time. It is a challenge to characterize the syntactic and semantic features of LTLf via neural networks. To tackle this challenge, we propose LTLfNet, a recursive neural network that captures syntactic features of LTLf by recursively combining the embeddings of sub-formulae. LTLfNet models permutation invariance and sequentiality in the semantics of LTLf through different aggregation mechanisms of sub-formulae. Experimental results demonstrate that LTLfNet achieves good performance in synthetic datasets and generalizes across large-scale datasets. They also show that LTLfNet is competitive with state-of-the-art symbolic approaches such as nuXmv and CDLSC.
更多查看译文
关键词
soft contrastive clustering,clusters
AI 理解论文
溯源树
样例
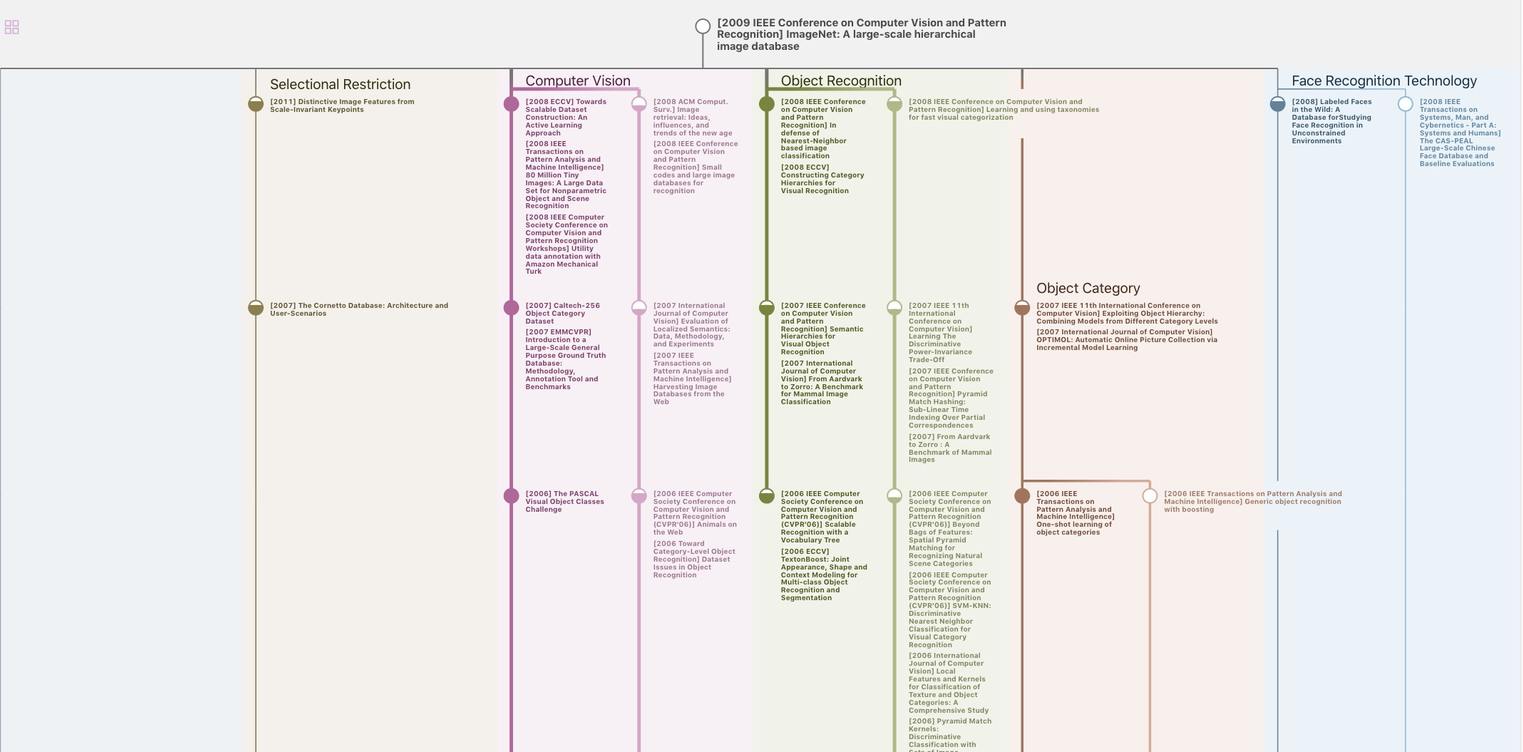
生成溯源树,研究论文发展脉络
Chat Paper
正在生成论文摘要