Parameters exploration of SOFC for dynamic simulation using adaptive chaotic grey wolf optimization algorithm
Energy(2022)
摘要
One of the most important indicators of solid oxide fuel cells (SOFC) is to maximize their production capacity. In this paper, the optimal values of SOFC unit are achieved using the combined model of the multi-layer perceptron neural network and different meta-heuristic optimization algorithms, including particle swarm optimization, ant colony optimization algorithm, genetic algorithm, Grey Wolf Optimizer, Multi-Verse Optimization algorithm, Accelerated Grey Wolf Optimization, Chaotic Grey Wolf Optimization, and Adaptive Chaotic Grey Wolf optimization hybrid algorithm. This article calculates the optimal decision factors such as power, temperature, voltage, and electrolyte thickness. The fuel cell modeling method is done by neural network training based on available experimental data. And the hyper-parameters of the neural network are obtained by optimization algorithms. Standard Error (SE), Mean Squared Error (MSE), Mean Absolute Percentage Error (MAPE), Root Mean Squared Error (RMSE), Mean Absolute Error (MAE), Relative absolute error (RAE), Pearson's correlation coefficient (R), and coefficient of determination (R2) are applied as evaluation criteria. Results are presented for testing, learning, and total data mode. Comparison between the proposed method results and other well-known algorithms showed that the Adaptive Chaotic Grey Wolf optimization hybrid algorithm was superior in terms of performance criteria.
更多查看译文
关键词
Solid oxide fuel cell,Grey wolf optimizer,Algorithm identification,Performance improvement
AI 理解论文
溯源树
样例
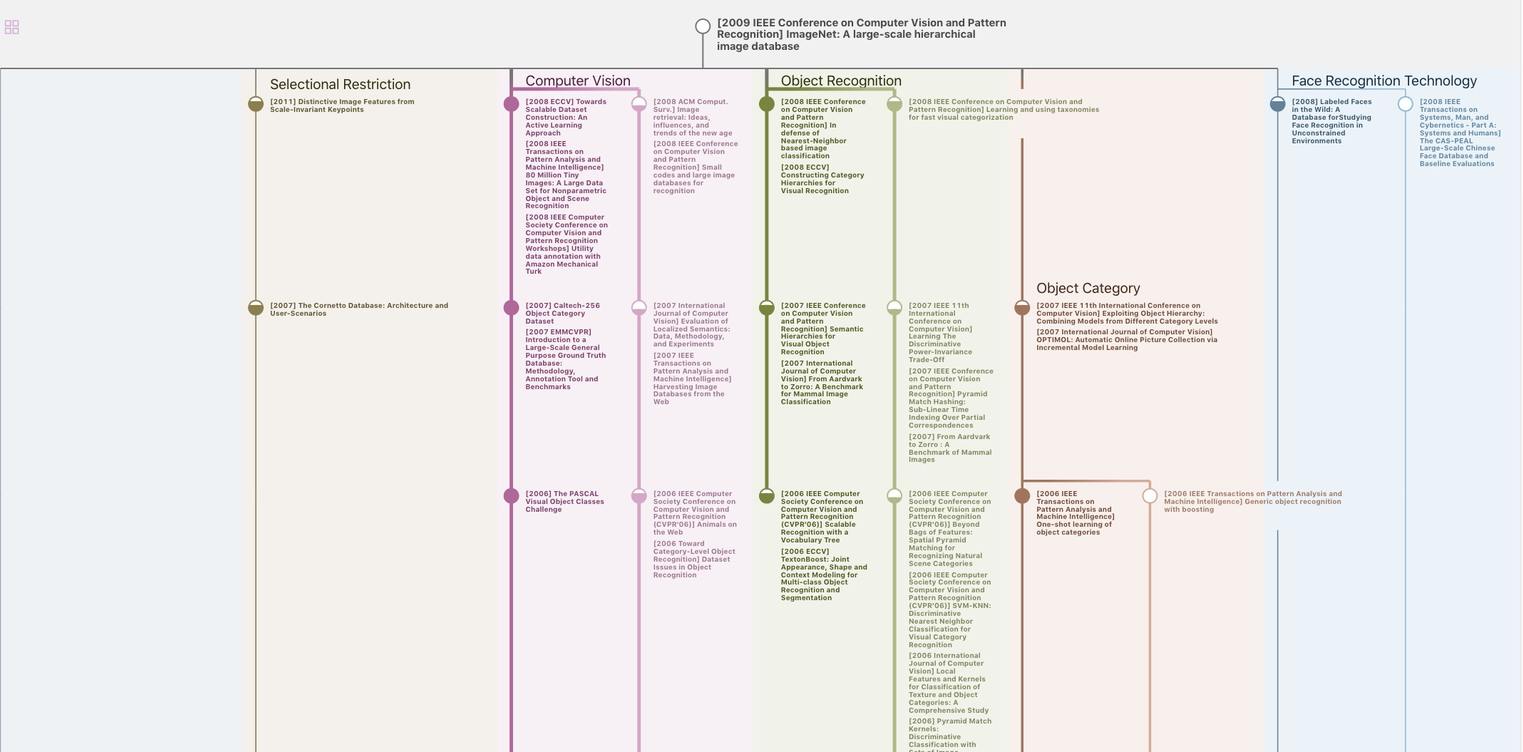
生成溯源树,研究论文发展脉络
Chat Paper
正在生成论文摘要