Computationally Efficient Robust Model Predictive Control for Uncertain System Using Causal State-Feedback Parameterization
arxiv(2022)
摘要
This paper investigates the problem of robust model predictive control (RMPC) of linear-time-invariant (LTI) discrete-time systems subject to structured uncertainty and bounded disturbances. Typically, the constrained RMPC problem with state-feedback parameterizations is nonlinear (and nonconvex) with a prohibitively high computational burden for online implementation. To remedy this, a novel approach is proposed to linearize the state-feedback RMPC problem, with minimal conservatism, through the use of semidefinite relaxation techniques. The proposed algorithm computes the state-feedback gain and perturbation online by solving a linear matrix inequality (LMI) optimization that, in comparison to other schemes in the literature is shown to have a substantially reduced computational burden without adversely affecting the tracking performance of the controller. Additionally, an offline strategy that provides initial feasibility on the RMPC problem is presented. The effectiveness of the proposed scheme is demonstrated through numerical examples from the literature.
更多查看译文
关键词
Elimination lemma,linear matrix inequalities,robust model predictive control (RMPC),semidefinite relaxation,state-feedback control,uncertain systems
AI 理解论文
溯源树
样例
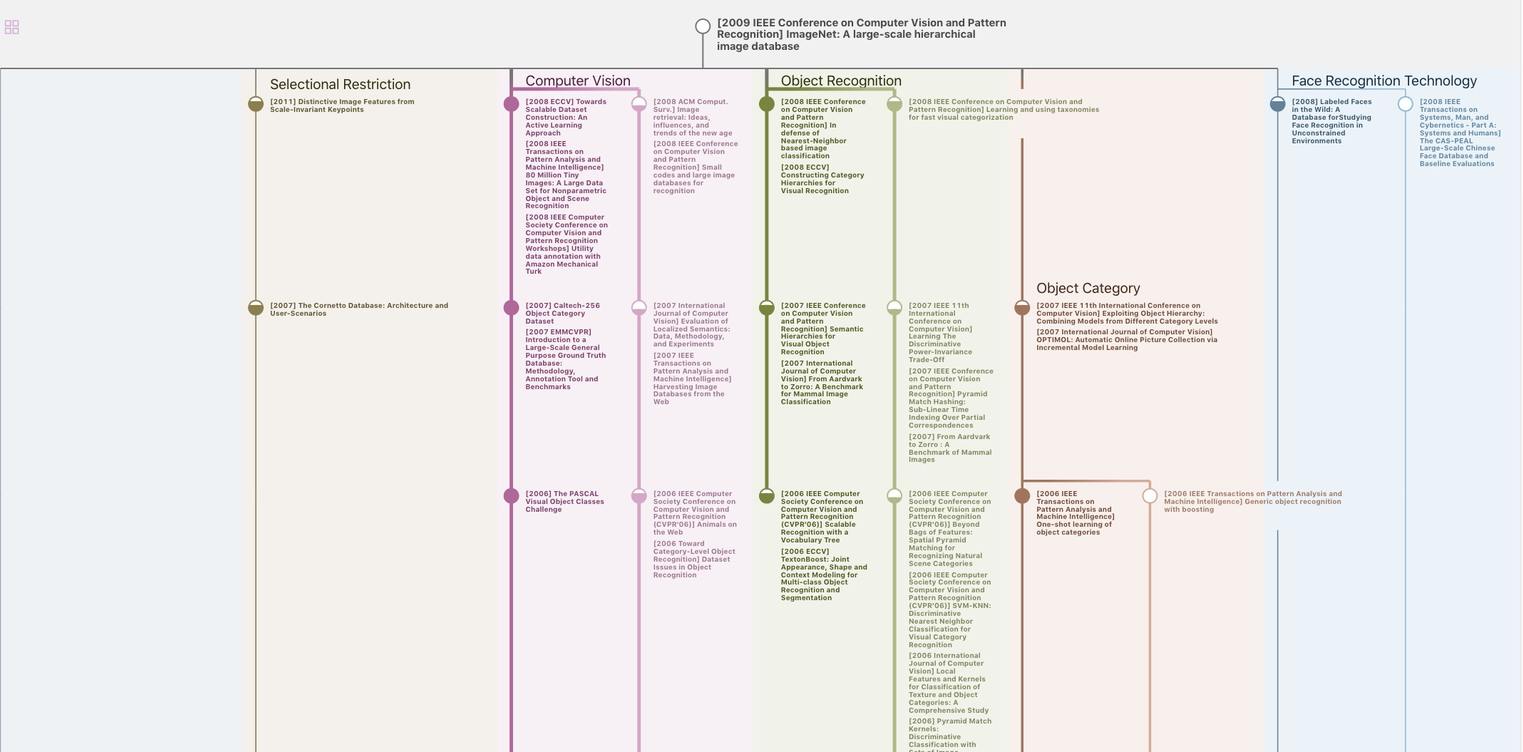
生成溯源树,研究论文发展脉络
Chat Paper
正在生成论文摘要