A study on quality control using delta data with machine learning technique
HELIYON(2022)
摘要
Background: In the big data era, patient-based real-time quality control (PBRTQC), as an emerging quality control (QC) method, is expanding within the clinical laboratory industry. However, the main issue of current PBRTQC methodology is data stability. Our study is aimed to explore a novel protocol for data stability by combining delta data with machine learning (ML) technique to improve the capacity of QC event detection.Methods: A data set of 423,290 laboratory results from Beijing Chao-yang Hospital 2019 patient results were used as a training set (n = 380960, 90%) and internal validation set (n = 42330, 10%). A further 22,460 results from Beijing Long-fu Hospital 2019 patient results were used as a test set. Three-type data (1) Single-type data pro-cessed by truncation limits; (2) delta-type data processed by truncation limits and (3)delta-type data processed by Isolated Forest (IF) algorithm were evaluated with accuracy, sensitivity, NPed, etc., and compared with previously published statistical methods. Results: The optimal model was based on Random Forest (RF) algorithm by using delta-type data processed by IF algorithm. The model had a better accuracy (0.99), sensitivity (0.99) specificity (0.99) and AUC (0.99) with the dependent test set, surpassing the critical bias of PBRTQC by over 50%. For the LYMPH#, HGB, and PLT, the cumulative MNPed of MLQC were reduced by 95.43%, 97.39%, and 97.97% respectively when compared to the best of the PBRTQC. Conclusion: Final results indicate that by integrating an innovative ML algorithm with the overall data processing protocol the detection of QC events is improved.
更多查看译文
关键词
Delta data, Machine learning, Random forest, Data processing, Patient-based real-time quality control
AI 理解论文
溯源树
样例
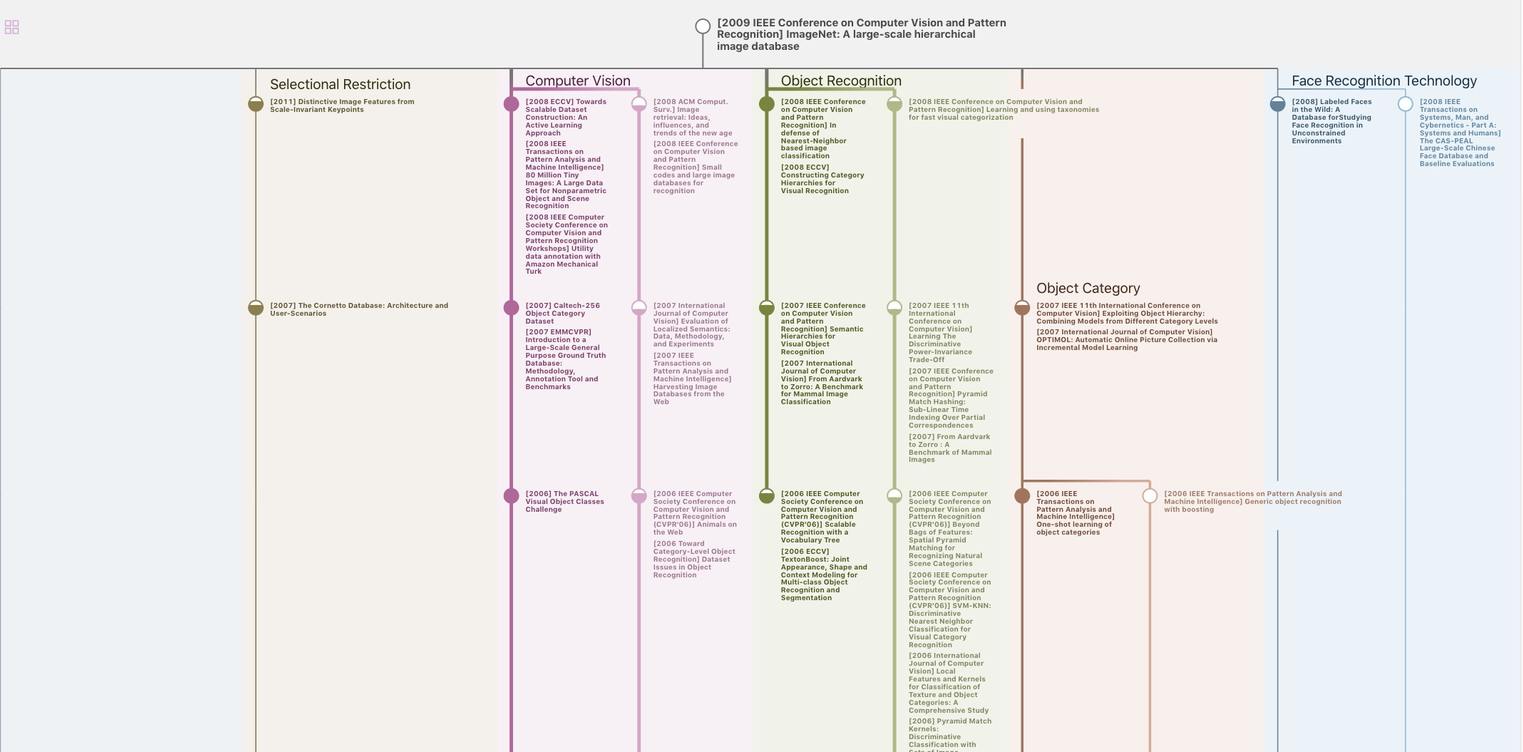
生成溯源树,研究论文发展脉络
Chat Paper
正在生成论文摘要