Least-squares RTM with shifted total variation regularization for depth imaging of sparse short-offset seismic data
Second International Meeting for Applied Geoscience & Energy(2022)
Abstract
PreviousNext No AccessSecond International Meeting for Applied Geoscience & EnergyLeast-squares RTM with shifted total variation regularization for depth imaging of sparse short-offset seismic dataAuthors: Toktam ZandAndrzej GorszczykAli GholamiHasan GhasemzadehAlison MalcolmToktam ZandPolish Academy of Sciences and K. N. Toosi University of TechnologySearch for more papers by this author, Andrzej GorszczykPolish Academy of Sciences and Université Grenoble AlpesSearch for more papers by this author, Ali GholamiUniversity of TehranSearch for more papers by this author, Hasan GhasemzadehK. N. Toosi University of TechnologySearch for more papers by this author, and Alison MalcolmMemorial University of NLSearch for more papers by this authorhttps://doi.org/10.1190/image2022-3751356.1 SectionsAboutPDF/ePub ToolsAdd to favoritesDownload CitationsTrack CitationsPermissions ShareFacebookTwitterLinked InRedditEmail AbstractLeast-squares reverse time migration (LSRTM) is a leading tool in imaging complex geological structures. This is because it includes the full-wave equation via reverse time migration (RTM), and solves the linearized wave equation by the least-squares (LS) minimization. To stabilize the LSRTM solution and mitigate the shortcomings that arise from linearization, simplification, approximation in simulation, and imperfection of data acquisition an appropriate regularization must be used. However, the conventional regularization methods such as Tikhonov, total variation (TV), or sparse regularization perform suboptimally, even in recovering structurally simple earth models. Here we introduce a new extension of Tikhonov-TV compound regularization, called shifted TV, to regularize the unknown reflectivity image. This new regularization implemented in the frame of a nonlinear migration allows for high-resolution and stable imaging in the presence of rough migration velocity models. We demonstrate the performance of our method using a short offset academic-style streamer data generated in the crustal-scale GO 3D OBS synthetic subduction zone model. The results confirm that the shifted regularization increases the robustness of LSRTM with an imperfect background velocity model and allows us to estimate high-quality reflectivity images of complex geological setting despite the limited streamer length.Note: This paper was accepted into the Technical Program but was not presented at IMAGE 2022 in Houston, Texas.Keywords: reverse-time migration, least-squares migration, regularization, shifted total variationPermalink: https://doi.org/10.1190/image2022-3751356.1FiguresReferencesRelatedDetails Second International Meeting for Applied Geoscience & EnergyISSN (print):1052-3812 ISSN (online):1949-4645Copyright: 2022 Pages: 3694 publication data© 2022 Published in electronic format with permission by the Society of Exploration Geophysicists and the American Association of Petroleum GeologistsPublisher:Society of Exploration Geophysicists HistoryPublished Online: 15 Aug 2022 CITATION INFORMATION Toktam Zand, Andrzej Gorszczyk, Ali Gholami, Hasan Ghasemzadeh, and Alison Malcolm, (2022), "Least-squares RTM with shifted total variation regularization for depth imaging of sparse short-offset seismic data," SEG Technical Program Expanded Abstracts : 2714-2718. https://doi.org/10.1190/image2022-3751356.1 Plain-Language Summary Keywordsreverse-time migrationleast-squares migrationregularizationshifted total variationPDF DownloadLoading ...
MoreTranslated text
Key words
total variation regularization,depth imaging,least-squares,short-offset
AI Read Science
Must-Reading Tree
Example
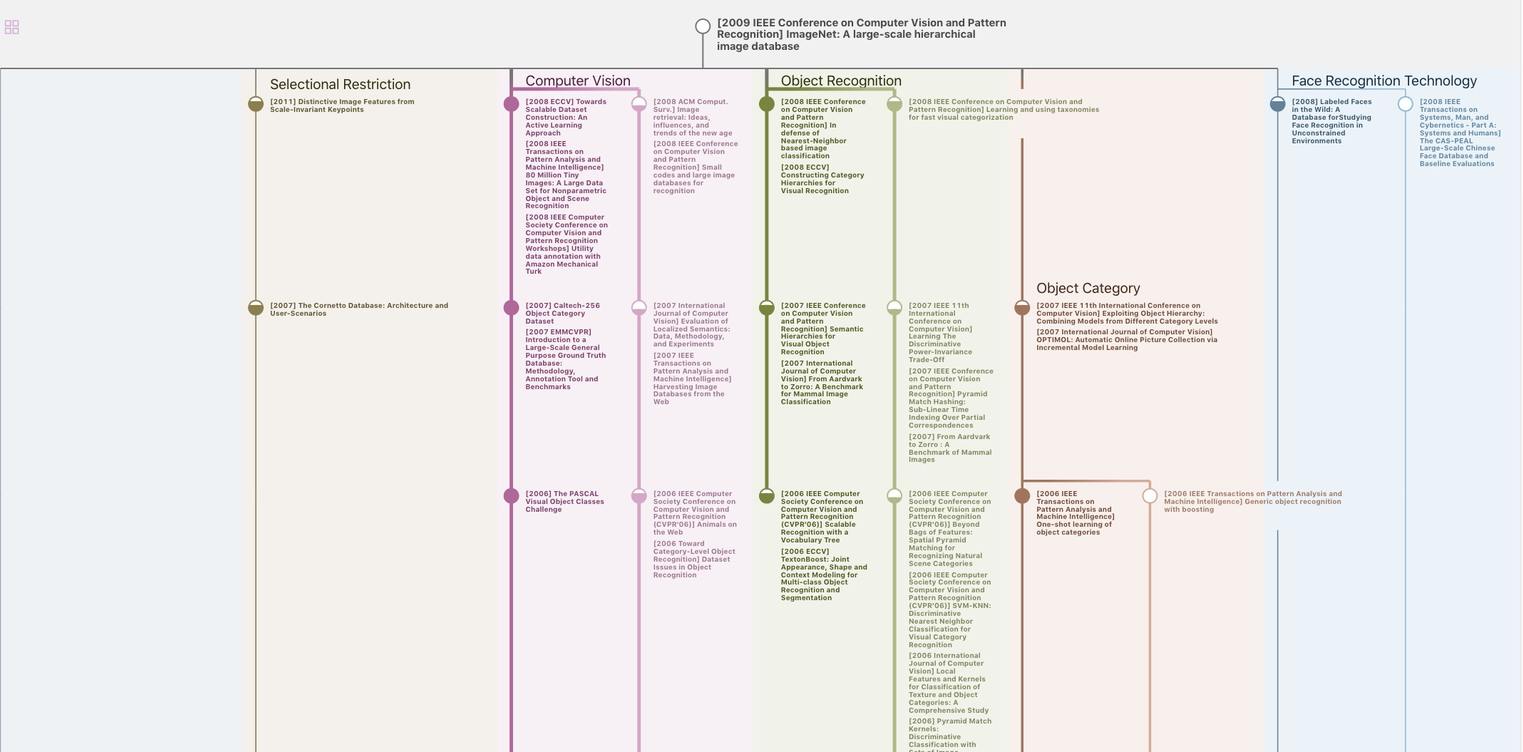
Generate MRT to find the research sequence of this paper
Chat Paper
Summary is being generated by the instructions you defined