Above-Ground Biomass Prediction for Croplands at a Sub-Meter Resolution Using UAV-LiDAR and Machine Learning Methods
REMOTE SENSING(2022)
摘要
Current endeavors to enhance the accuracy of in situ above-ground biomass (AGB) prediction for croplands rely on close-range monitoring surveys that use unstaffed aerial vehicles (UAVs) and mounted sensors. In precision agriculture, light detection and ranging (LiDAR) technologies are currently used to monitor crop growth, plant phenotyping, and biomass dynamics at the ecosystem scale. In this study, we utilized a UAV-LiDAR sensor to monitor two crop fields and a set of machine learning (ML) methods to predict real-time AGB over two consecutive years in the region of Mid-Jutland, Denmark. During each crop growing period, UAV surveys were conducted in parallel with AGB destructive sampling every 7-15 days, the AGB samples from which were used as the ground truth data. We evaluated the ability of the ML models to estimate the real-time values of AGB at a sub-meter resolution (0.17-0.52 m(2)). An extremely randomized trees (ERT) regressor was selected for the regression analysis, based on its predictive performance for the first year's growing season. The model was retrained using previously identified hyperparameters to predict the AGB of the crops in the second year. The ERT performed AGB estimation using height and reflectance metrics from LiDAR-derived point cloud data and achieved a prediction performance of R-2 = 0.48 at a spatial resolution of 0.35 m(2). The prediction performance could be improved significantly by aggregating adjacent predictions (R-2 = 0.71 and R-2 = 0.93 at spatial resolutions of 1 m(2) and 2 m(2), respectively) as they ultimately converged to the reference biomass values because any individual errors averaged out. The AGB prediction results were examined as function of predictor type, training set size, sampling resolution, phenology, and canopy density. The results demonstrated that when combined with ML regression methods, the UAV-LiDAR method could be used to provide accurate real-time AGB prediction for crop fields at a high resolution, thereby providing a way to map their biochemical constituents.
更多查看译文
关键词
UAV-LiDAR,machine learning,above-ground biomass,precision agriculture
AI 理解论文
溯源树
样例
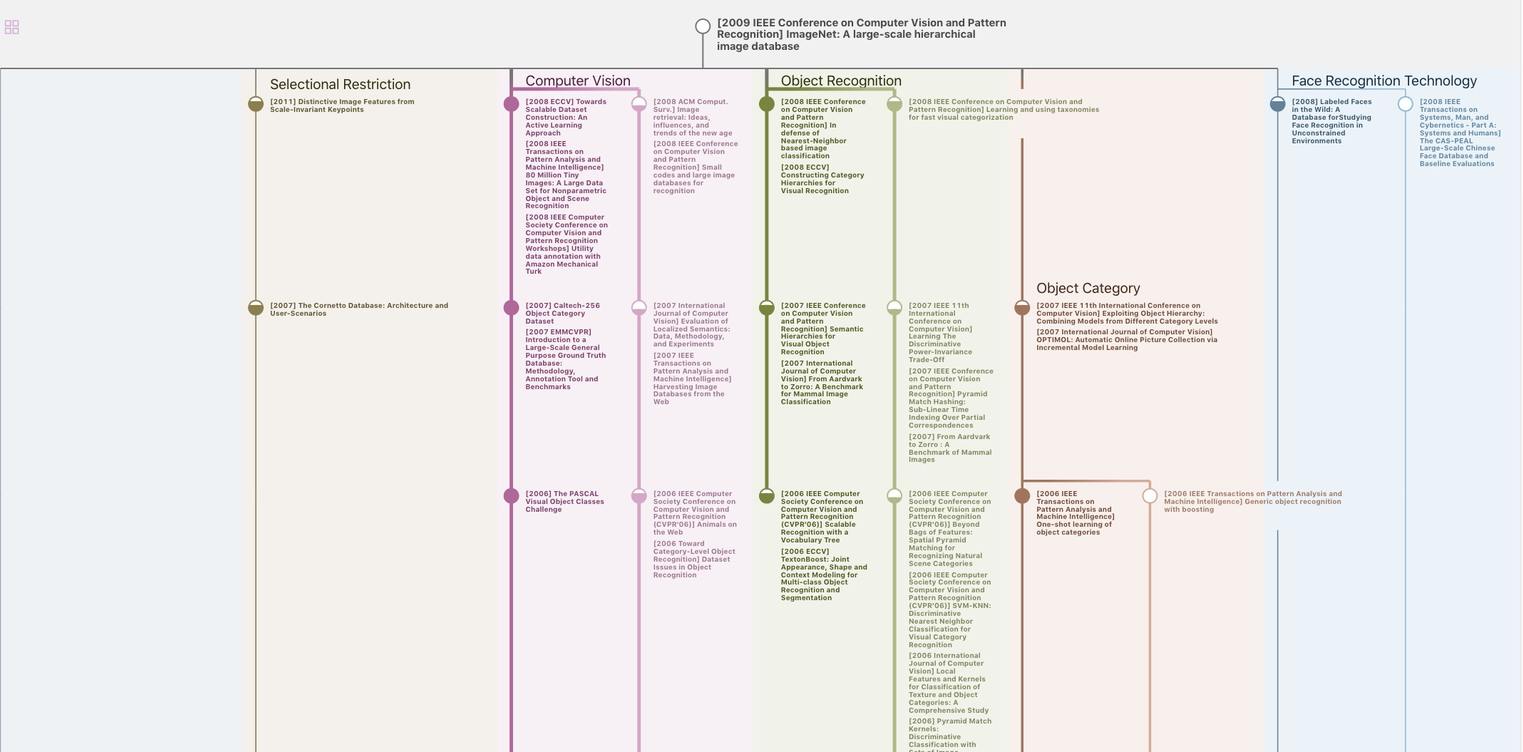
生成溯源树,研究论文发展脉络
Chat Paper
正在生成论文摘要