Deep learning Laplace-Fourier full-waveform inversion with virtual supershot gathers
Second International Meeting for Applied Geoscience & Energy(2022)
Abstract
PreviousNext No AccessSecond International Meeting for Applied Geoscience & EnergyDeep learning Laplace-Fourier full-waveform inversion with virtual supershot gathersAuthors: Lei FuDaniele ColomboWeichang LiErnesto Sandoval-CurielErsan TurkogluLei FuAramco AmericasSearch for more papers by this author, Daniele ColomboSaudi AramcoSearch for more papers by this author, Weichang LiAramco AmericasSearch for more papers by this author, Ernesto Sandoval-CurielSaudi AramcoSearch for more papers by this author, and Ersan TurkogluSaudi AramcoSearch for more papers by this authorhttps://doi.org/10.1190/image2022-3745792.1 SectionsAboutPDF/ePub ToolsAdd to favoritesDownload CitationsTrack CitationsPermissions ShareFacebookTwitterLinked InRedditEmail AbstractIn the past decade, full waveform inversion (FWI) has become a powerful tool to obtain high-resolution subsurface velocity and imaging. The conventional way to solve this ill- posed inverse problem is through iterative algorithms. However, it suffers issues like high computational cost and cycle-skipping. Additionally, the application of FWI to land seismic data faces difficulties related to the complex physics, unknown and spatially varying source signatures, and low signal-to-noise ratio (SNR) in the data. In this study, we propose a new method to reconstruct the velocity model from seismic data organized in the virtual super gathers (VSG) in the Laplace-Fourier domain by deep learning network (DNN). The proposed new method is applied to a synthetic data experiment, which demonstrates that the application of DNN on VSG in Laplace-Fourier domain provides an effective solution for high-resolution velocity mapping in complex near-surface conditions.Keywords: machine learning, deep learning, full-waveform inversion, near surface, transmissionPermalink: https://doi.org/10.1190/image2022-3745792.1FiguresReferencesRelatedDetails Second International Meeting for Applied Geoscience & EnergyISSN (print):1052-3812 ISSN (online):1949-4645Copyright: 2022 Pages: 3694 publication data© 2022 Published in electronic format with permission by the Society of Exploration Geophysicists and the American Association of Petroleum GeologistsPublisher:Society of Exploration Geophysicists HistoryPublished Online: 15 Aug 2022 CITATION INFORMATION Lei Fu, Daniele Colombo, Weichang Li, Ernesto Sandoval-Curiel, and Ersan Turkoglu, (2022), "Deep learning Laplace-Fourier full-waveform inversion with virtual supershot gathers," SEG Technical Program Expanded Abstracts : 1940-1944. https://doi.org/10.1190/image2022-3745792.1 Plain-Language Summary Keywordsmachine learningdeep learningfull-waveform inversionnear surfacetransmissionPDF DownloadLoading ...
MoreTranslated text
Key words
deep learning,inversion,laplace-fourier,full-waveform
AI Read Science
Must-Reading Tree
Example
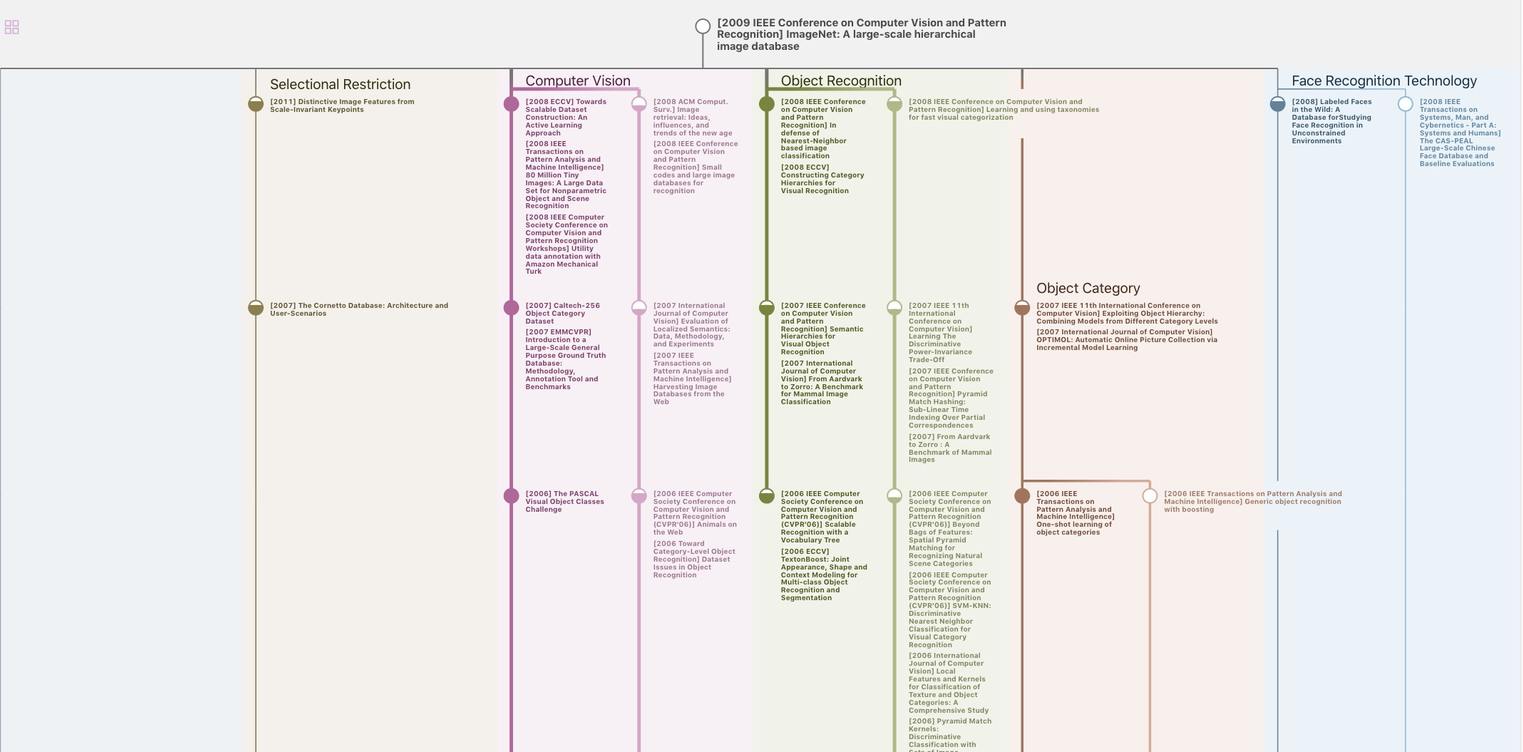
Generate MRT to find the research sequence of this paper
Chat Paper
Summary is being generated by the instructions you defined