Dual Regression for Efficient Hand Pose Estimation
2022 International Conference on Robotics and Automation (ICRA)(2022)
摘要
Hand pose estimation constitutes prime attainment for human-machine interaction-based applications. Real-time operation is vital in such tasks. Thus, a reliable estimator should exhibit low computational complexity and high precision at the same time. Previous works have explored the regression techniques, including the coordinate regression and heatmap regression methods. Primarily incorporating ideas from them, in this paper, we propose a novel, fast and accurate method for hand pose estimation, which adopts a lightweight network architecture and a post-processing scheme. Hence, our architecture uses a Dual Regression strategy, consisting of two regression branches, namely the coordinate and the heatmap ones, and we refer to the proposed method as DRHand. By carefully selecting the branches' characteristics, the proposed structure has been designed to exploit the benefits of the two methods mentioned above while impoverishing their weaknesses to some extent. The two branches are supervised separately during training, and a post-processing module estimates their outputs to boost reliability. This way, our novel pipeline is considerably faster, reaching 44.39 frames-per-second on an NVIDIA Jetson TX2 graphics processing unit, offering a beyond real-time performance for any custom robotics application. Lastly, extensive experiments conducted on two publicly-available datasets demonstrate that the proposed framework outperforms previous state-of-the-art techniques and can generalize on various hand pose scenarios.
更多查看译文
关键词
efficient hand pose estimation,dual regression
AI 理解论文
溯源树
样例
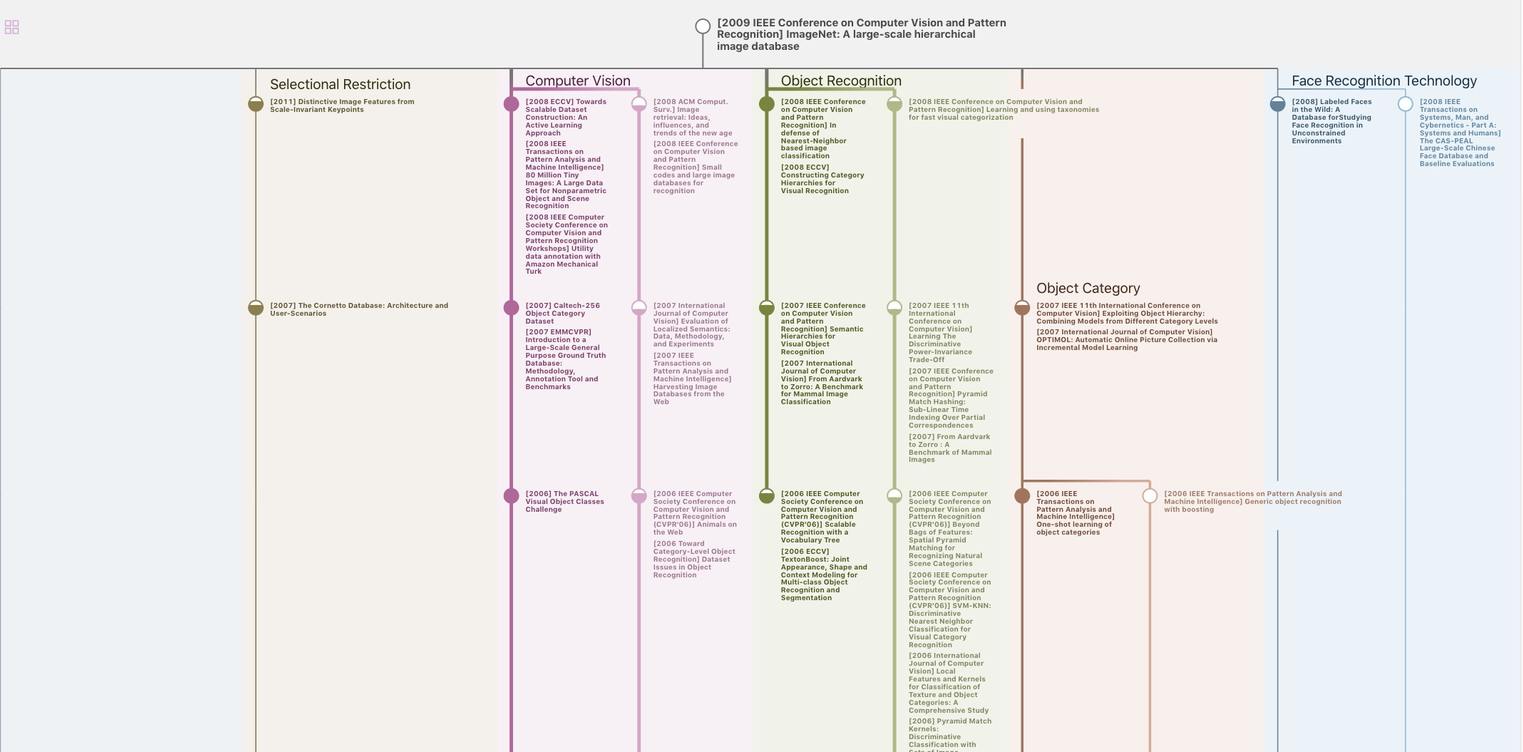
生成溯源树,研究论文发展脉络
Chat Paper
正在生成论文摘要