The Robust Multi-Scale Deep-SVDD Model for Anomaly Online Detection of Rolling Bearings
SENSORS(2022)
摘要
Aiming at the online detection problem of rolling bearings, the limited amount of target bearing data leads to insufficient model in training and feature representation. It is difficult for the online detection model to construct an accurate decision boundary. To solve the problem, a multi-scale robust anomaly detection method based on data enhancement technology is proposed in this paper. Firstly, the training data are transformed into multiple subspaces through the data enhancement technology. Then, a prototype clustering method is introduced to enhance the robustness of features representation under the framework of the robust deep auto-encoding algorithm. Finally, the robust multi-scale Deep-SVDD hyper sphere model is constructed to achieve online detection of abnormal state data. Experiments are conducted on the IEEE PHM Challenge 2012 bearing data set and XJTU-TU data set. The proposed method shows much greater susceptibility to incipient faults, and it has fewer false alarms. The robust multi-scale Deep-SVDD hyper sphere model significantly improves the performance of incipient fault detection for rolling bearings.
更多查看译文
关键词
incipient fault detection, robustness, reinforcement learning, anomaly detection
AI 理解论文
溯源树
样例
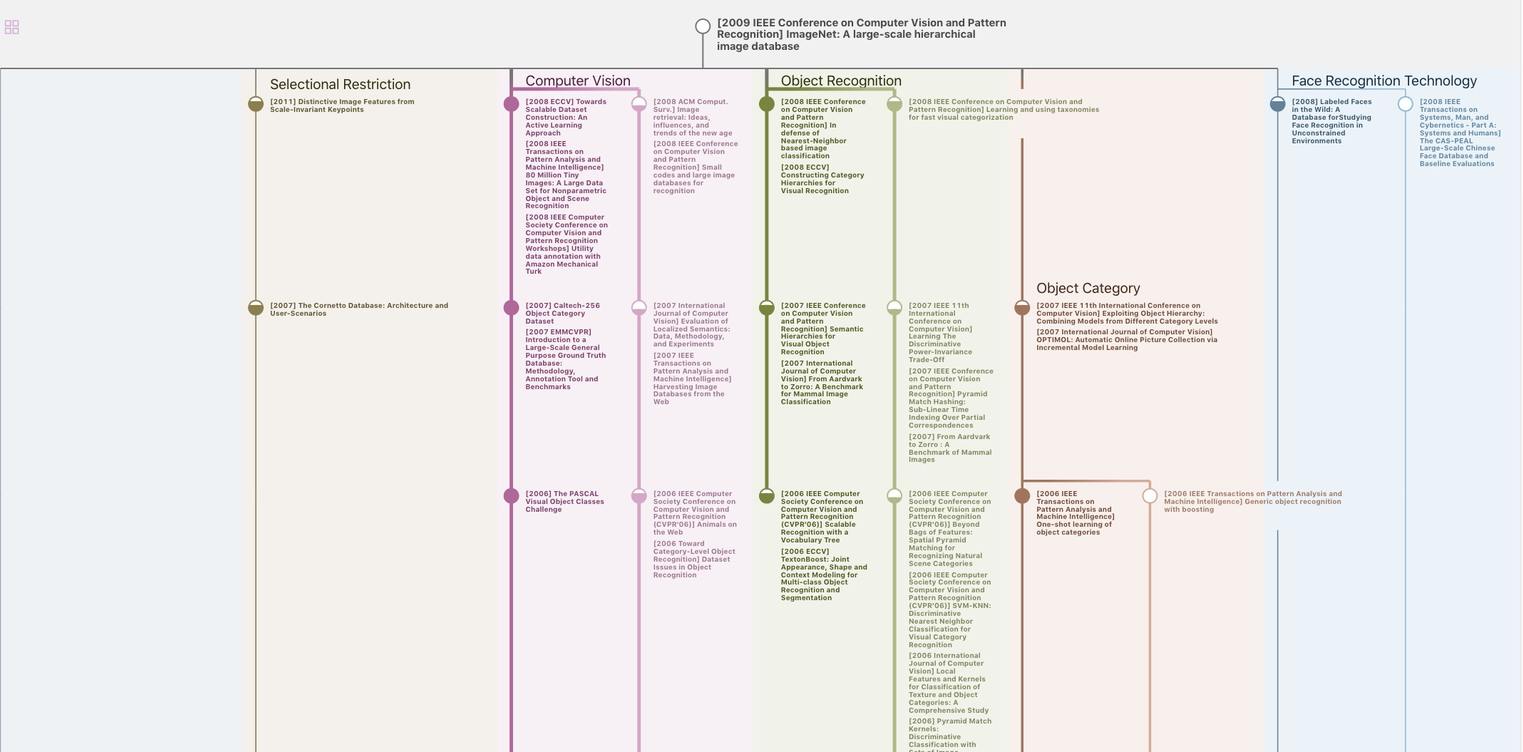
生成溯源树,研究论文发展脉络
Chat Paper
正在生成论文摘要