Accelerating the Layup Sequences Design of Composite Laminates via Theory-Guided Machine Learning Models
POLYMERS(2022)
摘要
Experimental and numerical investigations are presented for a theory-guided machine learning (ML) model that combines the Hashin failure theory (HFT) and the classical lamination theory (CLT) to optimize and accelerate the design of composite laminates. A finite element simulation with the incorporation of the HFT and CLT were used to generate the training dataset. Instead of directly mapping the relationship between the ply angles of the laminate and its strength and stiffness, a multi-layer interconnected neural network (NN) system was built following the logical sequence of composite theories. With the forward prediction by the NN system and the inverse optimization by genetic algorithm (GA), a benchmark case of designing a composite tube subjected to the combined loads of bending and torsion was studied. The ML models successfully provided the optimal layup sequences and the required fiber modulus according to the preset design targets. Additionally, it shows that the machine learning models, with the guidance of composite theories, realize a faster optimization process and requires less training data than models with direct simple NNs. Such results imply the importance of domain knowledge in helping improve the ML applications in engineering problems.
更多查看译文
关键词
composite laminate, mechanical property, layup design, finite element simulation, neural network
AI 理解论文
溯源树
样例
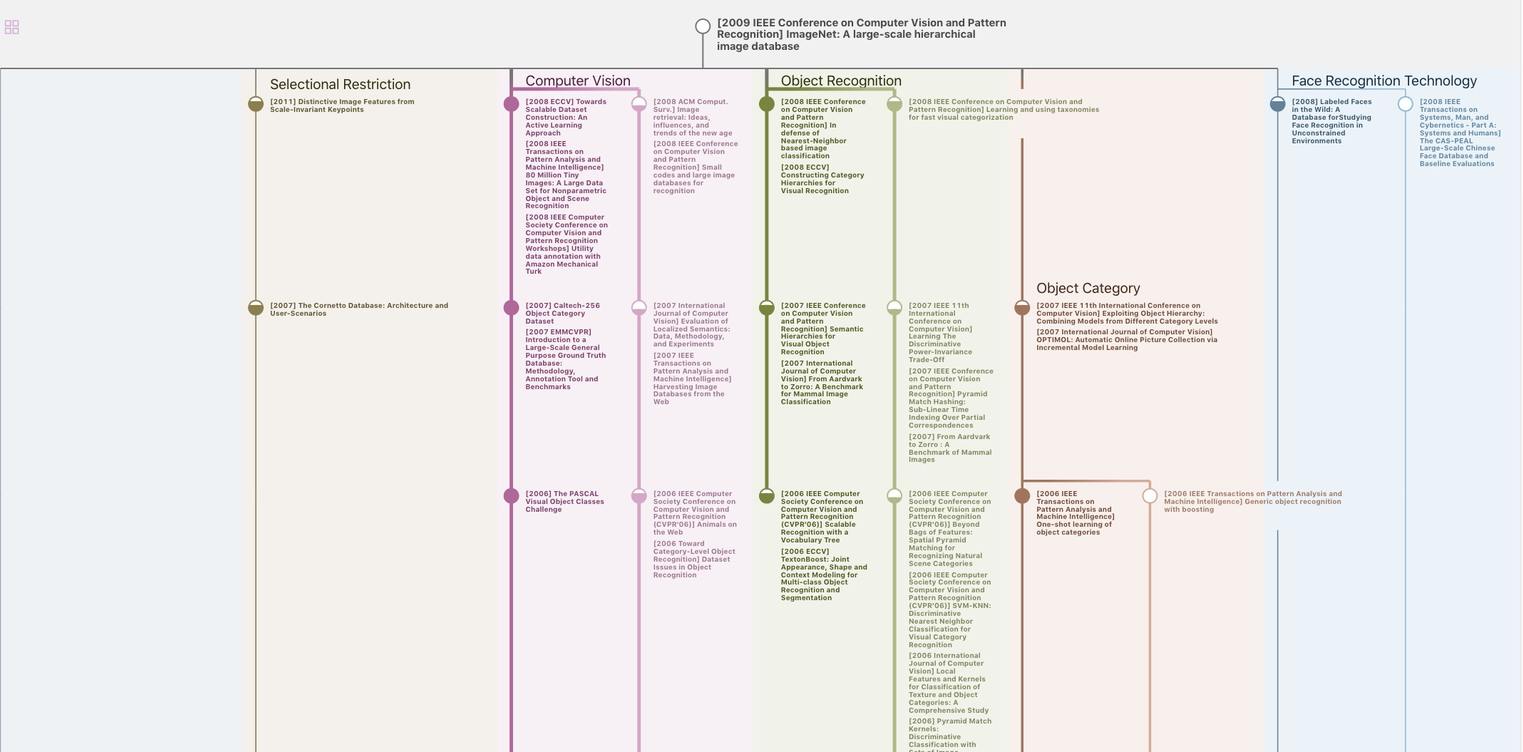
生成溯源树,研究论文发展脉络
Chat Paper
正在生成论文摘要