Action Recognition based on Cross-Situational Action-object Statistics
2022 IEEE International Conference on Development and Learning (ICDL)(2022)
摘要
Machine learning models of visual action recognition are typically trained and tested on data from specific situations where actions are associated with certain objects. It is an open question how action-object associations in the training set influence a model’s ability to generalize beyond trained situations. We set out to identify properties of training data that lead to action recognition models with greater generalization ability. To do this, we take inspiration from a cognitive mechanism called cross-situational learning, which states that human learners extract the meaning of concepts by observing instances of the same concept across different situations. We perform controlled experiments with various types of action-object associations, and identify key properties of action-object co-occurrence in training data that lead to better classifiers. Given that these properties are missing in the datasets that are typically used to train action classifiers in the computer vision literature, our work provides useful insights on how we should best construct datasets for efficiently training for better generalization.
更多查看译文
关键词
recognition,statistics,cross-situational,action-object
AI 理解论文
溯源树
样例
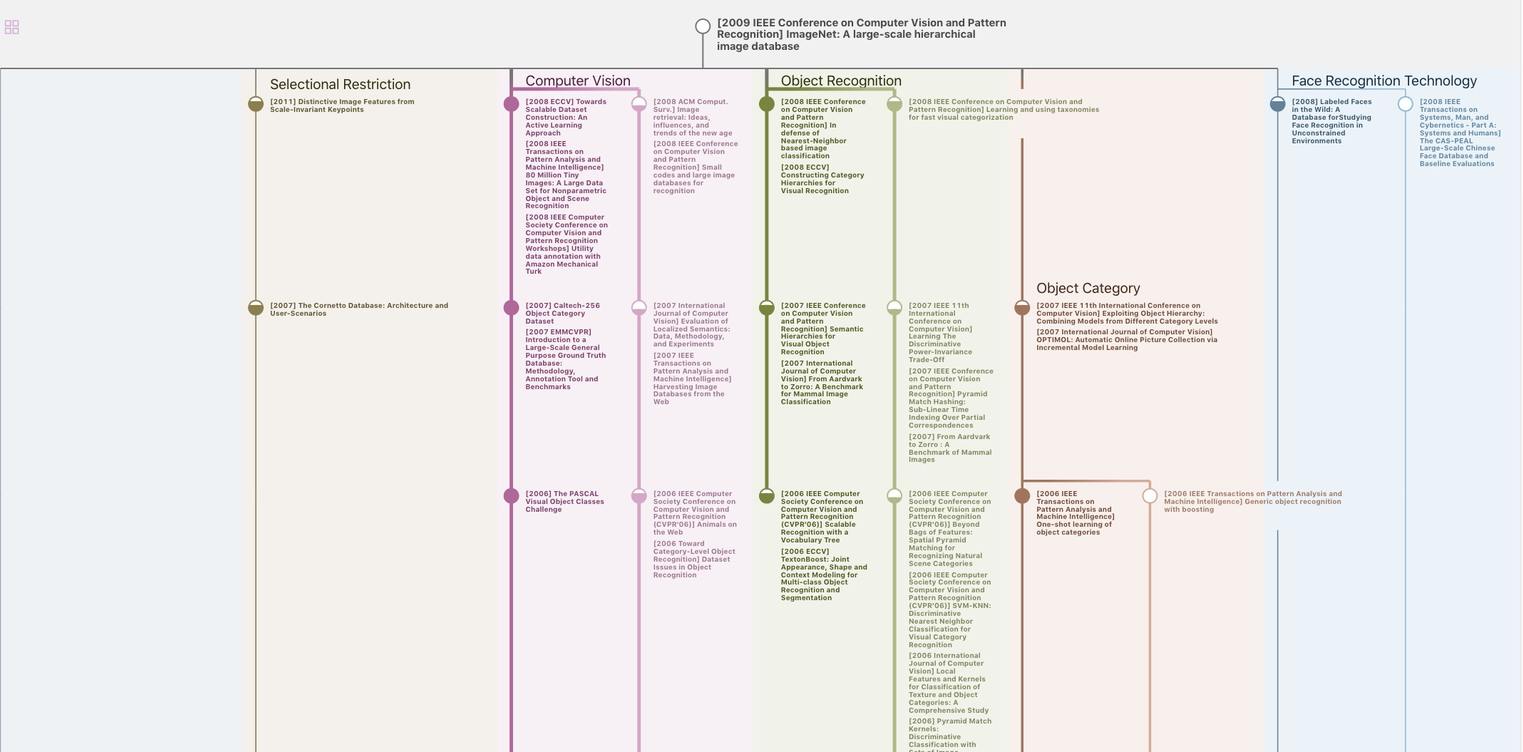
生成溯源树,研究论文发展脉络
Chat Paper
正在生成论文摘要