Energy and Spectrum Efficient Federated Learning via High-Precision Over-the-Air Computation
IEEE TRANSACTIONS ON WIRELESS COMMUNICATIONS(2024)
摘要
Federated learning (FL) enables mobile devices to collaboratively learn a shared prediction model while keeping data locally. However, there are two major research challenges to practically deploy FL over mobile devices: (i) frequent wireless updates of huge size gradients v.s. limited spectrum resources, and (ii) energy-hungry FL communication and local computing during training v.s. battery-constrained mobile devices. To address those challenges, in this paper, we propose a novel multi-bit over-the-air computation (M-AirComp) approach for spectrum-efficient aggregation of local model updates in FL and further present an energy-efficient FL design for mobile devices. Specifically, a high-precision digital modulation scheme is designed and incorporated in the M-AirComp, allowing mobile devices to upload model updates at the selected positions simultaneously in the multi-access channel. Moreover, we theoretically analyze the convergence property of our FL algorithm. Guided by FL convergence analysis, we formulate a joint transmission probability and local computing control optimization, aiming to minimize the overall energy consumption (i.e., iterative local computing + multi-round communications) of mobile devices in FL. Extensive simulation results show that our proposed scheme outperforms existing ones in terms of spectrum utilization, energy efficiency, and learning accuracy.
更多查看译文
关键词
Federated learning,over-the-air computation,gradient quantization,energy efficiency
AI 理解论文
溯源树
样例
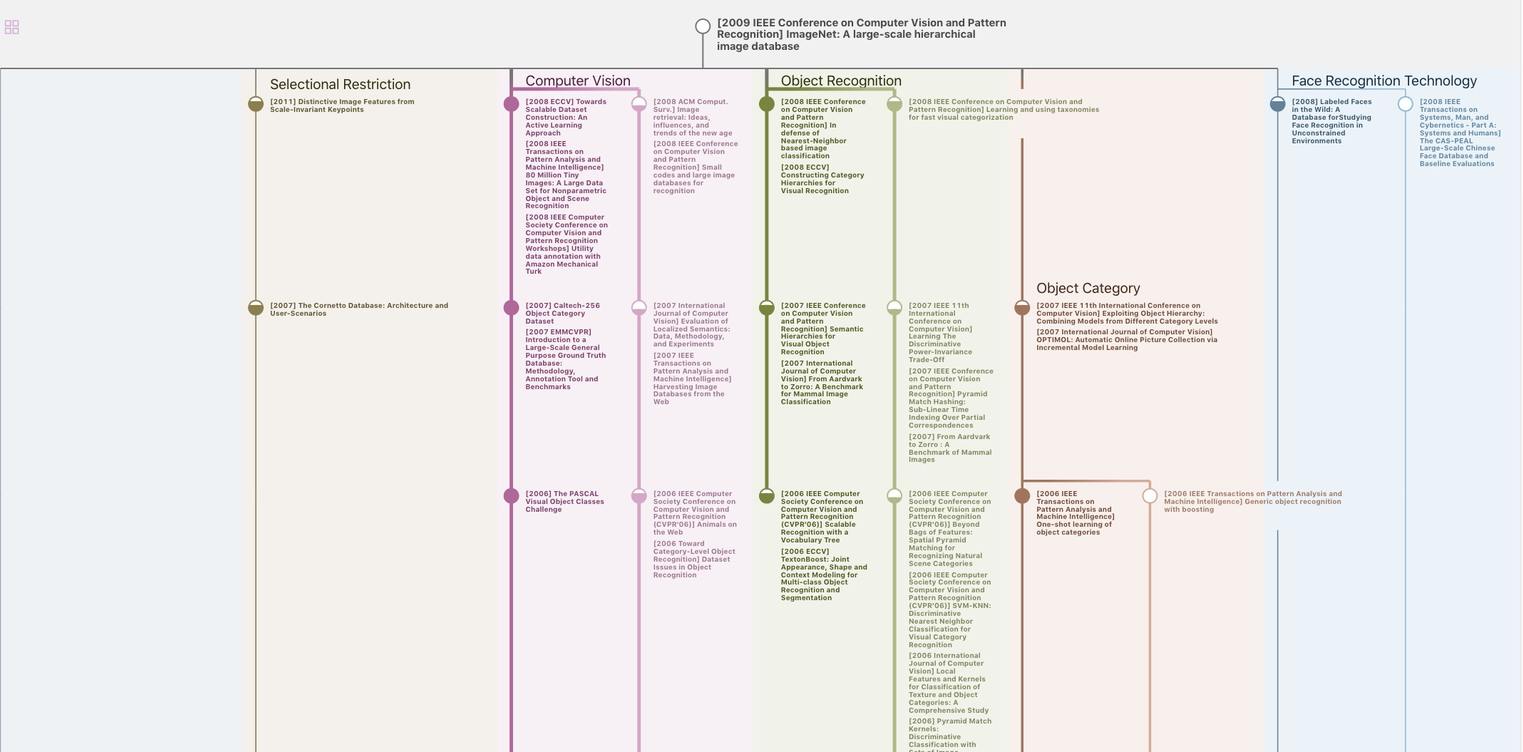
生成溯源树,研究论文发展脉络
Chat Paper
正在生成论文摘要