AMinerGNN: Heterogeneous Graph Neural Network for Paper Click-through Rate Prediction with Fusion Query
Proceedings of the 31st ACM International Conference on Information & Knowledge Management(2022)
Abstract
Paper recommendation with user-generated keyword is to suggest papers that simultaneously meet user's interests and are relevant to the input keyword. This is a recommendation task with two queries, a.k.a. user ID and keyword. However, existing methods focus on recommendation according to one query, a.k.a. user ID, and are not applicable to solving this problem. In this paper, we propose a novel click-through rate (CTR) prediction model with heterogeneous graph neural network, called AMinerGNN, to recommend papers with two queries. Specifically, AMinerGNN constructs a heterogeneous graph to project user, paper, and keyword into the same embedding space by graph representation learning. To process two queries, a novel query attentive fusion layer is designed to recognize their importances dynamically and then fuse them as one query to build a unified and end-to-end recommender system. Experimental results on our proposed dataset and online A/B tests prove the superiority of AMinerGNN.
MoreTranslated text
Key words
Click-Through Rate Prediction,Graph Neural Network,Recommender System
AI Read Science
Must-Reading Tree
Example
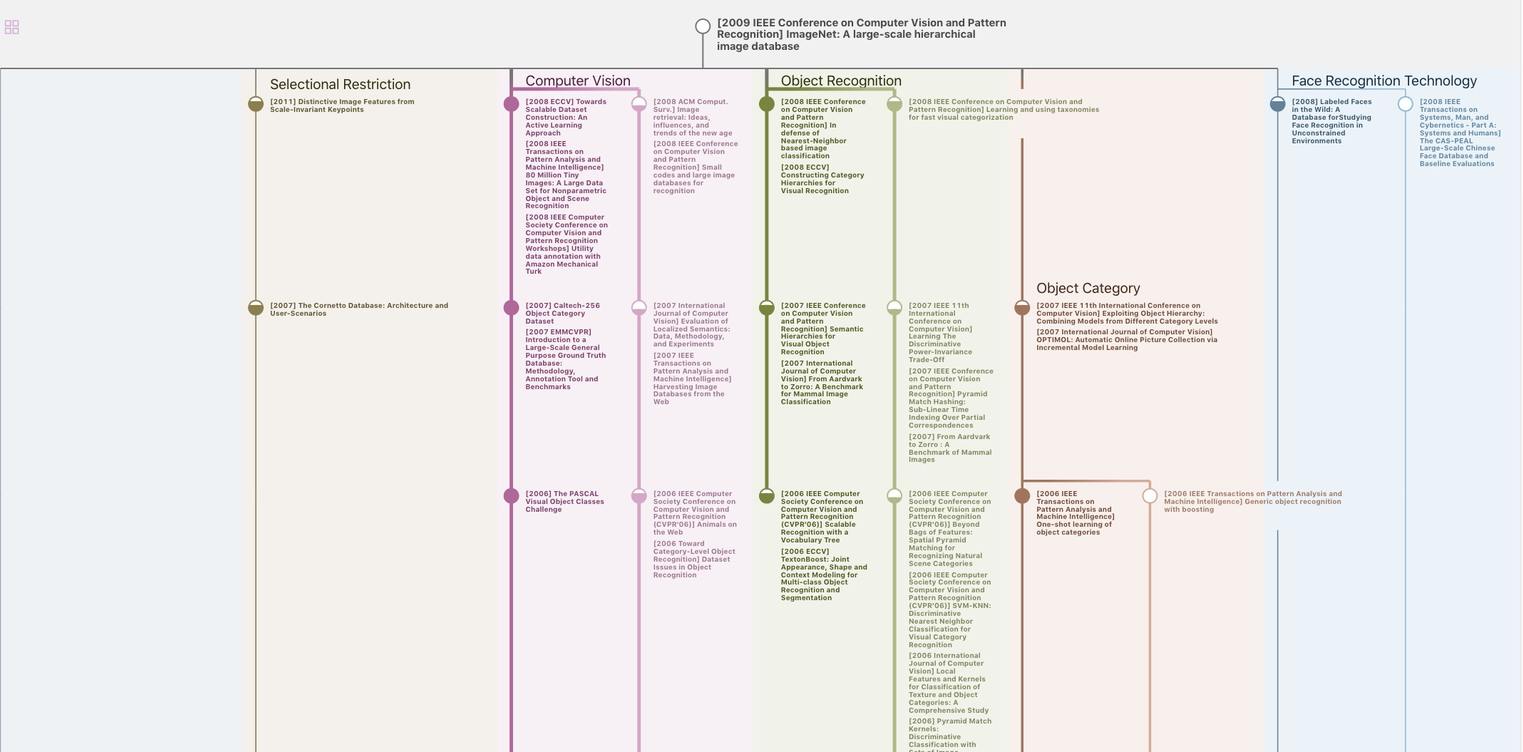
Generate MRT to find the research sequence of this paper
Chat Paper
Summary is being generated by the instructions you defined