A Configurable Spiking Convolution Architecture Supporting Multiple Coding Schemes on FPGA
IEEE Transactions on Circuits and Systems II: Express Briefs(2022)
Abstract
In this brief, an event-driven spiking convolution architecture with multi-kernel and multi-layer capability is designed. The proposed architecture can be configured in multiple-spike (MS) mode or single-spike (SS) mode to adapt to different spiking convolution neural network (SCNN) models with either rate coding scheme or temporal coding scheme. A skipped zero kernel step is designed to reduce access neuron membrane potentials in memories. In addition, the proposed design supports two pooling methods for increasing flexibility. The proposed architecture is implemented in a Xilinx Kintex-7 FPGA development board, and two SCNN models with different coding schemes are applied to verify the efficiency of the architecture. For the first SCNN model with rate coding scheme, the proposed architecture achieves a 99 % classification accuracy with 0.46 mJ/classification. For the second SCNN model with temporal coding scheme, the proposed architecture obtains a classification accuracy over 95.4 % with 7.4 uJ/classification. Experimental results demonstrate that the proposed design is adaptable and has a low power consumption overhead.
MoreTranslated text
Key words
Spiking convolutional neural network (SCNN),image classification,configurable network,field programmable gate array (FPGA),event-driven
AI Read Science
Must-Reading Tree
Example
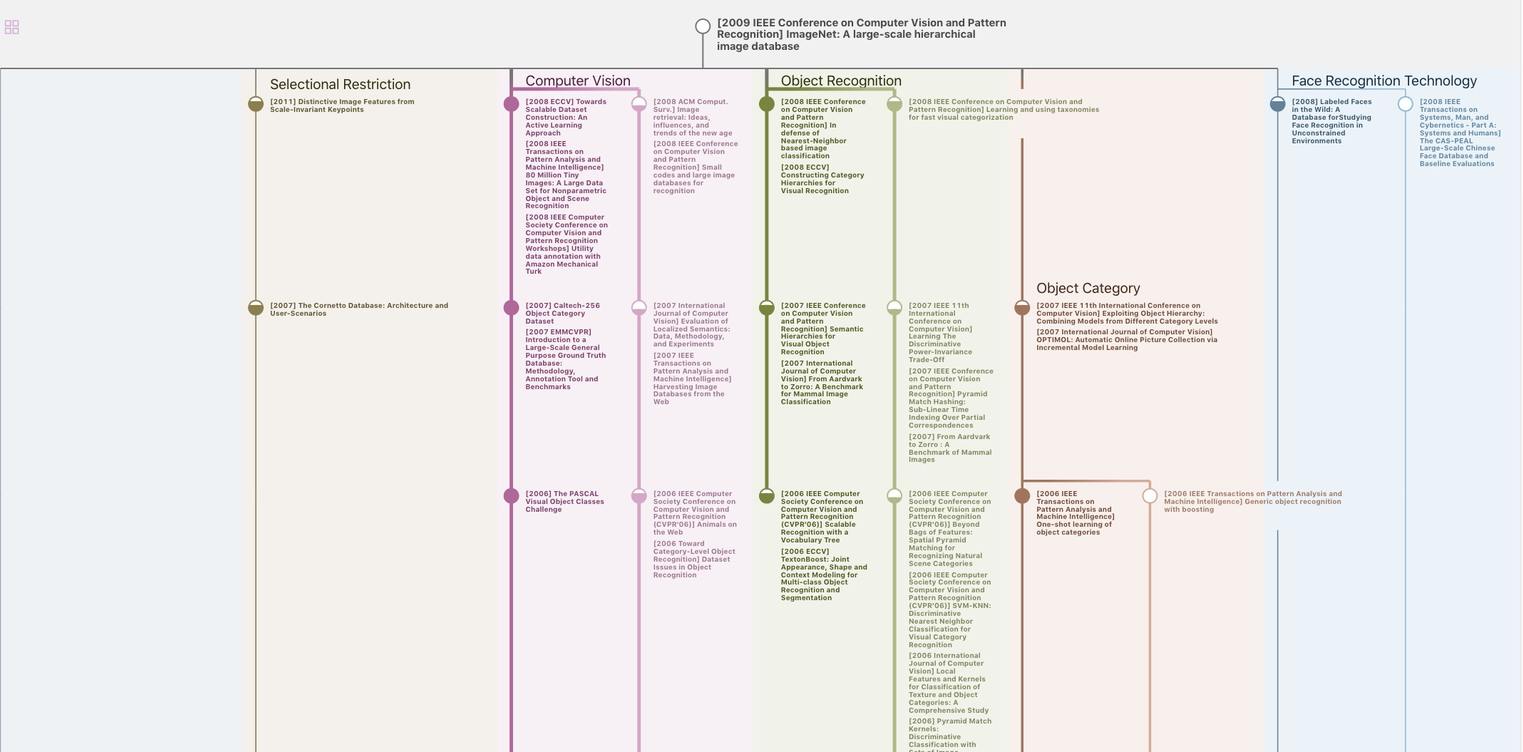
Generate MRT to find the research sequence of this paper
Chat Paper
Summary is being generated by the instructions you defined