Multivariate Time Series Prediction in Industrial Processes via a Deep Hybrid Network under Data Uncertainty
IEEE Transactions on Industrial Informatics(2022)
摘要
With the rapid progress of the industrial Internet of Things (IIoT), reducing data uncertainty has become a critical issue in predicting the development trends of systems and formulating future maintenance strategies. This article proposes an end-to-end, deep hybrid network-based, short-term, multivariate time-series prediction framework for industrial processes. First, the maximal information coefficient is adopted to extract the nonlinear variate correlation features. Second, a convolutional neural network with a residual elimination module is designed to eliminate data uncertainty. Third, a bidirectional gated recurrent unit network is connected in a time-distributed form to achieve step-ahead prediction. Last, an optimized Bayesian optimization method is adopted to optimize the model's learning rate. A comparison with other state-of-the-art, deep learning-based, time-series prediction methods in the case study illustrates the superiority of the proposed framework in noisy IIoT environments.
更多查看译文
关键词
Data uncertainty,deep hybrid networks,hyperparameter optimization,industrial Internet of Things (IIoT),multivariate time-series prediction
AI 理解论文
溯源树
样例
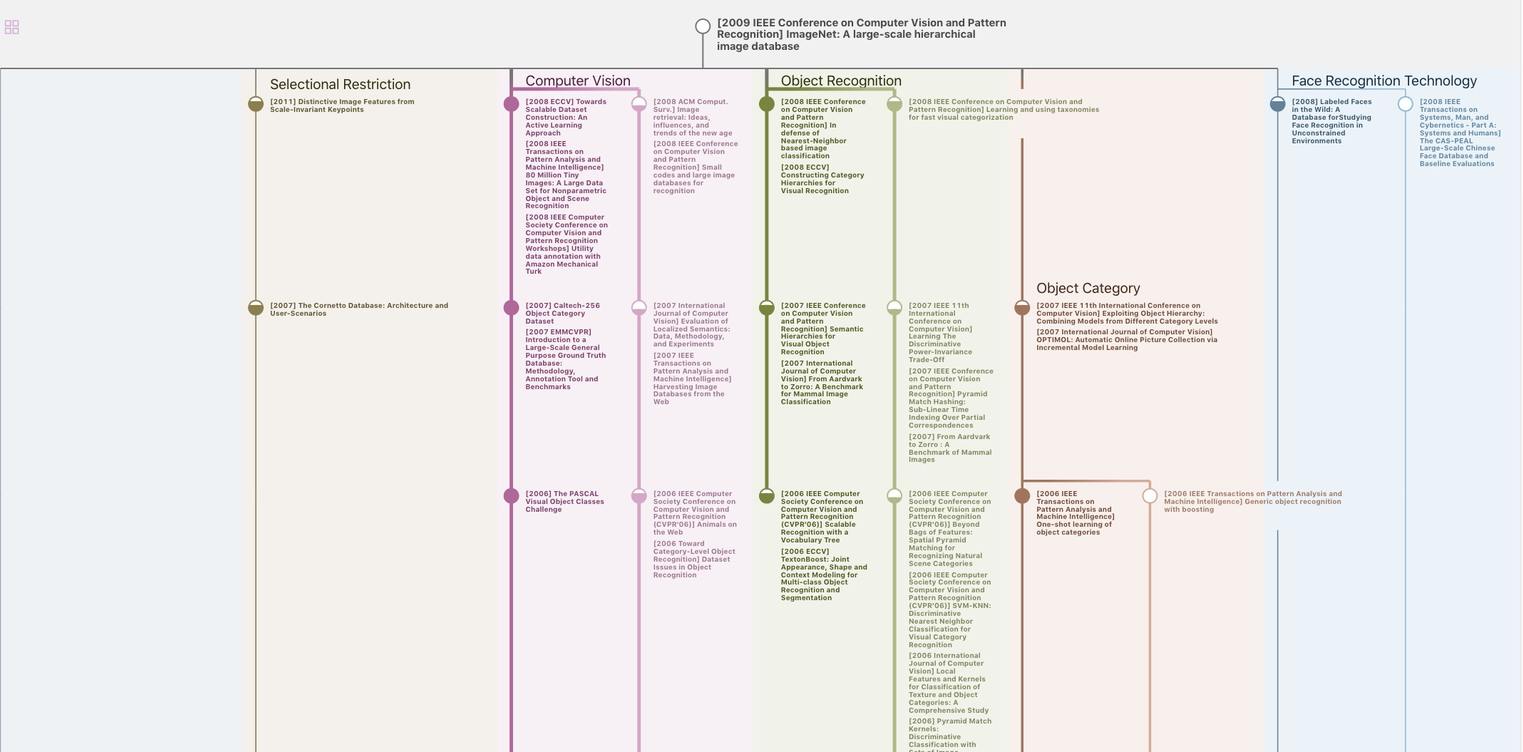
生成溯源树,研究论文发展脉络
Chat Paper
正在生成论文摘要