RenyiCL: Contrastive Representation Learning with Skew Renyi Divergence
arxiv(2023)
摘要
Contrastive representation learning seeks to acquire useful representations by estimating the shared information between multiple views of data. Here, the choice of data augmentation is sensitive to the quality of learned representations: as harder the data augmentations are applied, the views share more task-relevant information, but also task-irrelevant one that can hinder the generalization capability of representation. Motivated by this, we present a new robust contrastive learning scheme, coined R\'enyiCL, which can effectively manage harder augmentations by utilizing R\'enyi divergence. Our method is built upon the variational lower bound of R\'enyi divergence, but a na\"ive usage of a variational method is impractical due to the large variance. To tackle this challenge, we propose a novel contrastive objective that conducts variational estimation of a skew R\'enyi divergence and provide a theoretical guarantee on how variational estimation of skew divergence leads to stable training. We show that R\'enyi contrastive learning objectives perform innate hard negative sampling and easy positive sampling simultaneously so that it can selectively learn useful features and ignore nuisance features. Through experiments on ImageNet, we show that R\'enyi contrastive learning with stronger augmentations outperforms other self-supervised methods without extra regularization or computational overhead. Moreover, we also validate our method on other domains such as graph and tabular, showing empirical gain over other contrastive methods.
更多查看译文
关键词
Contrastive learning,Self-supervised learning,Representation learning,Data augmentation
AI 理解论文
溯源树
样例
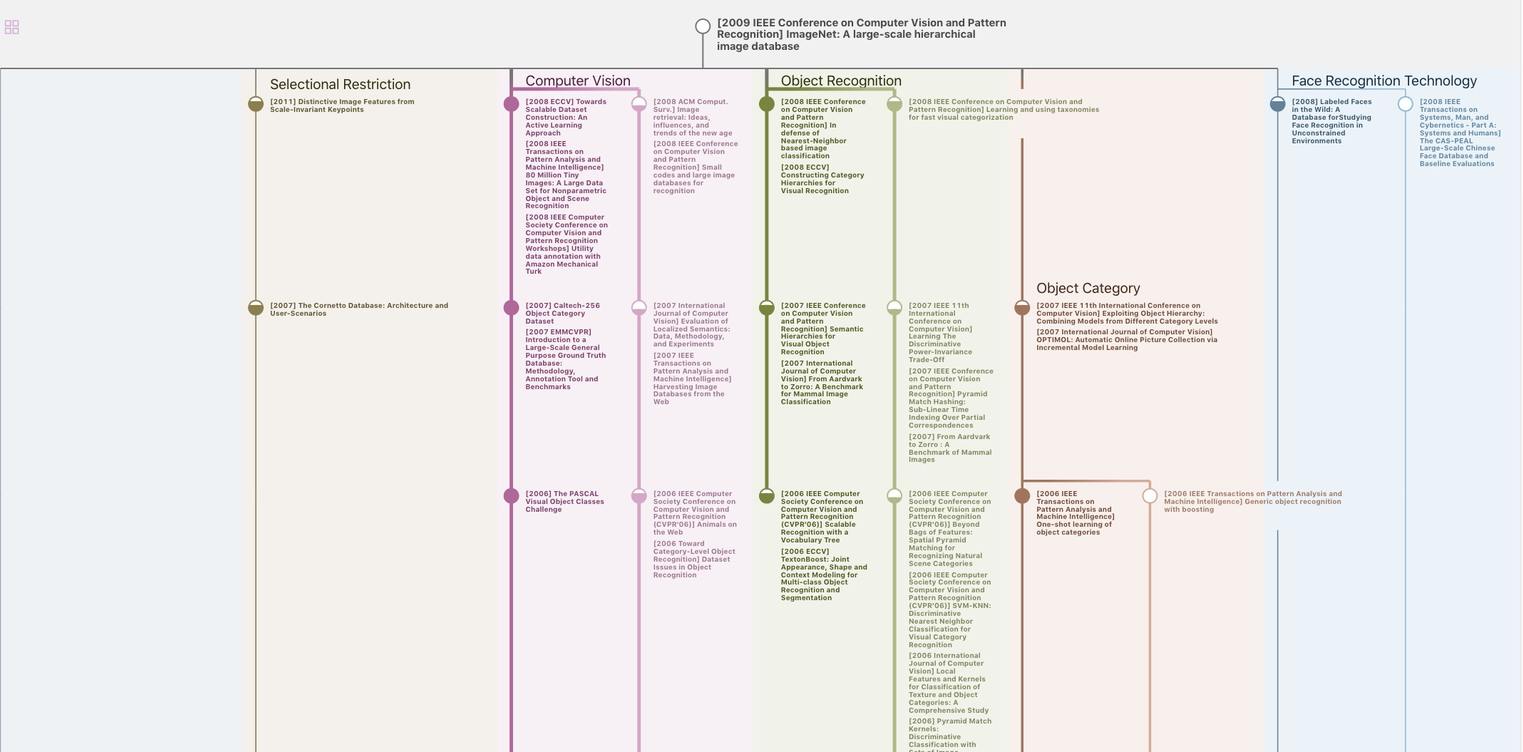
生成溯源树,研究论文发展脉络
Chat Paper
正在生成论文摘要