Conditional Antibody Design as 3D Equivariant Graph Translation.
ICLR 2023(2023)
摘要
Antibody design is valuable for therapeutic usage and biological research. Existing deep-learning-based methods encounter several key issues: 1) incomplete context for Complementarity-Determining Regions (CDRs) generation; 2) incapable of capturing the entire 3D geometry of the input structure; 3) inefficient prediction of the CDR sequences in an autoregressive manner. In this paper, we propose Multi-channel Equivariant Attention Network (MEAN), an end-to-end model that is able to co-design 1D sequences and 3D structures of CDRs. To be specific, MEAN formulates antibody design as a conditional graph translation problem by importing extra components including the target antigen and the light chain of the antibody. Then, MEAN resorts to E(3)-equivariant message passing along with a proposed attention mechanism to better capture the geometrical correlation between different components. Finally, it outputs both the 1D sequences and 3D structure via a multi-round progressive full-shot scheme, which enjoys more efficiency against previous autoregressive approaches. Our method significantly surpasses state-of-the-art models in sequence and structure modeling, antigen-binding antibody design, and binding affinity optimization. Specifically, the relative improvement to baselines is about 23% in antigen-binding CDR design and 34% for affinity optimization.
更多查看译文
关键词
conditional antibody generation,equivariant,multi-channel attention
AI 理解论文
溯源树
样例
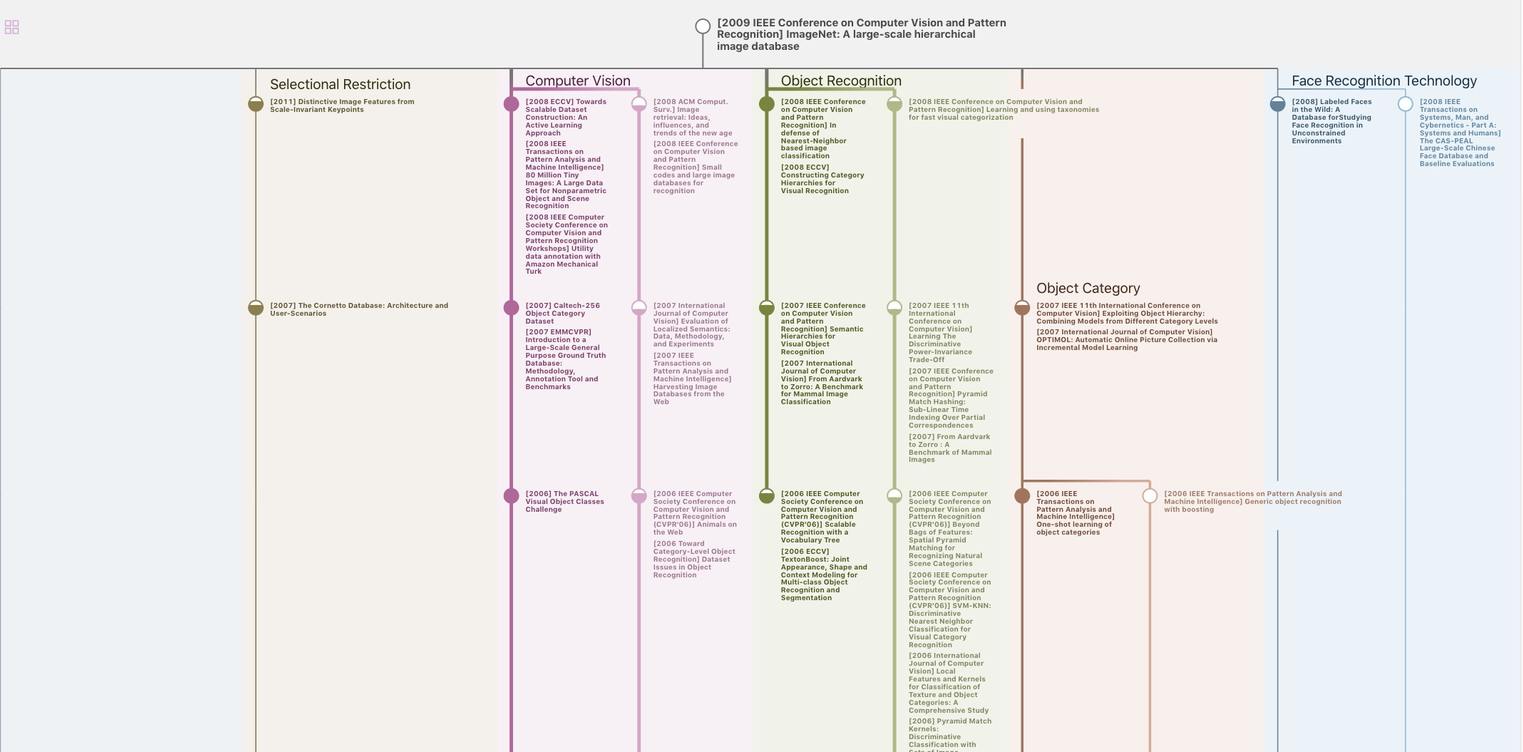
生成溯源树,研究论文发展脉络
Chat Paper
正在生成论文摘要