Analysing Supercomputer Nodes Behaviour with the Latent Representation of Deep Learning Models
EURO-PAR 2022: PARALLEL PROCESSING(2022)
摘要
Anomaly detection systems are vital in ensuring the availability of modern High-Performance Computing (HPC) systems, where many components can fail or behave wrongly. Building a data-driven representation of the computing nodes can help with predictive maintenance and facility management. Luckily, most of the current supercomputers are endowed with monitoring frameworks that can build such representations in conjunction with Deep Learning (DL) models. In this work, we propose a novel semi-supervised DL approach based on autoencoder networks and clustering algorithms (applied to the latent representation) to build a digital twin of the computing nodes of the system. The DL model projects the node features into a lower-dimensional space. Then, clustering is applied to capture and reveal underlying, non-trivial correlations between the features. The extracted information provides valuable insights for system administrators and managers, such as anomaly detection and node classification based on their behaviour and operative conditions. We validated the approach on 240 nodes from the Marconi 100 system, a Tier-0 supercomputer located in CINECA (Italy), considering a 10-month period.
更多查看译文
关键词
supercomputer monitoring, deep Learning, unsupervised learning, autoencoders, predictive maintenance
AI 理解论文
溯源树
样例
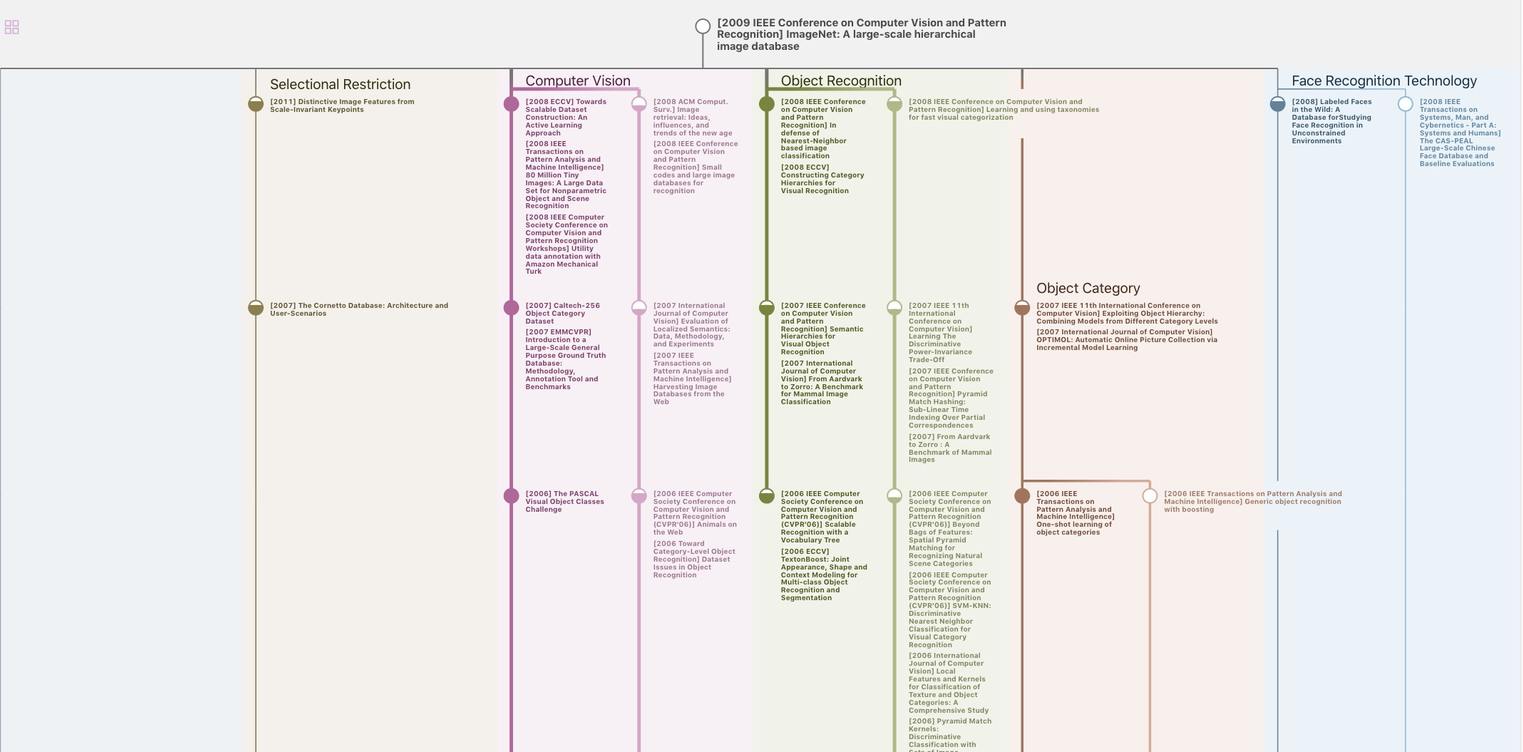
生成溯源树,研究论文发展脉络
Chat Paper
正在生成论文摘要