MANomaly: Mutual adversarial networks for semi-supervised anomaly detection
Information Sciences(2022)
摘要
In network intrusion detection, since the available attack traffic is much less than normal traffic, detecting attacks and intrusions from these unbalanced traffic can be a problem of semi-supervised learning, i.e., finding outliers (anomalies) from a data population that obeys a certain distribution. In this paper, we design a novel network model named the mutual adversarial network (MAN), which has two identical reconstruction autoencoder (RecAE) subnetworks. In training, these two subnetworks use the proposed mutual adversarial training to learn the data distribution of normal traffic samples. In detection, we identify anomalies based on the residual values obtained after different samples are reconstructed by MAN. In addition, we devise a novel method to identify anomalies from anomaly scores named the high anomaly suppression (HAS) determination mechanism, which uses the mean values to suppress the effect of noisy data in the test sample. Then, we construct a novel semi-supervised reconstruction anomaly detection framework named MANomaly by combining MAN with the HAS determination mechanism. Meanwhile, we design three different mutual adversarial training approaches to MANomaly and evaluate them on two publicly available network traffic datasets: NSL-KDD and UNSW-NB15. Experimental results show that our method achieves excellent performance by using only 5% of normal training data.
更多查看译文
关键词
Network intrusion detection,Anomaly detection,Mutual adversarial network,Mutual adversarial training,High anomaly suppression
AI 理解论文
溯源树
样例
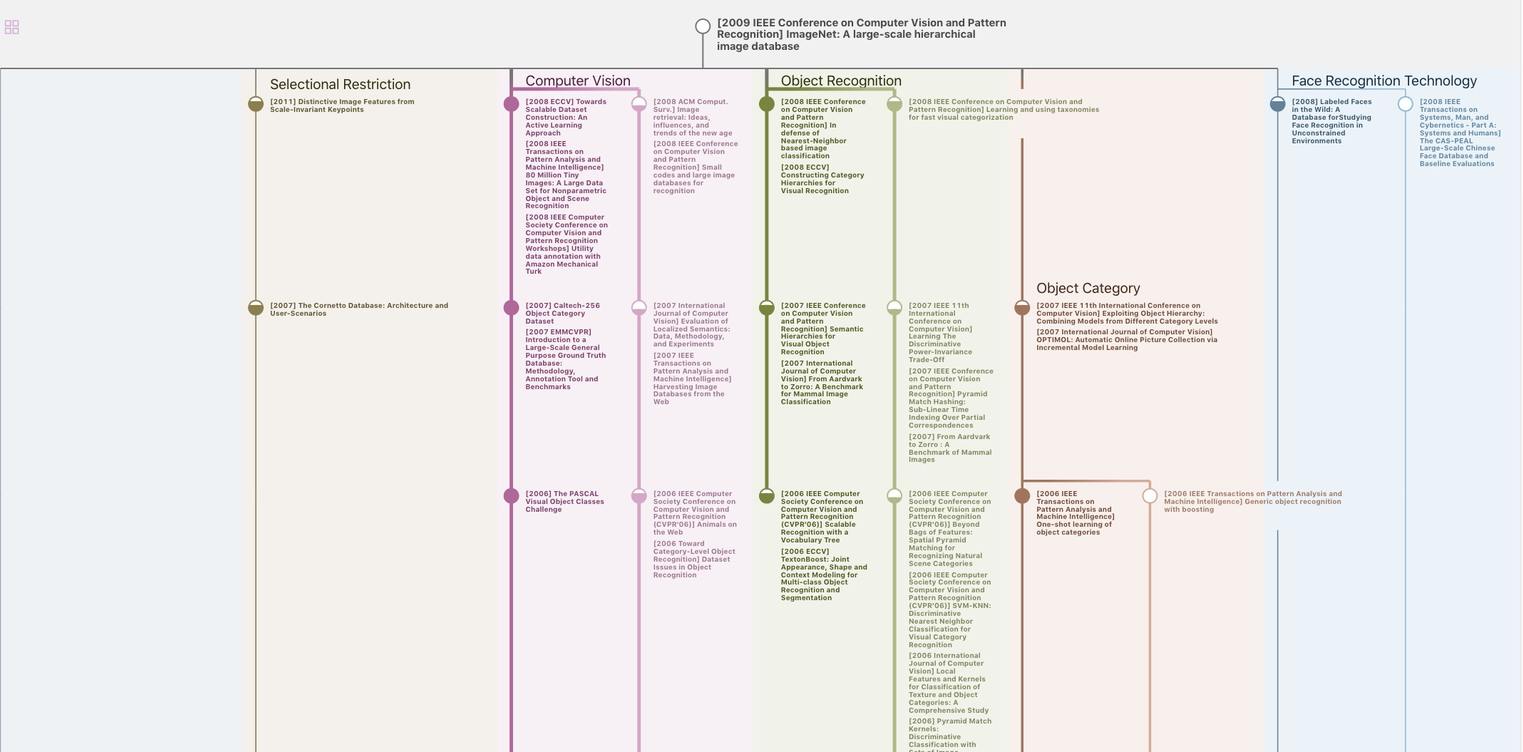
生成溯源树,研究论文发展脉络
Chat Paper
正在生成论文摘要