Reinforcement learning-based optimal operation of ash deposit removal system to improve recycling efficiency of biomass for CO2 reduction
Journal of Cleaner Production(2022)
摘要
Black liquor from pulp mills is valuable biomass that can be recycled as a CO2-neutral, renewable fuel. However, biomass combustion produces significant ash deposits reducing the overall process efficiency. A recovery boiler generally uses an ash deposit removal system (ADRS), but ADRS operation is inefficient, and the recycling efficiency of the biomass is decreased, leading to an increase in CO2 emission. This work proposed an optimal operation of ADRS to improve the recycling efficiency of biomass for CO2 emission reduction based on reinforcement learning. The optimal operation of the ADRS was derived by the following steps. 1) Real-time process operating data (i.e., temperatures of the flue gas, water, and steam) were gathered and a computational fluid dynamics model was developed to predict the flue gas temperature in the superheater section. 2) The decrease in the heat transfer rate was calculated using the gathered data to define a reward update matrix. 3) A modified Q-learning algorithm was developed based on the defined reward update matrix, and the algorithm was used to derive the Q-matrix, a function that predicted the expected dynamic reward (i.e., priority for ash deposit removal) of performing a given action (i.e., sootblowing) at a given state (i.e., each sootblowing location). 4) Using the obtained Q-matrix, the optimal operating sequence was derived. As a result, 22.58 ton/d of black liquor was saved and the CO2 emission decreased by 755–1390 ton/y with an increase in the net profit by $1,010,000.
更多查看译文
关键词
Recovery boiler,Ash deposits,Biomass,CO2 neutral,Reinforcement learning
AI 理解论文
溯源树
样例
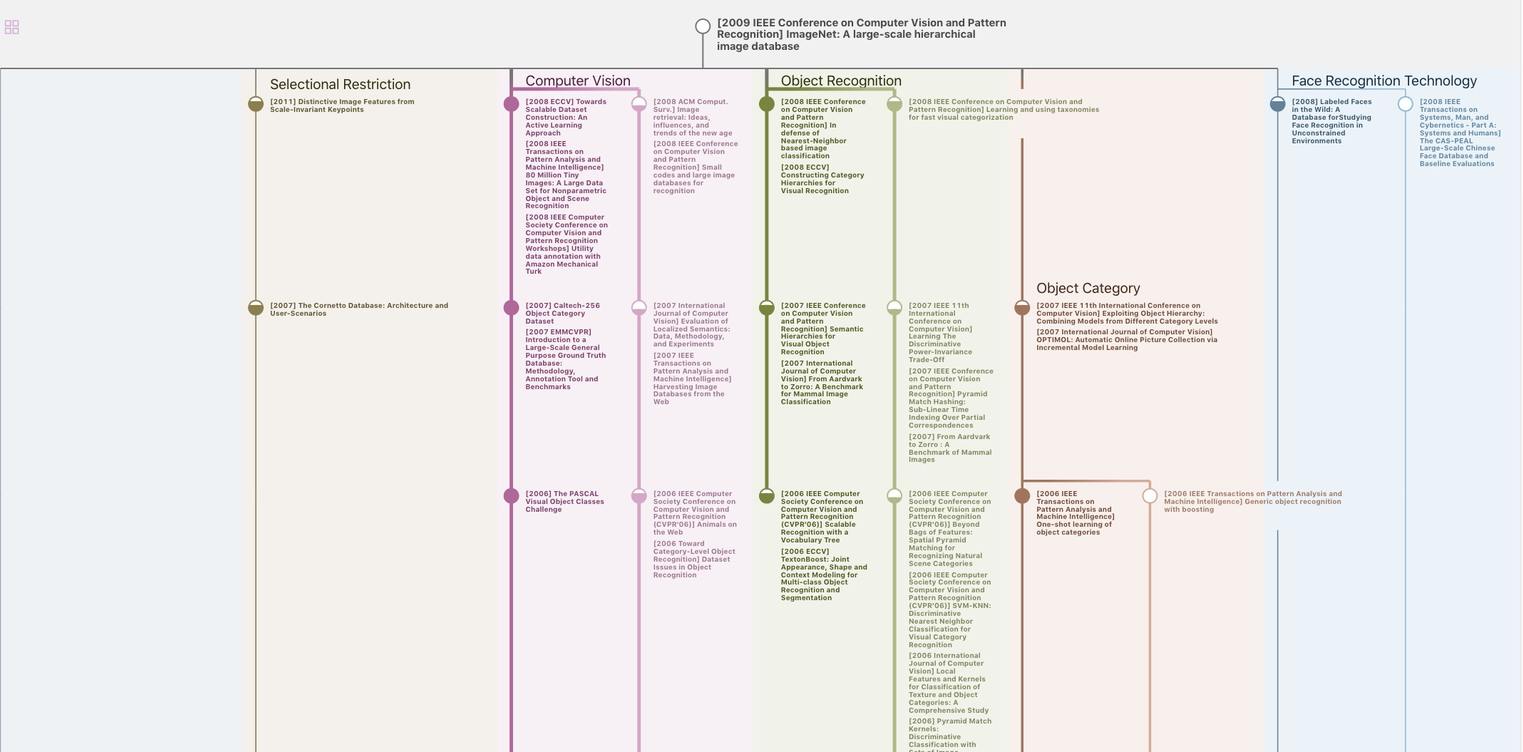
生成溯源树,研究论文发展脉络
Chat Paper
正在生成论文摘要