DeepLabCut increases markerless tracking efficiency in X-ray video analysis of rodent locomotion
JOURNAL OF EXPERIMENTAL BIOLOGY(2022)
摘要
Despite the prevalence of rat models to study human disease and injury, existing methods for quantifying behavior through skeletal movements are problematic owing to skin movement inaccuracies associated with optical video analysis, or require invasive implanted markers or time-consuming manual rotoscoping for X-ray video approaches. We examined the use of a machine learning tool, DeepLabCut, to perform automated, markerless tracking in bi-planar X-ray videos of locomoting rats. Models were trained on 590 pairs of video frames to identify 19 unique skeletal landmarks of the pelvic limb. Accuracy, precision and time savings were assessed. Machineidentified landmarks deviated from manually labeled counterparts by 2.4 +/- 0.2 mm (n=1710 landmarks). DeepLabCut decreased analysis time by over three orders of magnitude (1627x) compared with manual labeling. Distribution of these models may enable the processing of a large volume of accurate X-ray kinematics locomotion data in a fraction of the time without requiring surgically implanted markers.
更多查看译文
关键词
Biomechanics, X-ray videography, Rat kinematics, XMA
AI 理解论文
溯源树
样例
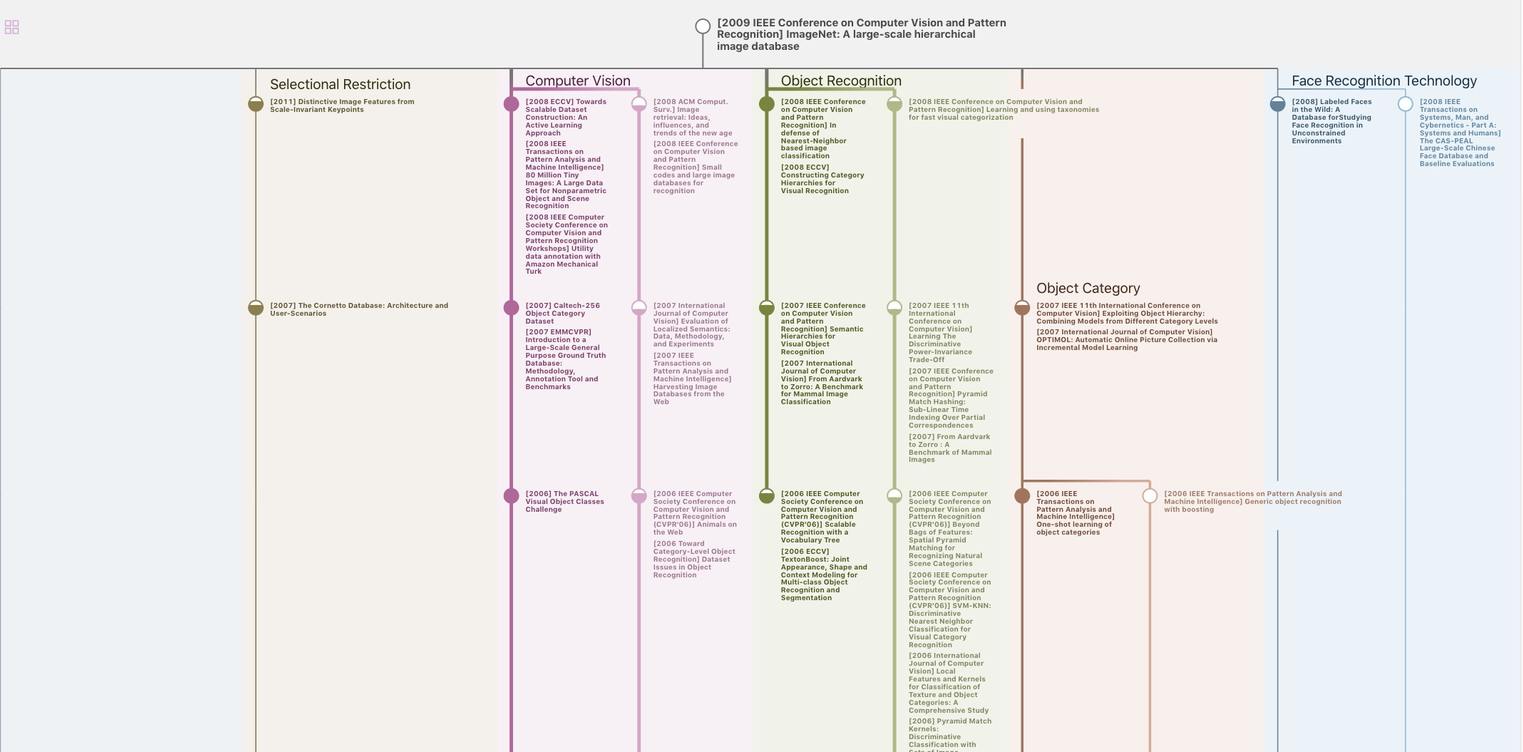
生成溯源树,研究论文发展脉络
Chat Paper
正在生成论文摘要