Identifying the tumor location-associated candidate genes in development of new drugs for colorectal cancer using machine-learning-based approach
Medical & Biological Engineering & Computing(2022)
摘要
Numerous studies have been conducted to elucidate the relation of tumor proximity to cancer prognosis and treatment efficacy in colorectal cancer. However, the molecular pathways and prognoses of left- and right-sided colorectal cancers are different, and this difference has not been fully investigated at the genomic level. In this study, a set of data science approaches, including six feature selection methods and three classification models, were used in predicting tumor location from gene expression profiles. Specificity, sensitivity, accuracy, and Mathew’s correlation coefficient (MCC) evaluation metrics were used to evaluate the classification ability. Gene ontology enrichment analysis was applied by the Gene Ontology PANTHER Classification System. For the most significant 50 genes, protein–protein interactions and drug-gene interactions were analyzed using the GeneMANIA, CytoScape, CytoHubba, MCODE, and DGIdb databases. The highest classification accuracy (90%) is achieved with the most significant 200 genes when the ensemble-decision tree classification model is used with the ReliefF feature selection method. Molecular pathways and drug interactions are investigated for the most significant 50 genes. It is concluded that a machine-learning-based approach could be useful to discover the significant genes that may have an important role in the development of new therapies and drugs for colorectal cancer. Graphical abstract
更多查看译文
关键词
Machine-learning, Classification, Druggable gene, Tumor location, Colorectal cancer, Gene expression
AI 理解论文
溯源树
样例
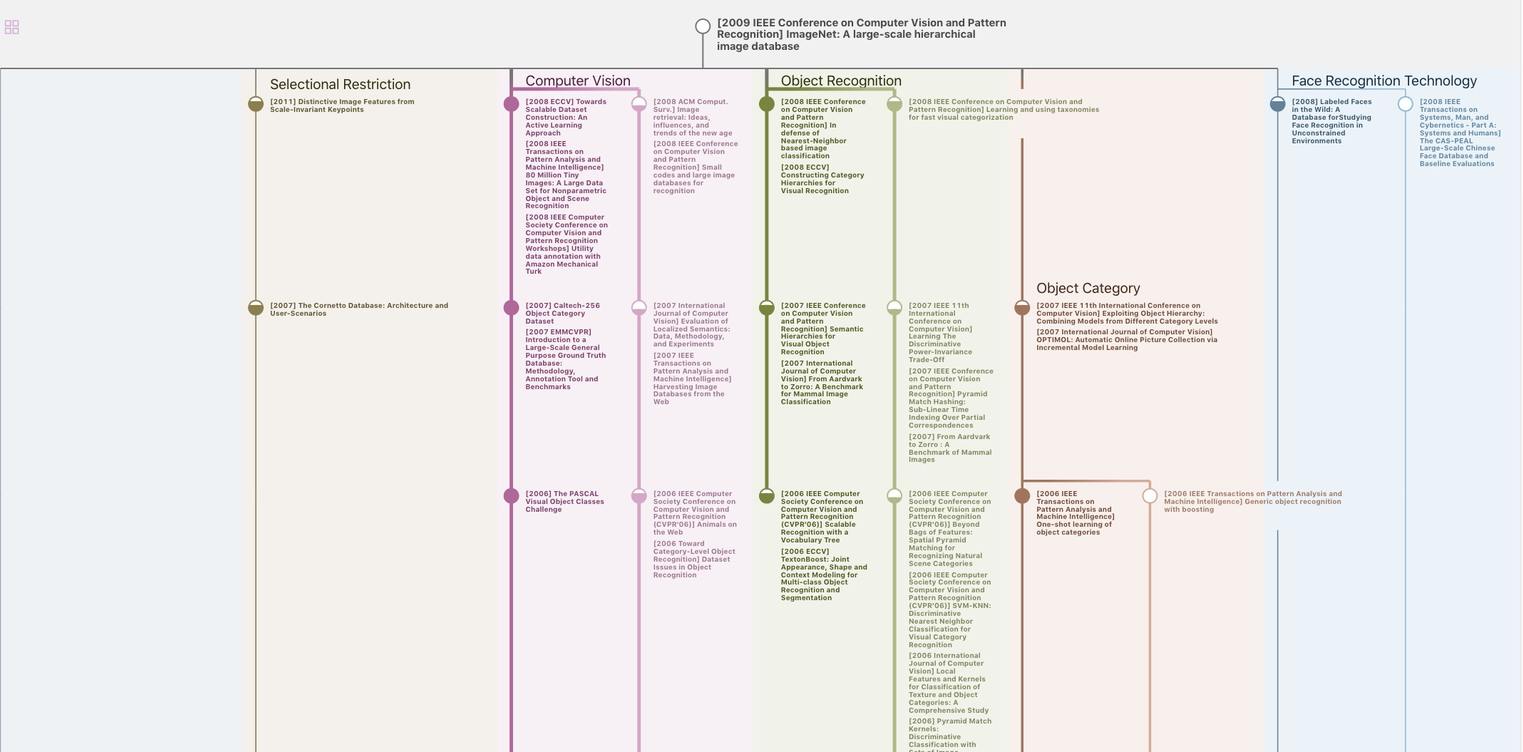
生成溯源树,研究论文发展脉络
Chat Paper
正在生成论文摘要