Quantitative signal quality assessment for large-scale continuous scalp electroencephalography from a big data perspective.
Physiological measurement(2023)
摘要
. Despite electroencephalography (EEG) being a widely used neuroimaging technique with an excellent temporal resolution, in practice, the signals are heavily contaminated by artifacts masking responses of interest in an experiment. It is thus essential to guarantee a prompt and effective detection of artifacts that provides quantitative quality assessment (QA) on raw EEG data. This type of pipeline is crucial for large-scale EEG studies. However, current EEG QA studies are still limited.. In this study, combined from a big data perspective, we therefore describe a quantitative signal quality assessment pipeline, a stable and general threshold-based QA pipeline that automatically integrates artifact detection and new QA measures to assess continuous resting-state raw EEG data. One simulation dataset and two resting-state EEG datasets from 42 healthy subjects and 983 clinical patients were utilized to calibrate the QA pipeline.. The results demonstrate that (1) the QA indices selected are sensitive: they almost strictly and linearly decrease as the noise level increases; (2) stable, replicable QA thresholds are valid for other experimental and clinical EEG datasets; and (3) use of the QA pipeline on these datasets reveals that high-frequency noises are the most common noises in EEG practice. The QA pipeline is also deployed in the WeBrain cloud platform (https://webrain.uestc.edu.cn/, the Chinese EEG Brain Consortium portal).. These findings suggest that the proposed QA pipeline may be a stable and promising approach for quantitative EEG signal quality assessment in large-scale EEG studies.
更多查看译文
关键词
artifact detection,big data,quality assessment,quantitative analysis,raw scalp EEG
AI 理解论文
溯源树
样例
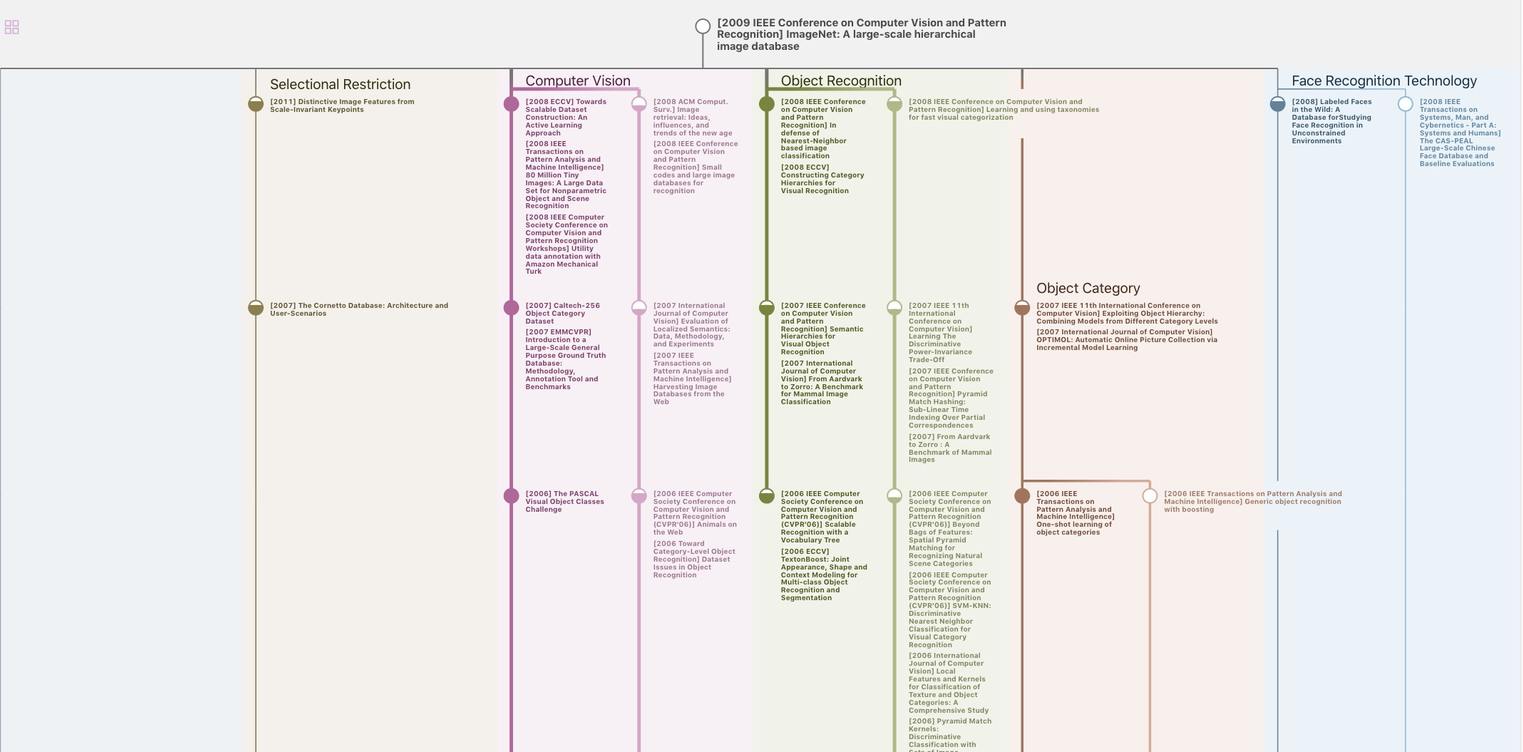
生成溯源树,研究论文发展脉络
Chat Paper
正在生成论文摘要