A Load Forecasting Algorithm for Power Internet of Things Using Training Data Dimension Expansion and Ensemble Learning Technique
JOURNAL OF SENSORS(2022)
摘要
With the development of the power internet of things (IOT), load forecasting will play an important role the power system. It can optimize the power generation planning and improve the economical operation of power IOT. In this paper, a new loading forecasting algorithm for power IOT is proposed using training data dimension expansion and ensemble learning. In the offline phase, the obtained meteorological data and time information is normalized to remove the unit effect at first. Then, the Hampel filter is used to cope with the outliers of the meteorological data from sensors. Through the preprocessing, the fingerprint of the training data is constructed. Next, the matrix multiplication method is proposed to expand the dimension of training data fingerprint information. Finally, the ensemble learning combining multiple long short-term memory (LSTM) networks are proposed to obtain multiple power load forecasting models and corresponding weight coefficients. In the online phase, the obtained meteorological data and time information are preprocessed to form the input of and power load forecasting models. The final power load forecasting is obtained by linear weighted sum method with intermediate forecasting result. In the proposed algorithm, more features of training data can be obtained by the data dimension expansion. Moreover, the ensemble learning using LSTM can make fully use of the timing sequence of training and improve the generalization performance of offline training. Experiment results illustrate that the proposed algorithm has better forecasting performance than existing methods.
更多查看译文
关键词
load forecasting algorithm,power internet,training data dimension expansion
AI 理解论文
溯源树
样例
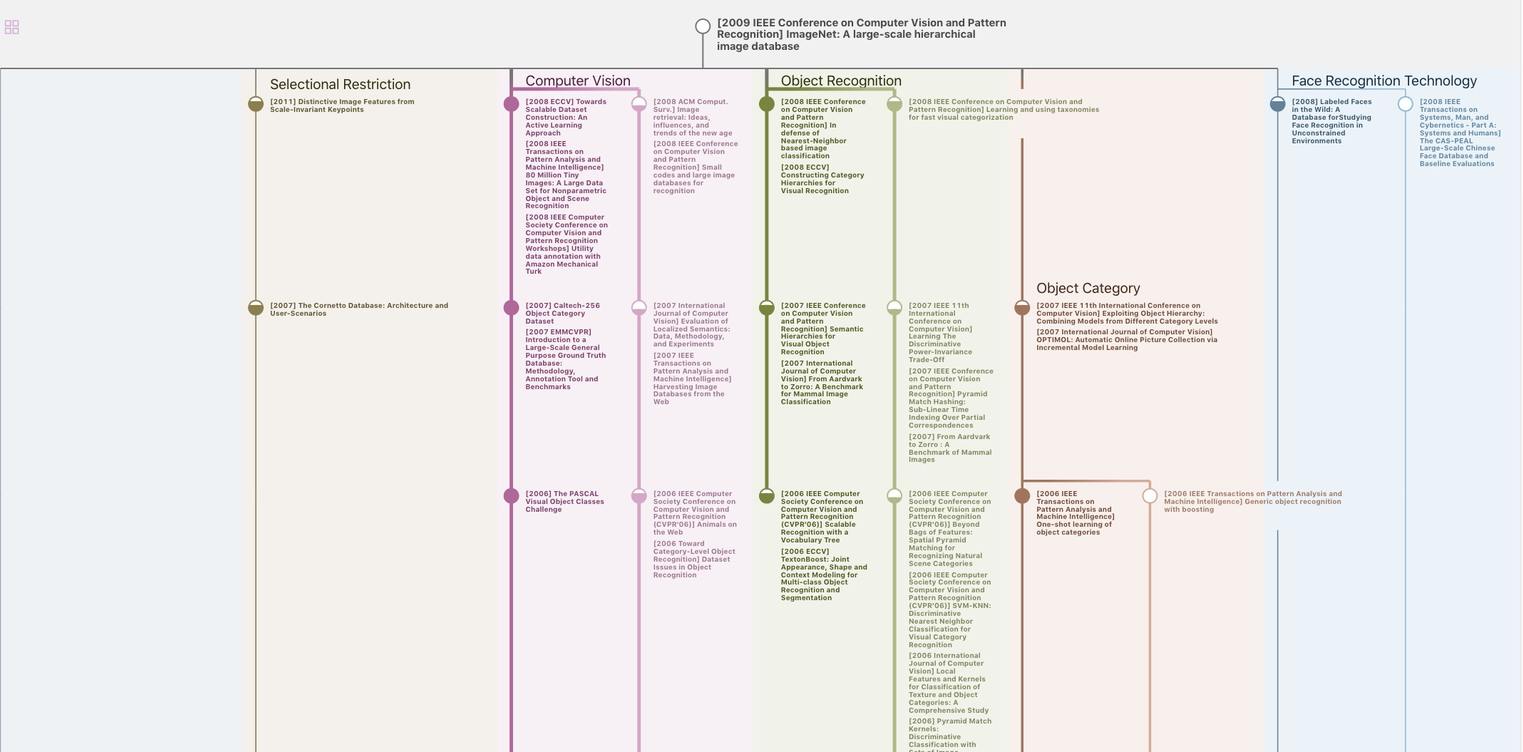
生成溯源树,研究论文发展脉络
Chat Paper
正在生成论文摘要