Soybean yield variability per plant in subtropical climate: sample size definition and prediction models for precision statistics
EUROPEAN JOURNAL OF AGRONOMY(2022)
Abstract
Empirical decision-making on the number of plants to be sampled per experimental unit when assessing soybean grain yield is a commonly observed situation. The cause for this is unknowing how that decision affects experimental precision statistics, which has not yet been reported. Therefore, the aims of this study were: to analyze the response of experimental precision statistics as a function of the variations in the number of plants collected per experimental unit in highland and lowland systems; to define the optimal sample size per experimental unit for each precision statistic; and, to propose predictive models for estimating the precision of experiments with soybean. Field experiments were carried out in two locations of Rio Grande do Sul, Brazil, using 30 cultivars in the highland trials and 20 cultivars in the lowland trials. A validation trial was also performed using 8 cultivars. A randomized block design with three repetitions was used for all trials. The grain yield of 20 plants per experimental unit was assessed, thus totaling 9000 plants. In the validation experiment, grain yield was measured in 100 plants per experimental unit, totaling 2400 plants more. From these experiments, trials with sample scenarios of 1, 2, ..., 1000 plants per experimental unit were simulated with replacement. The confidence interval width of the precision statistics reduced as the number of plants measured per experimental unit increased, allowing a higher precision of its estimates. In general, up from values of >= 70%, precision gain, in the estimates of experimental precision statistic determined in soybean highland and lowland systems, tends to stabilize. The optimal sample number per experimental unit varied between systems and experimental precision statistics. The sampling of 18 plants per experimental unit is sufficient to estimate most precision indicators reliably. Interestingly, the mechanistic response of the confidence interval width is easily modeled and can be used for predicting experimental precision in highland and lowland cropping systems. From this analysis, a methodology for predicting experimental precision was outlined based on sample size per experimental unit, thus bringing new insight into future experimental planning for soybean experiments in highland and lowland systems in subtropical climate.
MoreTranslated text
Key words
Experimental planning, Highlands, Lowlands, Modeling
AI Read Science
Must-Reading Tree
Example
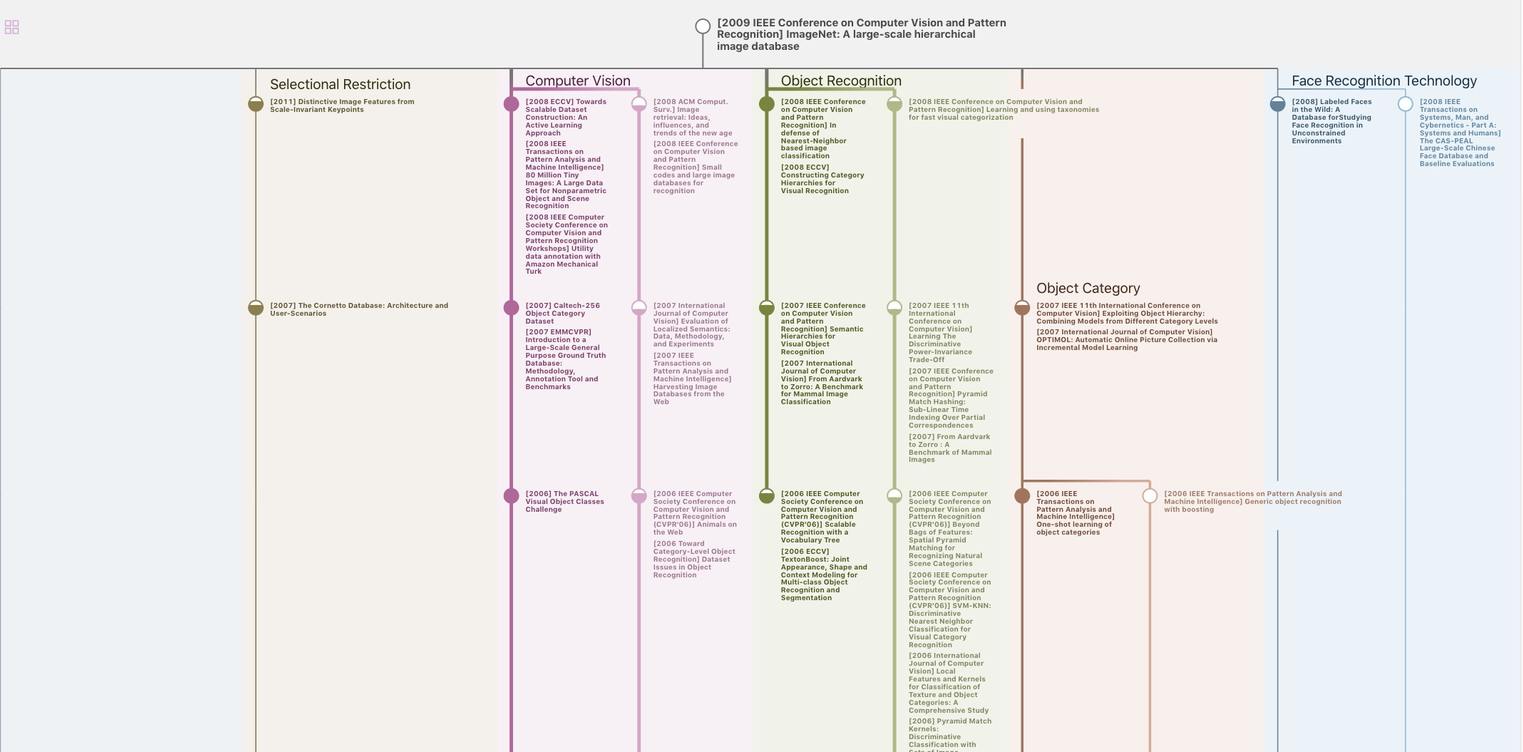
Generate MRT to find the research sequence of this paper
Chat Paper
Summary is being generated by the instructions you defined