Wind turbine blade icing detection: a federated learning approach
ENERGY(2022)
摘要
Wind farms are often located at high latitudes, which entails a high risk of icing for wind turbine blades. Traditional anti-icing methods rely primarily on manual observation, the use of special materials, or external sensors/tools, but these methods are limited by human experience, additional costs, and un-derstanding of the mechanical mechanism. Model-based approaches rely heavily on prior knowledge and are subject to misinterpretation. Data-driven approaches can deliver promising solutions but require large datasets for training, which might face significant challenges with respect to data management, e.g., privacy protection and ownership. To address these issues, this paper proposes a federated learning (FL) based model for blade icing detection. The proposed approach first creates a prototype-based model for each client and then aggregates all client models into a globally weighted model. The clients use a prototype-based modeling method to address the data imbalance problem, while using the FL-based learning method to ensure data security and safety. The proposed model is comprehensively evalu-ated using data from two wind farms, with 70 wind turbines. The results validate the effectiveness of the proposed prototype-based client model for feature extraction, and the superiority over the five baselines in terms of icing detection accuracy. In addition, the experiment demonstrates the promising result of online blade icing detection, with almost 100% accuracy.
更多查看译文
关键词
Wind turbine, Blade icing detection, Federated learning, Imbalanced learning, Time series classi fication
AI 理解论文
溯源树
样例
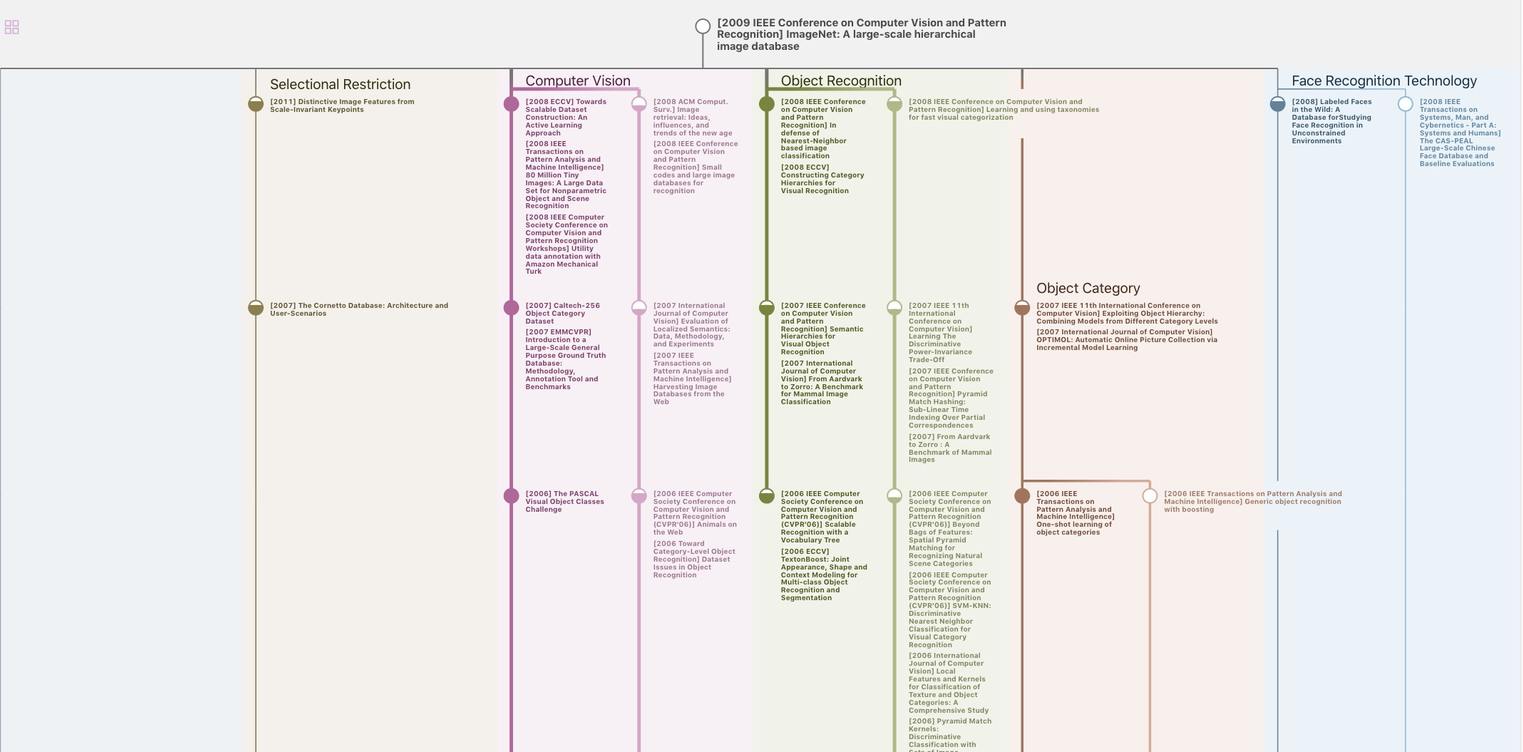
生成溯源树,研究论文发展脉络
Chat Paper
正在生成论文摘要