Optimal time-based strategy for automated negotiation
APPLIED INTELLIGENCE(2023)
摘要
Recent years are showing increased adoption of AI technology to automate business and production processes thanks to the recent successes of machine learning techniques. This leads to increased interest in automated negotiation as a method for achieving win-win agreements among self-interested agents. Research in automated negotiation can be traced back to the Nash bargaining game in the mid 20th century. Nevertheless, finding an optimal negotiation strategy against an unknown opponent with an unknown utility function is still an open area of research. The most recent result in this area is the Greedy Concession Algorithm (GCA) which can be shown to be optimal under specific constraints on both the negotiation protocol (non-repeating offers), opponent (static acceptance-model) and search space (deterministic time-based strategies). In this paper, we extend this line of work by providing an algorithmically faster version of GCA called Quick GCA which reduces the time-complexity of the search process from O((KT)-T-2) to O(KT) where K is the size of the outcome-space and T is the number of negotiation rounds allowed. Moreover, we show that GCA/QGCA can be applied in a more general setting; Namely with repeating-offers protocols and to search the more general probabilistic time-based strategies. Finally, we heuristically extend QGCA to more general opponents with general time-dependent acceptance-model and negotiation settings (real-time limited negotiations) in three steps called QGCA(+), PBS, and PA that iteratively and greedily modify the policy proposed by QGCA(+) applied to an approximate static acceptance model. The paper evaluates the proposed approach empirically against state of the art negotiation strategies (winners of all relevant ANAC competition winners) and shows that it outperforms them in a wide variety of negotiation scenarios.
更多查看译文
关键词
Automated negotiation,Concession policy,Simulated annealing
AI 理解论文
溯源树
样例
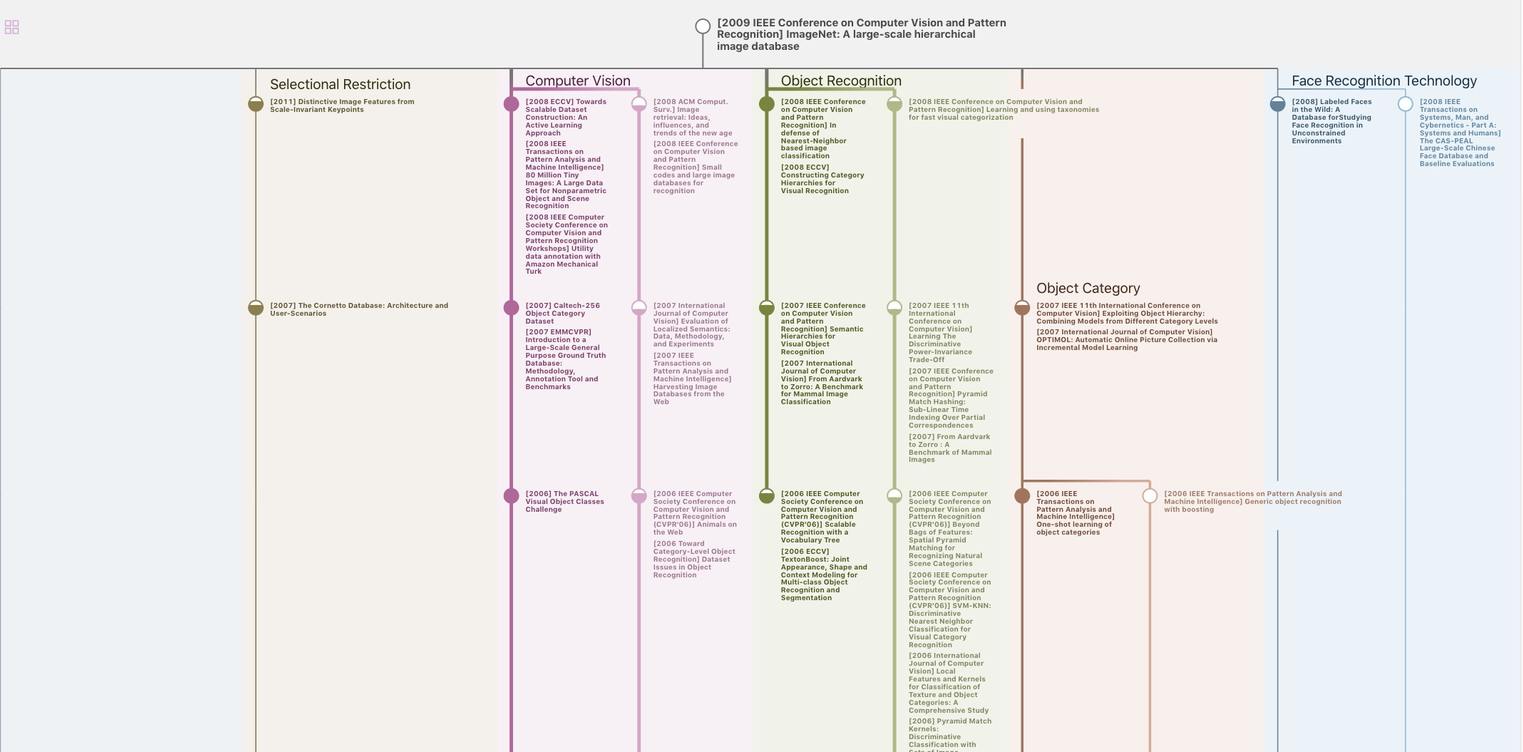
生成溯源树,研究论文发展脉络
Chat Paper
正在生成论文摘要