Identification of Lithology Using Sentinel-2A Through an Ensemble of Machine Learning Algorithms
INTERNATIONAL JOURNAL OF APPLIED GEOSPATIAL RESEARCH(2022)
摘要
Remotely sensed data has become an effective, operative, and applicable tool that provides critical support for geological surveys and studies by reducing the costs and increasing the precision. Advances in remote-sensing data analysis methods, like machine learning algorithms, allow for easy and impartial geological mapping. This study aims to carry out a rigorous comparison of the performance of three supervised classification methods, random forest, k-nearest neighbor, and maximum likelihood, using remote sensing data and additional information in Souk El Had N'Befourna region. The enhancement of remote sensing geological classification by using geomorphometric features, principal component analysis, gray level co-occurrence matrix (GLCM), and multispectral data of the Sentinel-2A imagery was highlighted. The random forest algorithm showed reliable results and discriminated limestone, dolomite, conglomerate, sandstone and rhyolite, silt and alluvium, ignimbrite, granodiorite, lutite, granite, and quartzite. The best overall accuracy (similar to 91%) was achieved by random forest algorithm.
更多查看译文
关键词
Geomorphometric Features, GLCM, Principal Component Analysis, Remote Sensing, Supervised Classification
AI 理解论文
溯源树
样例
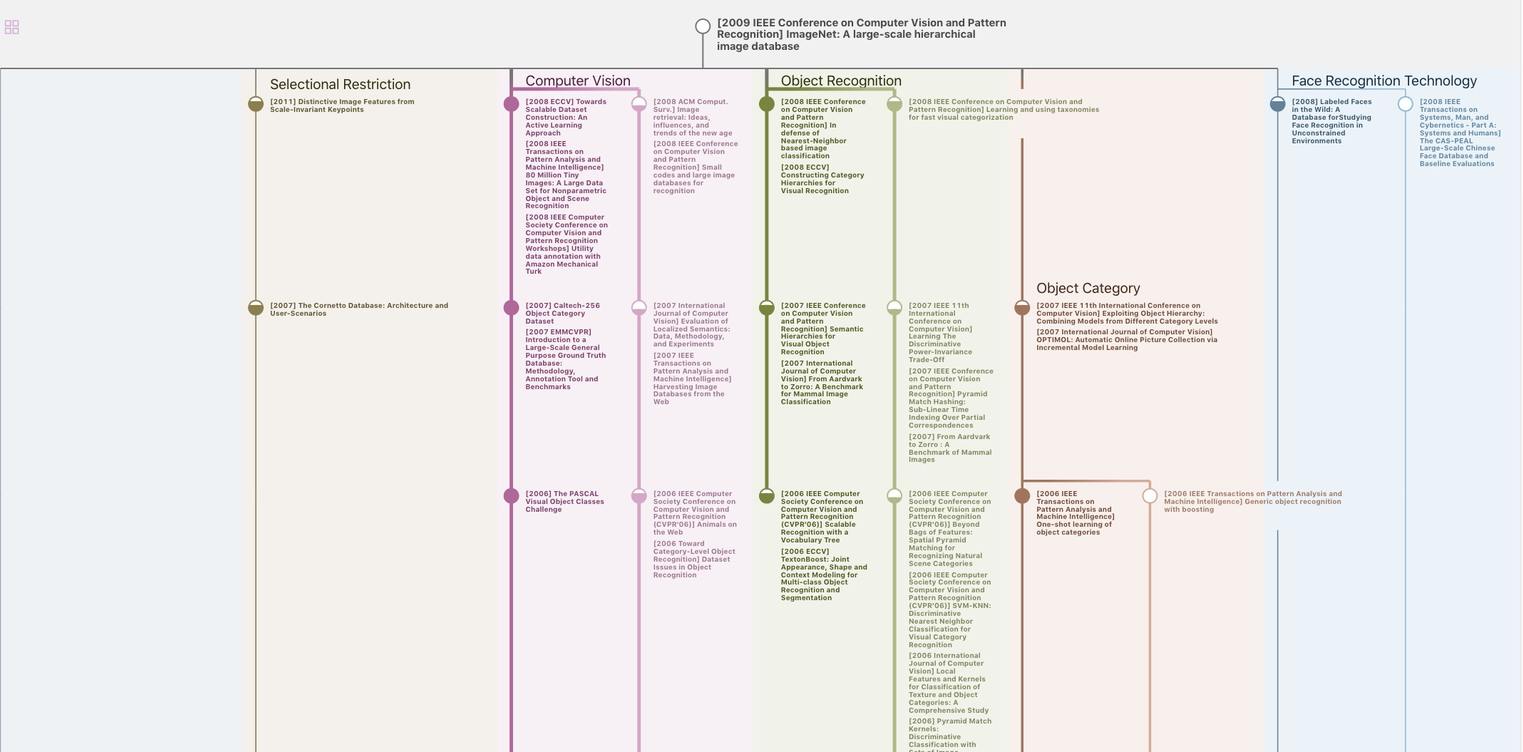
生成溯源树,研究论文发展脉络
Chat Paper
正在生成论文摘要